Deep Pyramid Network for Low-Light Endoscopic Image Enhancement.
IEEE Trans. Circuits Syst. Video Technol.(2024)
摘要
Endoscopic images captured under low-light enclosed intestinal environment usually have poor visibility (manifested as uneven illumination and noise), affecting the work efficiency of physicians and the accuracy of lesion detection. To improve the image quality, the literature has reported many low-light image enhancement (LIE) methods. However, most methods do not perform well in handling the low-light endoscopic image enhancement (LEIE) task, usually bringing additional artifacts or amplifying noise. In this paper, we propose a novel deep pyramid enhancement network (DPENet) to enhance endoscopic images from both global and local perspectives. Specifically, considering the uneven illumination of endoscopic images, DPENet utilizes an image pyramid framework with three parallel branches to explore and integrate both global and local features at different scales. To suppress noise, DPENet sets multiple scale-space feature extraction blocks (SFEBs) in each branch. SFEB consists of a contextual feature extraction module (CFEM) and a spatial residual attention module (SRAM). CFEM mines contextual information to help the network understand semantic information while suppress the isolated noise. SRAM leverages the spatial attention mechanism to help the network adaptively focus on dim regions. Experimental results on a public dataset and our collected dataset show that DPENet is competent for the LEIE task with promising results, and outperforms 9 state-of-the-art LIE methods in both qualitative and quantitative aspects.
更多查看译文
关键词
Image enhancement,endoscopic image,deep pyramid network,image quality
AI 理解论文
溯源树
样例
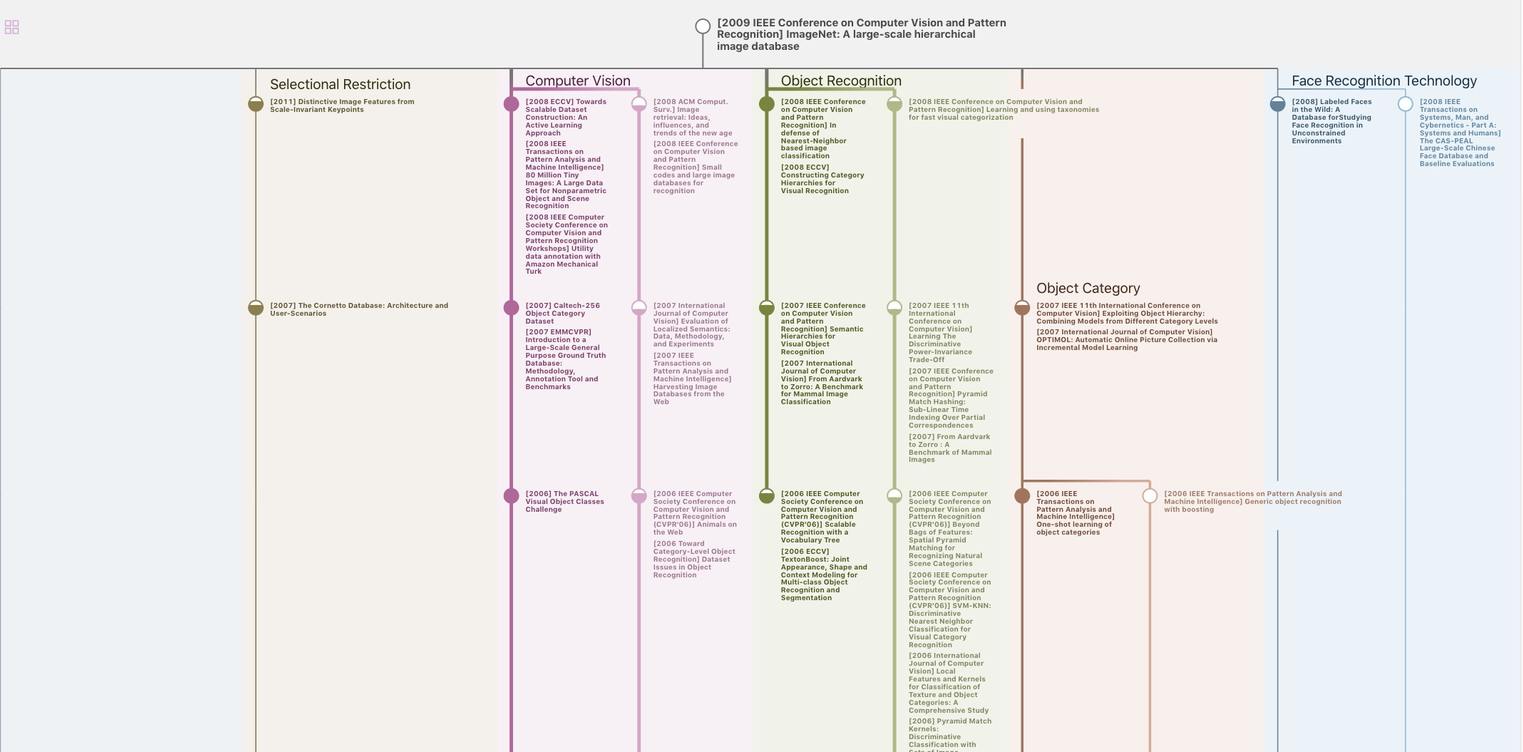
生成溯源树,研究论文发展脉络
Chat Paper
正在生成论文摘要