CHEESE: Distributed Clustering-Based Hybrid Federated Split Learning Over Edge Networks
IEEE Transactions on Parallel and Distributed Systems(2023)
摘要
Federated learning (FL) and split learning (SL) have emerged as two promising distributed machine learning paradigms. However, implementing either FL or SL over clients with limited computation and communication resources often faces the challenge of achieving delay-efficient model training. To overcome this challenge, we propose a novel distributed C lustering-based H ybrid f E d E rated S plit l E arning ( CHEESE ) framework, consolidating distributed computation resources among clients by device-to-device (D2D) communications, which works in an intra-serial inter-parallel manner. In CHEESE , each learning client (LC) can form a learning cluster with its neighboring helping clients via D2D communications to train an FL model collaboratively. Specifically, inside each cluster, the model is split into multiple model segments via a model splitting and allocation (MSA) strategy, while each cluster member trains one segment. After completing intra-cluster training, a transmission client (TC) is determined from each cluster to upload a complete model to the base station for global model aggregation under allocated bandwidth. Based on this, an overall training delay cost minimization problem is formulated, which involves the following subproblems: client clustering, MSA, TC selection, and bandwidth allocation. Due to its NP-Hardness, the problem is decoupled and solved iteratively. The client clustering problem is first transformed into a distributed clustering game based on potential game theory, where each cluster further investigates the remaining three subproblems to evaluate the utility of each clustering strategy. Specifically, a heuristic algorithm is proposed to solve the MSA problem under a given clustering strategy, and a greedy-based convex optimization approach is introduced to solve the joint TC selection and bandwidth allocation problem. Finally, we propose an overall algorithm to tackle the joint problem iteratively, to reach a Nash equilibrium. Extensive experiments on practical models and datasets demonstrate that CHEESE can significantly reduce training delay costs, as compared with conventional FL and vanilla SL.
更多查看译文
关键词
hybrid federated split learning,networks,edge,clustering-based
AI 理解论文
溯源树
样例
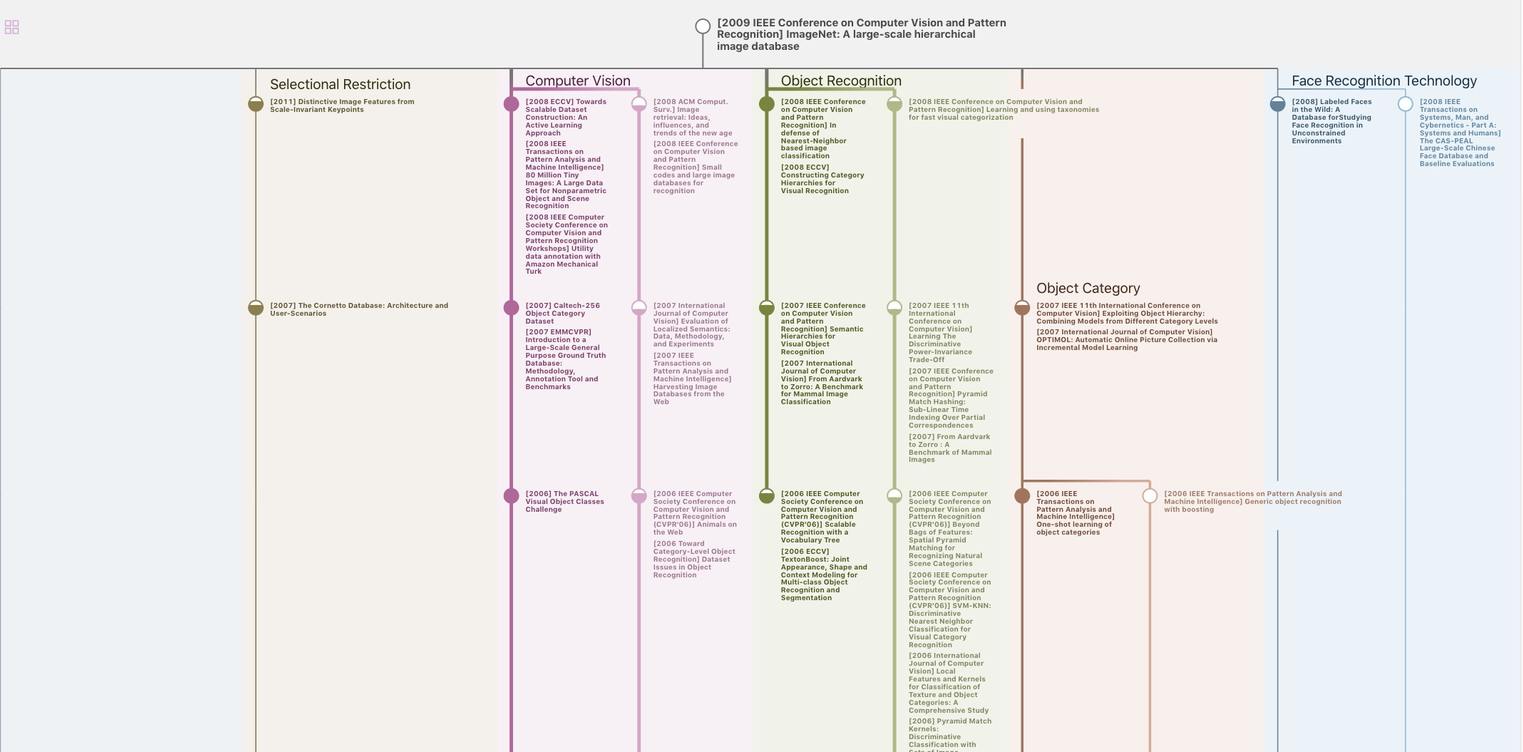
生成溯源树,研究论文发展脉络
Chat Paper
正在生成论文摘要