Uncertainty Quantification of PDE-based models using Deep Gaussian Processes and Variational Bayesian Inference
Research Square (Research Square)(2023)
摘要
Abstract Uncertainty quantification (UQ) and propagation (UP) in complex multi-scale physical models are challenging problems due to the high-dimensionality of the inputs and the outputs for such models and the corresponding computational complexity. These challenges could be tackled using Gaussian process (GP) models by constructing a surrogate response surface. This response surface is an efficient approximation of the underlying expensive model which can be then used for UQ and UP. However, the standard GP model is hampered by various issues such as the curse of dimensionality, difficulties in capturing the non-Gaussian complex nature of the input/output relation, and modelling discontinuities of 1 model outputs. To overcome these issues, this work aims to use a non-linear GP model, known as deep Gaussian process for the UQ and UP of multi-scale models. Deep GP is an efficient approach for deep learning and modelling of high-dimensional complex systems and consists of several hidden layers connected with non-linear mappings. In this work, a modified variational approximation framework is presented to analytically approximate the posterior distribution of the model outputs using the model inputs. The proposed framework automatically selects the dimensionality of each hidden layer to prevent over-fitting. Consequently, the deep GP model is more flexible and can be used to propagate uncertainty obtained in each layer across the hierarchy. The suitability of this model is shown in UQ tasks by computing the error bars for the statistics of interest in a standard stochastic PDE based model.
更多查看译文
关键词
deep gaussian processes,uncertainty,models,pde-based
AI 理解论文
溯源树
样例
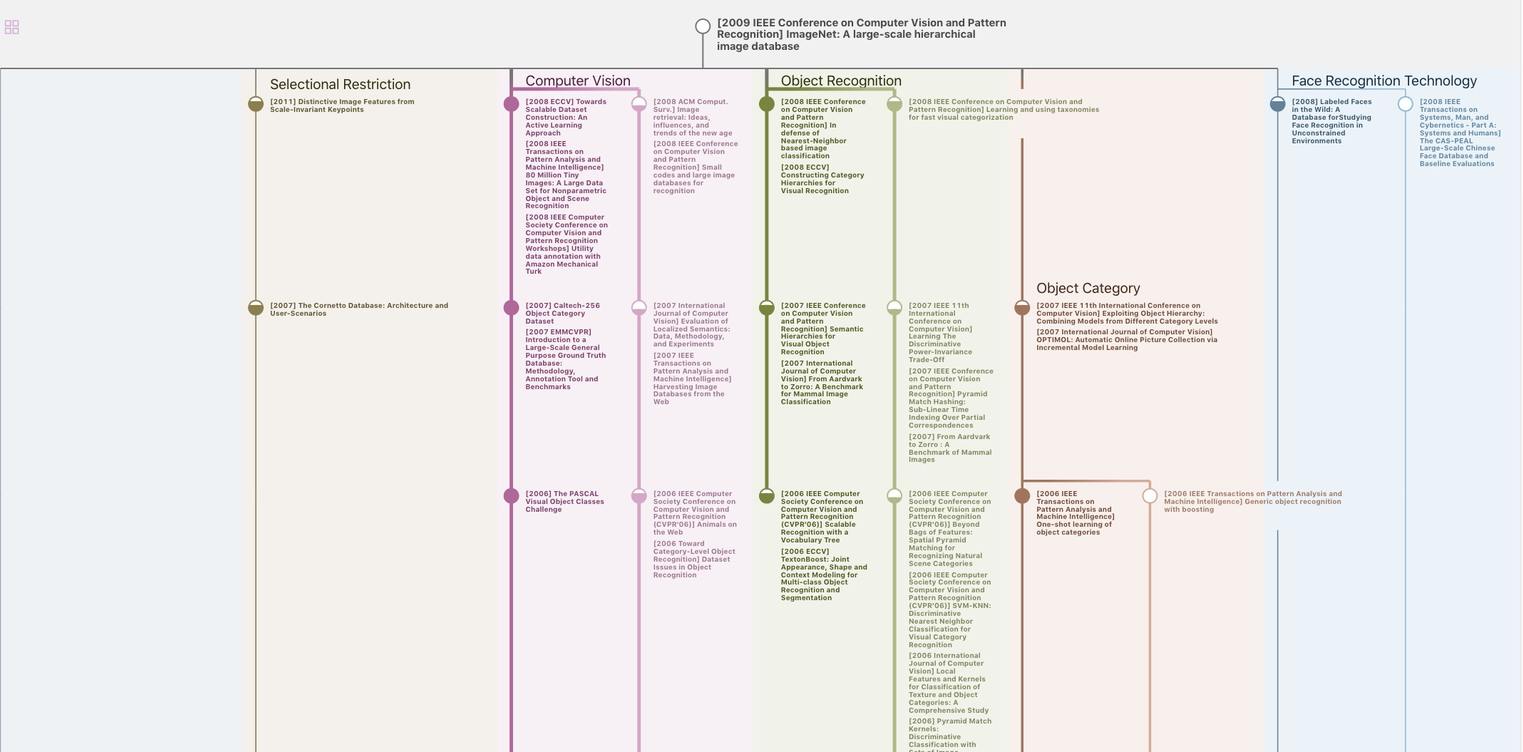
生成溯源树,研究论文发展脉络
Chat Paper
正在生成论文摘要