Robust Backdoor Attacks on Object Detection in Real World
arXiv (Cornell University)(2023)
摘要
Deep learning models are widely deployed in many applications, such as object detection in various security fields. However, these models are vulnerable to backdoor attacks. Most backdoor attacks were intensively studied on classified models, but little on object detection. Previous works mainly focused on the backdoor attack in the digital world, but neglect the real world. Especially, the backdoor attack's effect in the real world will be easily influenced by physical factors like distance and illumination. In this paper, we proposed a variable-size backdoor trigger to adapt to the different sizes of attacked objects, overcoming the disturbance caused by the distance between the viewing point and attacked object. In addition, we proposed a backdoor training named malicious adversarial training, enabling the backdoor object detector to learn the feature of the trigger with physical noise. The experiment results show this robust backdoor attack (RBA) could enhance the attack success rate in the real world.
更多查看译文
关键词
object detection,real world
AI 理解论文
溯源树
样例
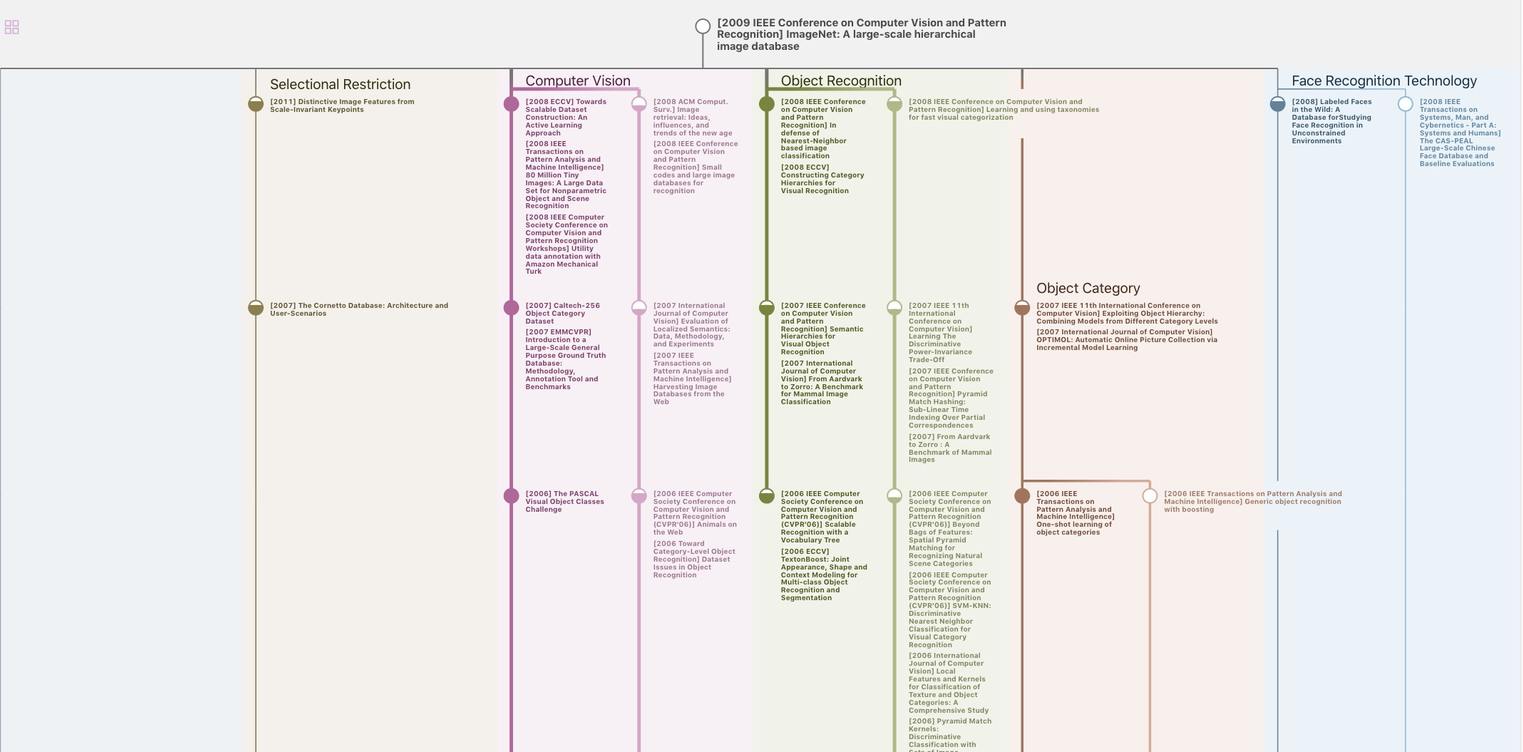
生成溯源树,研究论文发展脉络
Chat Paper
正在生成论文摘要