Photovoltaic Panel Fault Detection and Diagnosis Based on a Targeted Transformer-Style Model
IEEE Transactions on Industry Applications(2024)
摘要
The number of photovoltaic power plants is increasing rapidly and consequently their stability, efficiency and safety have become more important. In view, it is necessary to regularly detect, diagnose and maintain photovoltaic modules in a timely manner. In this work, a new image classification network based on the MPViT network structure is designed to solve the problem of fault detection and diagnosis of photovoltaic panels using image processing methods. Owing to the similarity between different types of photovoltaic panels an Enhanced Local Self-Attention block (ELSA block) is introduced to improve the learning ability of the model's local features so as to improve the model's ability to differentiate categories. Binary classification and multi-classification experiments are carried out on two datasets namely the photovoltaic module dataset based on infrared imaging (dataset1), and the photovoltaic module dataset based on electroluminescence imaging (dataset2). The M-E model (MPViT model introduced with ELSA block) achieves 94.1
$\%$
and 88.5
$\%$
, 90.7
$\%$
and 86.4
$\%$
accuracy for binary classification and multi-class classification on dataset1 and dataset2 better than MPViT respectively.
更多查看译文
关键词
Electroluminescence imaging,fault diagnosis,fault detection,infrared imaging,M-E model,photovoltaic modules
AI 理解论文
溯源树
样例
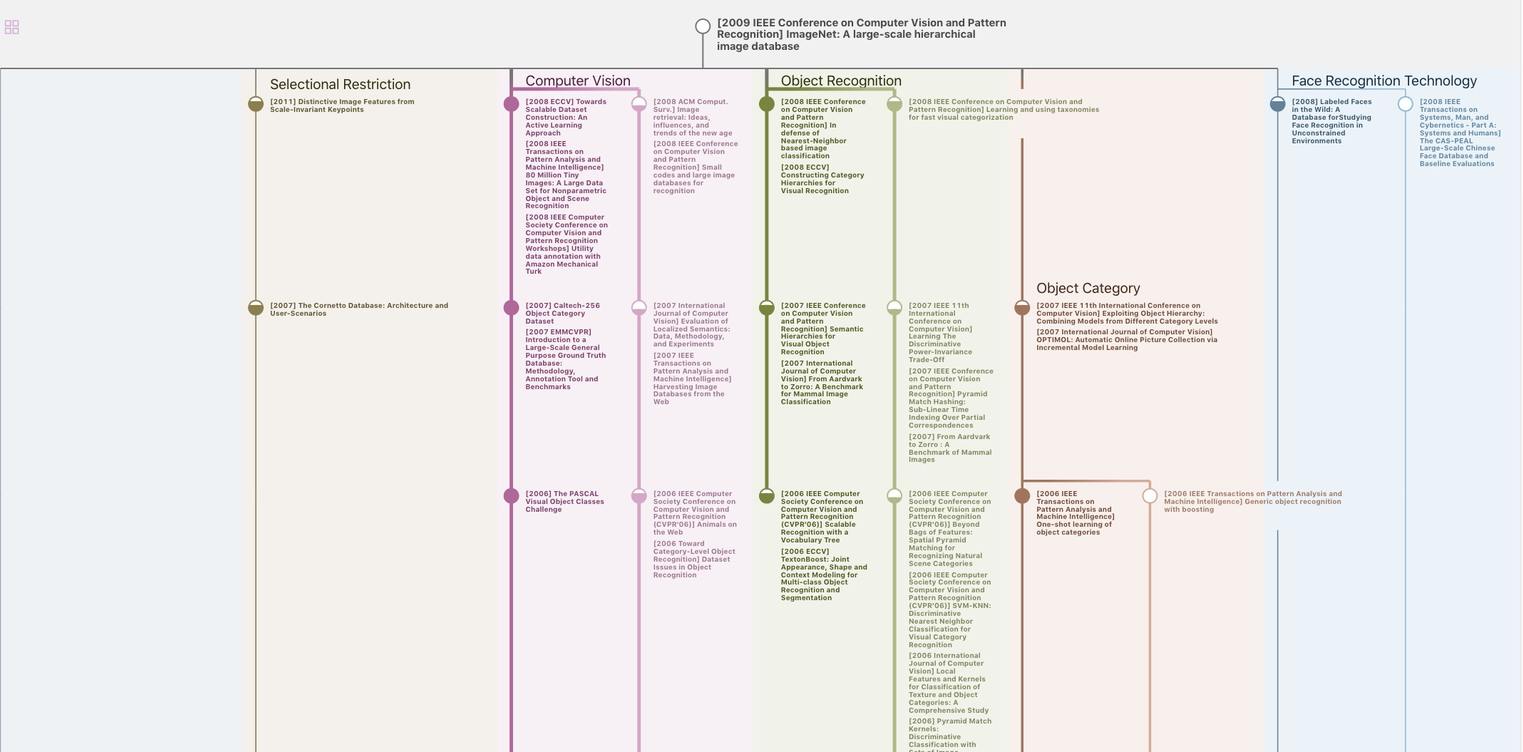
生成溯源树,研究论文发展脉络
Chat Paper
正在生成论文摘要