Dependency-Aware Dynamic Task Offloading Based on Deep Reinforcement Learning in Mobile-Edge Computing.
IEEE Trans. Netw. Serv. Manag.(2024)
摘要
The rapid advancement of mobile edge computing (MEC) networks has enabled the augmentation of the computational power of mobile devices (MDs) by offloading computationally intensive tasks to resource-rich edge nodes. This paper discusses the decision-making process for task offloading and resource allocation among multiple mobile devices connected to a base station. The primary objective is to minimize the time taken to complete tasks while simultaneously reducing energy consumption on the device under a time-varying wireless fading channel. This objective is formulated as an energy-efficiency cost (EEC) minimization problem, which cannot be solved by conventional methods. To address this challenge, we propose a dynamic offloading decision algorithm of dependent tasks (DODA-DT) that adjusts local task execution based on edge node status. The proposed algorithm facilitates fair competition among all devices for edge resources. Additionally, we use a deep reinforcement learning (DRL) algorithm based on an actor-critic learning structure to train the system to quickly identify near-optimal solutions. Numerical simulations demonstrate that the proposed algorithm effectively reduces the total cost of the task in comparison to previous algorithms.
更多查看译文
关键词
Mobile edge computing,task offloading,optimization algorithm,deep reinforcement learning
AI 理解论文
溯源树
样例
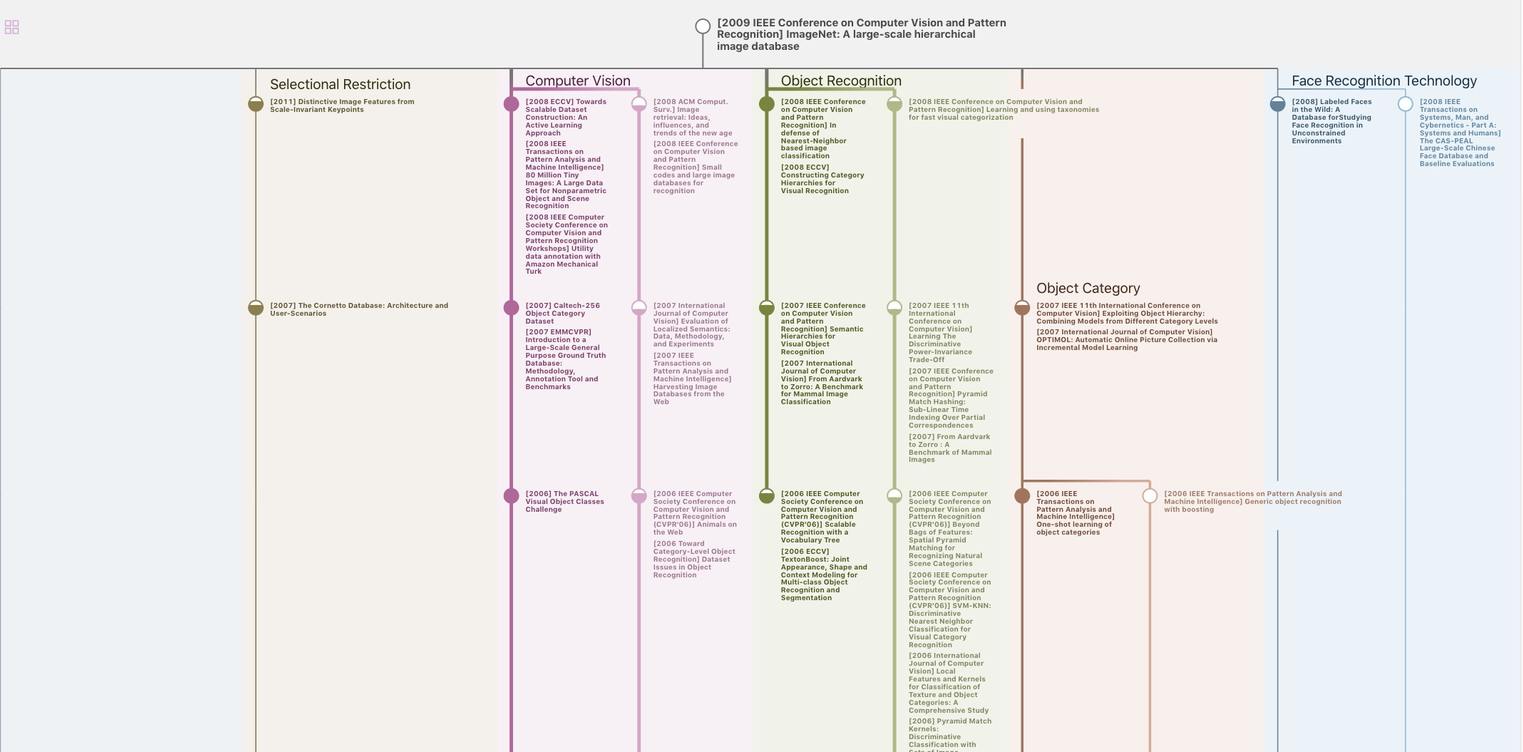
生成溯源树,研究论文发展脉络
Chat Paper
正在生成论文摘要