Covariate Measurement Error in Spatial Analysis of Count Data
Research Square (Research Square)(2023)
摘要
Measurement error arises whenever we fail to observe precisely one or more variables of interest. Covariate measurement error violates the standard assumption that covariates are fixed and accurately measured, resulting in biased parameter estimates and inflated variance estimates in a regression modeling setup. Adjustment to address this data limitation is necessary; otherwise, covariate effects may be underestimated or undetected in a regression analysis. The primary objective of this study is to develop methods for spatially correlated data, considering measurement error in covariates. To achieve our objectives, we consider the BYM2 model (an extension of the well-known Besag-York-Mollie (BYM) model), which takes into account both structured variation due to spatial adjacency of the units and unstructured variation due to random noise from the observed data. Although computationally intensive, the approach is highly efficient for spatial data analysis. We propose an extension of this methodology for error-prone covariates. We develop a Bayesian framework of the BYM2 model, with the error-prone covariates adjusted via two popular measurement error assumptions, namely, the Berkson measurement error model and the classical measurement error model. Our simulation study demonstrates that the use of a measurement error model for an error-prone covariate in the BYM2 model has the advantage of producing a superior fit. We also conclude that a BYM2 model without considering covariate measurement error may lead to highly biased estimates for certain parameters. Finally, we illustrate the application of the proposed methodology with an analysis of obesity data for 117 health regions across Canada.
更多查看译文
关键词
spatial analysis,measurement error,count
AI 理解论文
溯源树
样例
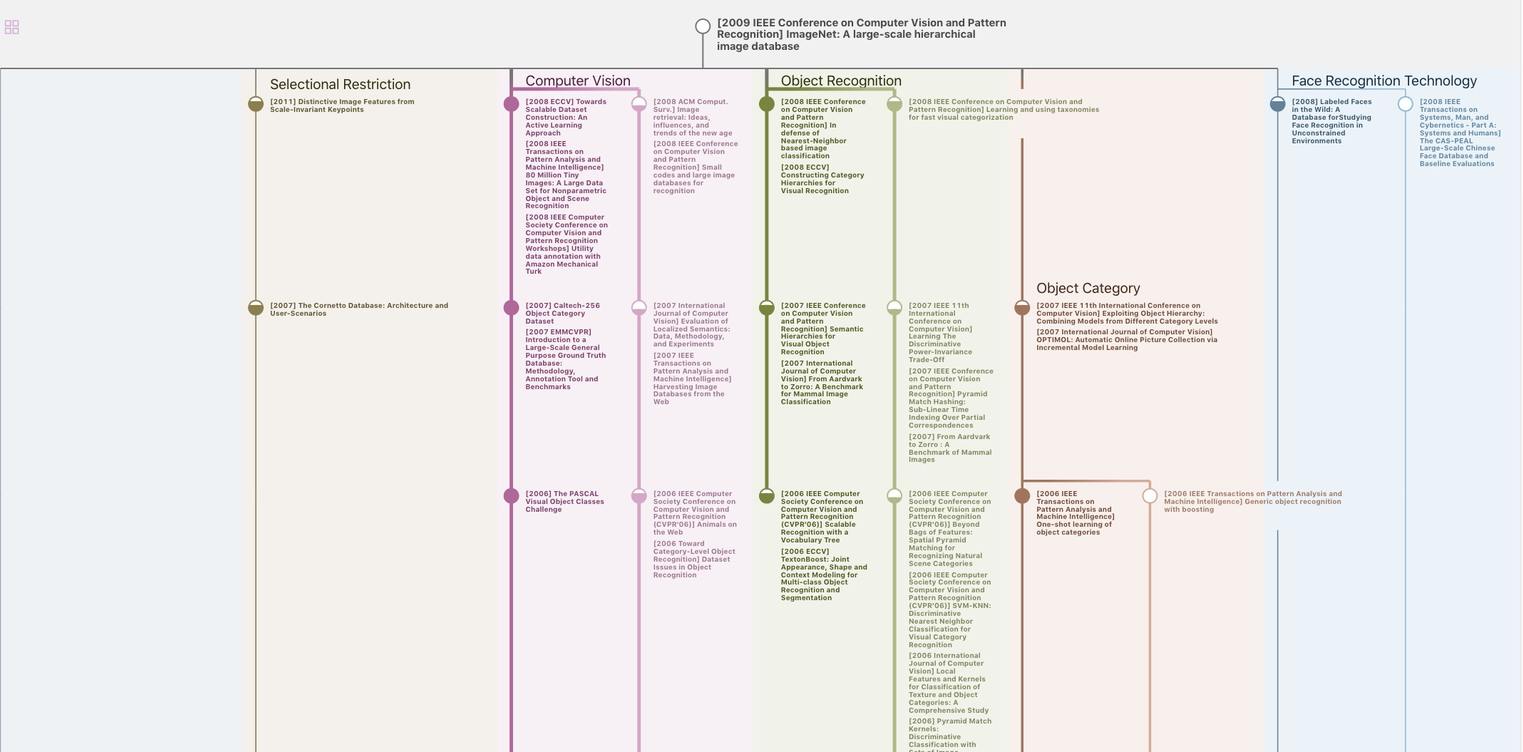
生成溯源树,研究论文发展脉络
Chat Paper
正在生成论文摘要