DeePKS Model for Halide Perovskites with the Accuracy of a Hybrid Functional
JOURNAL OF PHYSICAL CHEMISTRY C(2023)
摘要
Accurate prediction of the electronic structure properties of halide perovskites plays a significant role in the design of highly efficient and stable solar cells. While density functional theory (DFT) within the generalized gradient approximation (GGA) offers reliable prediction in terms of lattice constants and potential energy surface for halide perovskites, it severely underestimates the band gap due to the lack of a non-local exact exchange term, which exists in computationally expensive hybrid functionals. In this work, a universal Deep Kohn-Sham (DeePKS) model based on a neural network is trained so as to enable electronic structure calculations with the accuracy of the hybrid functional HSE06 and efficiency comparable to the GGA functional for a plethora of halide perovskites, i.e., ABX(3) (A = FA, MA, Cs; B = Sn, Pb; X = Cl, Br, I), as well as two types of Ruddlesden-Popper perovskites. Forces, band gaps, and density of states (DOS) predicted by our DeePKS model for all aforementioned perovskites are in good agreement with the HSE06 results, with significantly improved efficiency. In addition, even though the spin-orbit coupling (SOC) effect has not been taken into consideration during the training process, DeePKS + SOC offers a highly consistent band gap and DOS as compared to HSE06 + SOC for Pb-containing systems. We believe that such a DeePKS model can be readily applied for an accurate yet efficient prediction of various properties for the family of halide perovskites.
更多查看译文
关键词
halide perovskites
AI 理解论文
溯源树
样例
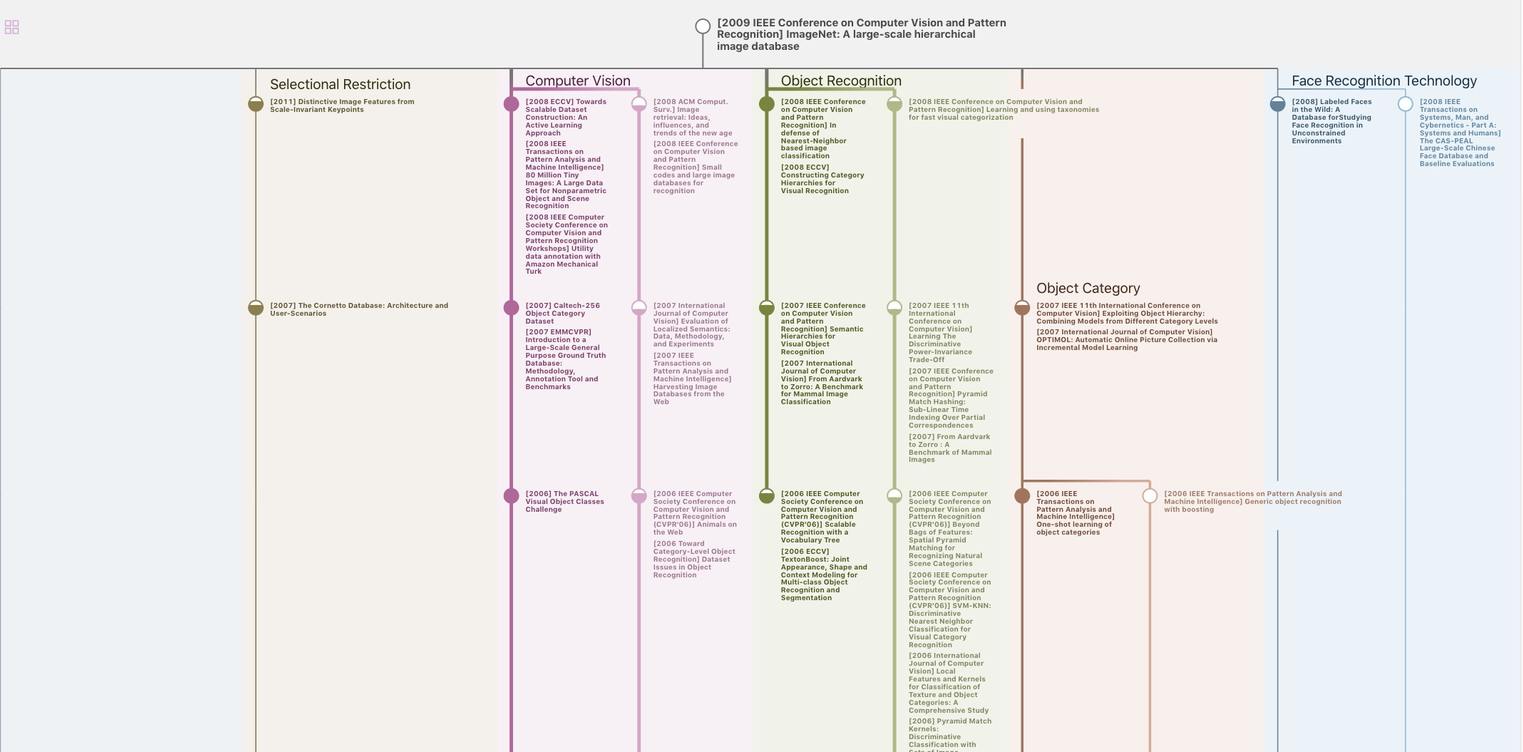
生成溯源树,研究论文发展脉络
Chat Paper
正在生成论文摘要