Smart Fiber-Optic Distributed Acoustic Sensing (sDAS) With Multitask Learning for Time-Efficient Ground Listening Applications.
IEEE Internet of Things Journal(2024)
摘要
In recent years, fiber-optical distributed acoustic sensing (DAS) has been applied to various large-scale infrastructure monitoring areas in smart cities, leading to a new generation of fiber-optic IoT for ground listening. However, its single-task-focused postprocessing methods cannot achieve real-time efficient ground event recognition and localization concurrently. In this paper, a two-level multi-task learning (MTL) enhanced smart fiber-optical distributed acoustic sensing (sDAS) system is proposed, for the first time, to simultaneously realize ground event recognition and localization. Performances and efficiency of both tasks are significantly improved by sharing knowledge across them. Besides, the imbalanced incremental learning ability for new events is also enhanced in the proposed MTL network. The total computation time for the two tasks is greatly shortened to 0.3ms for a spatial-temporal sample with 129m fiber length and 5s time frame, which equals to a processing time of 0.04s over a total fiber length of 18.7km with a spatial sampling interval of 1.29m, and is even better than the fastest single recognition reported to date. In the field test, such an MTL-enhanced sDAS system indicates excellent feature extraction performance with classification accuracy of up to 99.46% for five events and location error of 11m for two core-events at 8/16 different radial distances, which are much better than the DAS systems with multi-classifier and the combined single-task learning methods. Also, the MTL-enhanced sDAS shows strong robustness against environmental noises. Hence, it provides a breakthrough technology for time-efficient multi-task processing in smart distributed sensors.
更多查看译文
关键词
sDAS,real-time multi-task processing,synchronous event recognition and localization,fiber-optic IoT
AI 理解论文
溯源树
样例
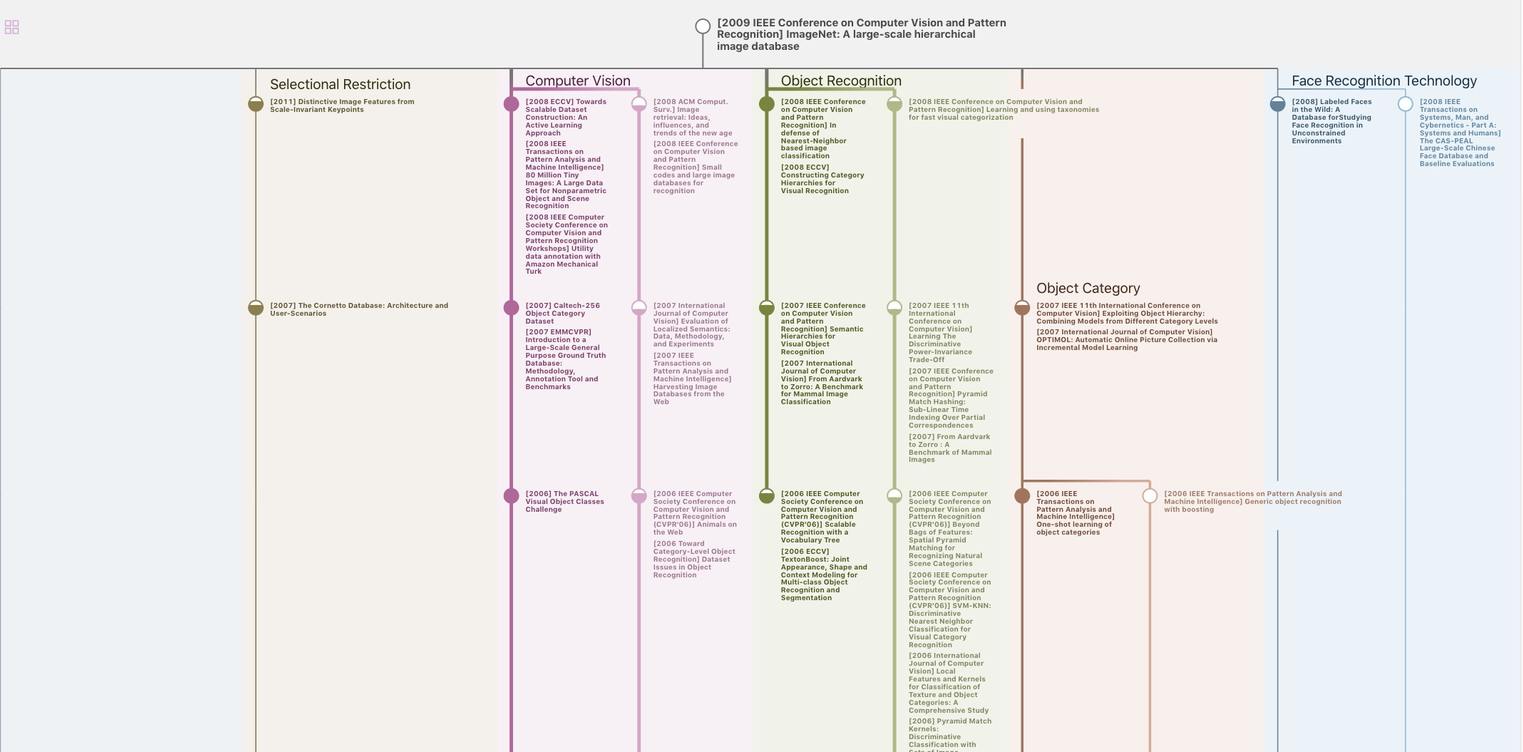
生成溯源树,研究论文发展脉络
Chat Paper
正在生成论文摘要