On the Differential Privacy in Federated Learning Based on Over-the-Air Computation
IEEE TRANSACTIONS ON WIRELESS COMMUNICATIONS(2024)
关键词
Federated learning,over-the-air computation,differential privacy,central limit theorem
AI 理解论文
溯源树
样例
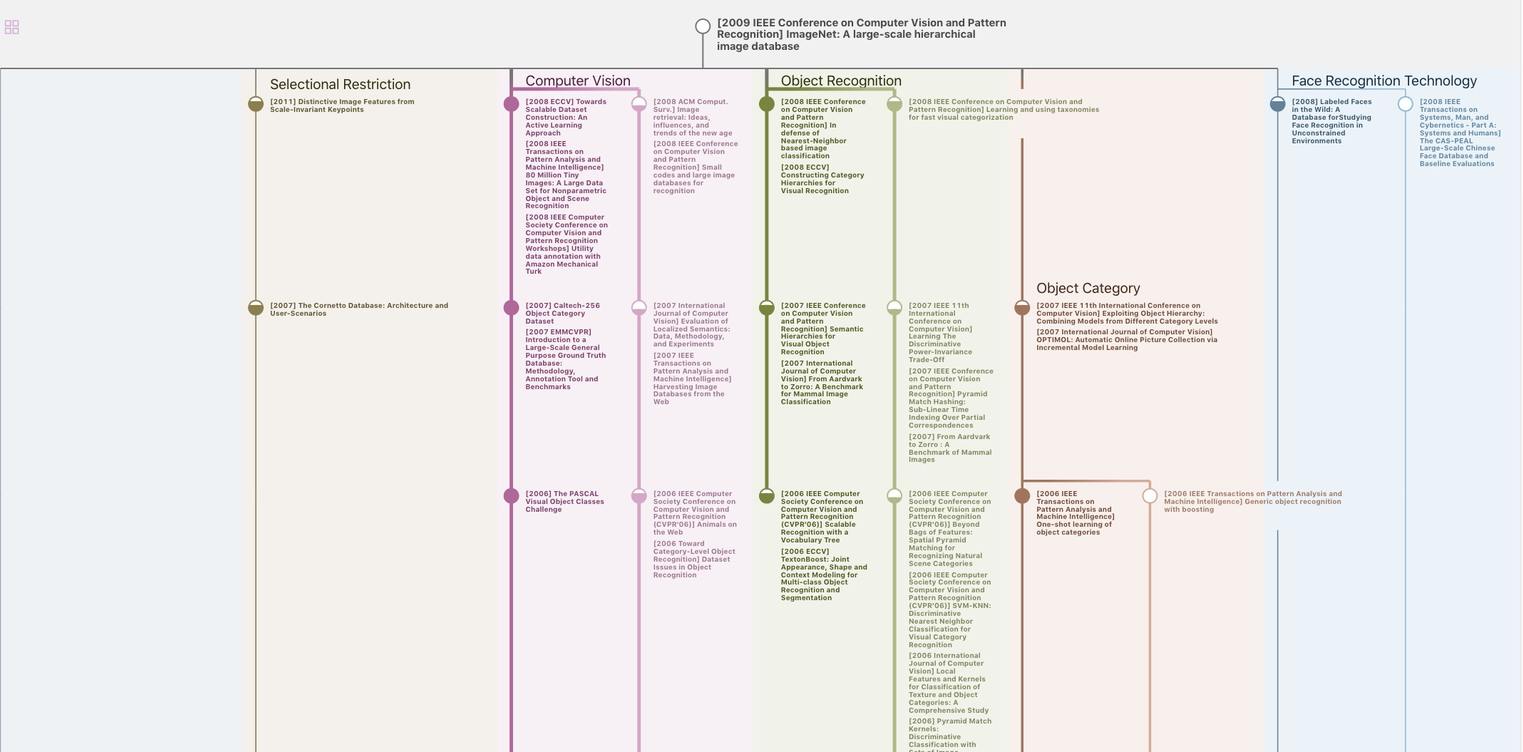
生成溯源树,研究论文发展脉络
Chat Paper
正在生成论文摘要