Surrogate-Assisted Evolutionary Multiobjective Neural Architecture Search based on Transfer Stacking and Knowledge Distillation
IEEE Transactions on Evolutionary Computation(2023)
摘要
Multiobjective neural architecture search (MONAS) methods based on evolutionary algorithms (EAs) are inefficient when the evaluation of each architecture incorporates parameter learning from scratch. A surrogate-assisted MONAS problem can be tough considering cold-start in surrogate construction, and the evaluation of predicted promising architectures could still be cumbersome. Previously solved MONAS problems are likely to convey useful knowledge that could assist solving the current MONAS problem. To take the benefit from knowledge of these previous practices, a framework tackling large-scale knowledge transfer is proposed. Through sparse-constraint transfer stacking, the surrogate for the current problem gets informative easily. With knee-region knowledge distillation from previously learned parameters of nondominated architectures, evaluation of current architectures could be efficient and credible. To avoid transferring knowledge from irrelevant problems, an iterative source selection algorithm is designed to avoid negative transfer. The proposed framework is analyzed under different source and target MONAS problem combinations. Results show that with the help of this framework, architectures with competitive performance could be found under limited evaluation budget.
更多查看译文
关键词
Surrogate-assisted evolutionary algorithm,neural architecture search,knowledge transfer,multiobjective optimization
AI 理解论文
溯源树
样例
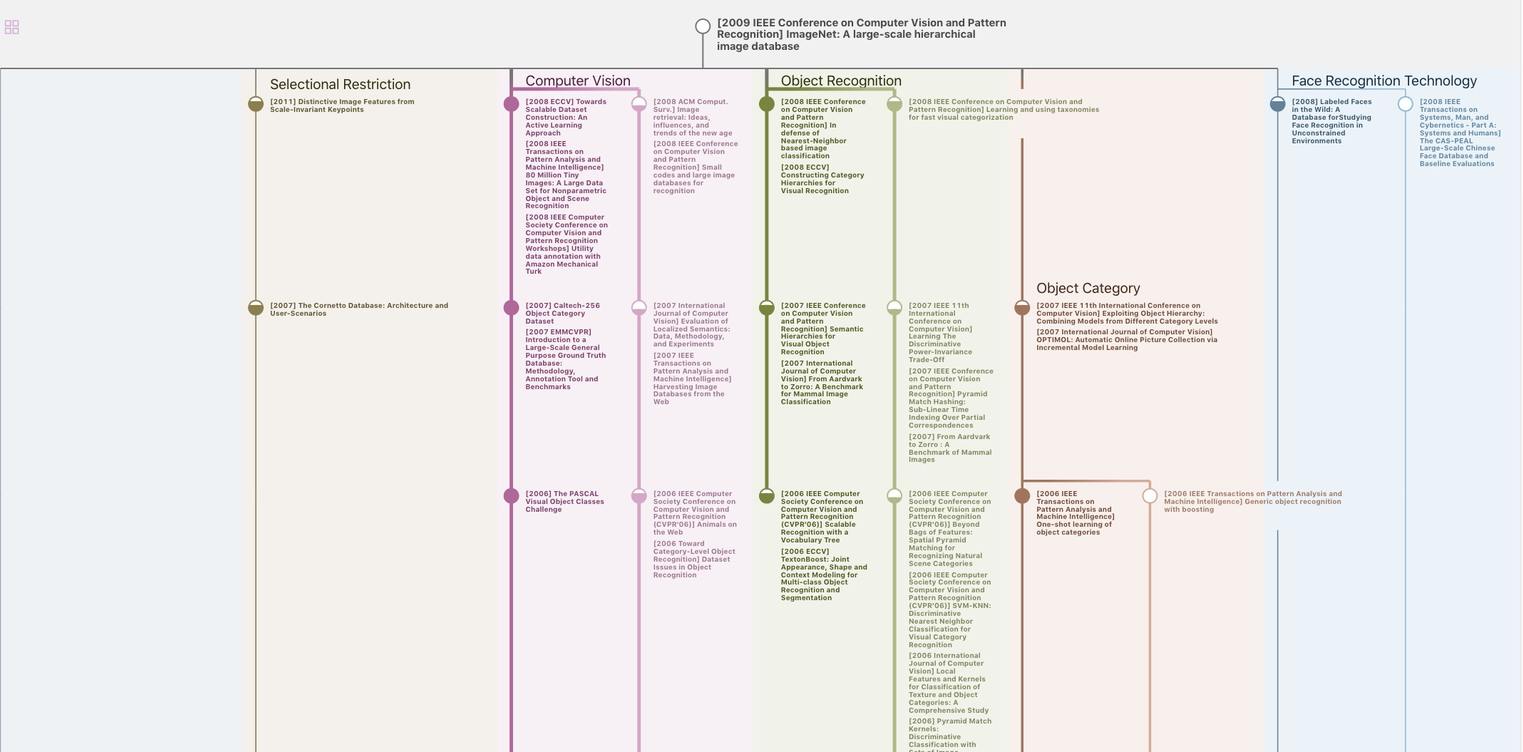
生成溯源树,研究论文发展脉络
Chat Paper
正在生成论文摘要