Optimizing Computed Tomography Image Reconstruction for Focal Hepatic Lesions: Deep Learning Image Reconstruction vs Iterative Reconstruction
Research Square (Research Square)(2023)
摘要
Background: Deep learning image reconstruction (DLIR) is a novel computed tomography (CT) reconstruction technique that minimizes image noise, enhances image quality, and enables radiation dose reduction. This study aims to compare the diagnostic performance of DLIR and iterative reconstruction (IR) in the evaluation of focal hepatic lesions. Methods: We conducted a retrospective study of 216 focal hepatic lesions in 109 adult participants who underwent abdominal CT scanning at our institution. We used DLIR (low, medium, and high strength) and IR (0%, 10%, 20%, and 30%) techniques for image reconstruction. Four experienced abdominal radiologists independently evaluated focal hepatic lesions based on five qualitative aspects (lesion detectability, lesion border, diagnostic confidence level, image artifact, and overall image quality). Quantitatively, we measured and compared the level of image noise for each technique at the liver and aorta. Results: There were significant differences ( p < .001) among the seven reconstruction techniques in terms of lesion borders, image artifacts, and overall image quality. Low-strength DLIR (DLIR-L) exhibited the best overall image quality. Although high-strength DLIR (DLIR-H) had the least image noise and fewest artifacts, it also had the lowest scores for lesion borders and overall image quality. Image noise showed a weak to moderate positive correlation with participants’ body mass index and waist circumference. Conclusions: The optimal-strength DLIR significantly improved overall image quality for evaluating focal hepatic lesions compared to the IR technique. DLIR-L achieved the best overall image quality while maintaining acceptable levels of image noise and quality of lesion borders.
更多查看译文
关键词
computed tomography image reconstruction,deep learning image reconstruction,focal hepatic lesions,iterative reconstruction
AI 理解论文
溯源树
样例
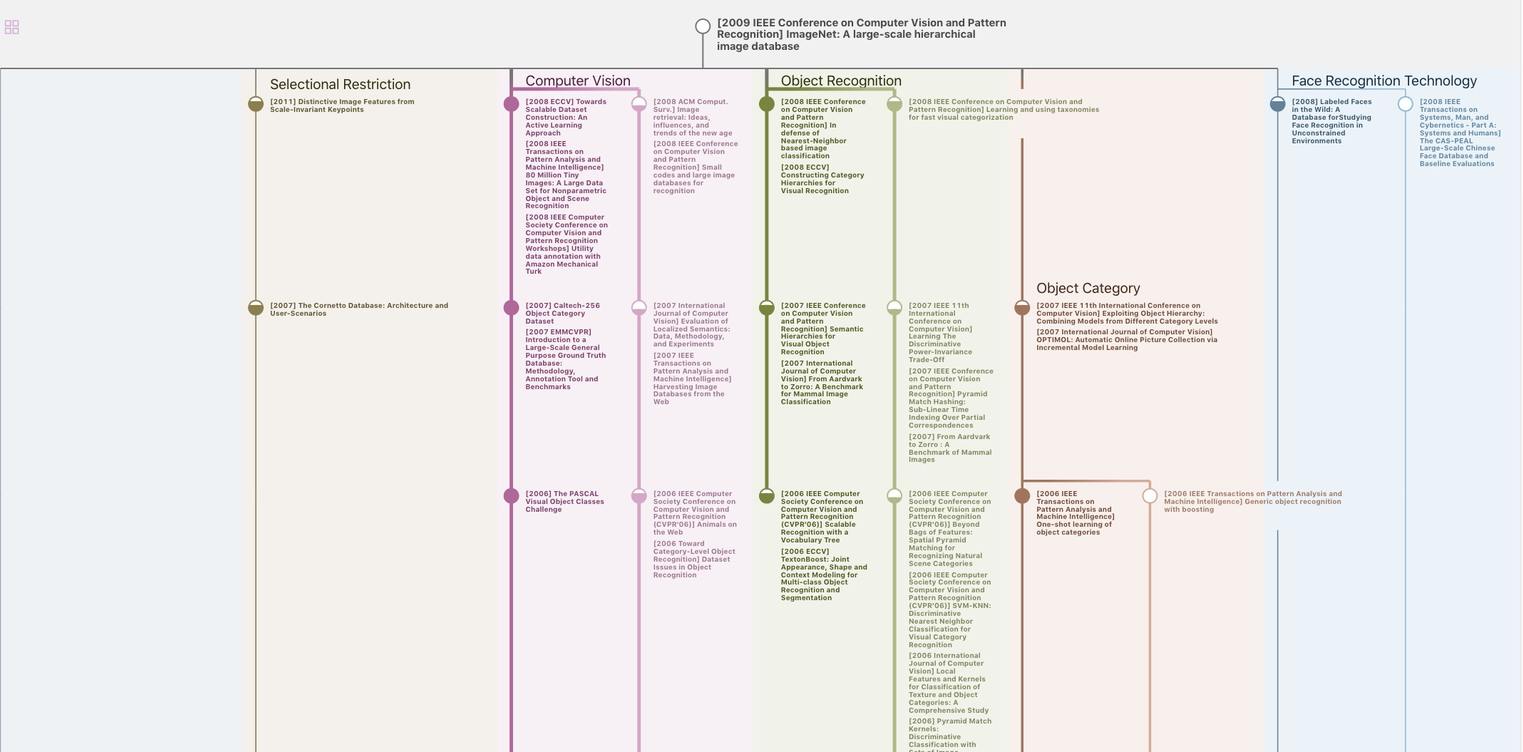
生成溯源树,研究论文发展脉络
Chat Paper
正在生成论文摘要