Comparison of regression techniques for generating surrogate models to predict the thermodynamic behavior of biomass gasification systems
Computer-aided chemical engineering(2023)
摘要
Biomass resources can play an important role in the energy transition, being a dynamic feedstock that can be transformed either into solid, liquid or gaseous fuels. Biomass gasification is a versatile way to convert waste into energy. In this work, the modeling and simulation of two different gasification processes using wood and black liquor as feedstock are performed using Aspen Plus®. The surrogate models for these biomass-based gasification systems are generated considering different techniques (e.g. artificial neural networks, random forest, and Gaussian process regression) using an Active Learning Artificial Intelligence approach. These techniques are compared in terms of their capabilities for predicting the thermodynamic behavior of the gasification systems for the different biomass resources. As a result, the surrogate models developed were able to estimate the process design and operating conditions, and the Gaussian process regression outperformed the artificial neural networks and random forest techniques. The generated models could be helpful to be further used for replacing the simulation systems in other applications, such as multi-objective optimization, at expense of lower computational requirements.
更多查看译文
关键词
surrogate models,regression techniques,thermodynamic behavior,predict
AI 理解论文
溯源树
样例
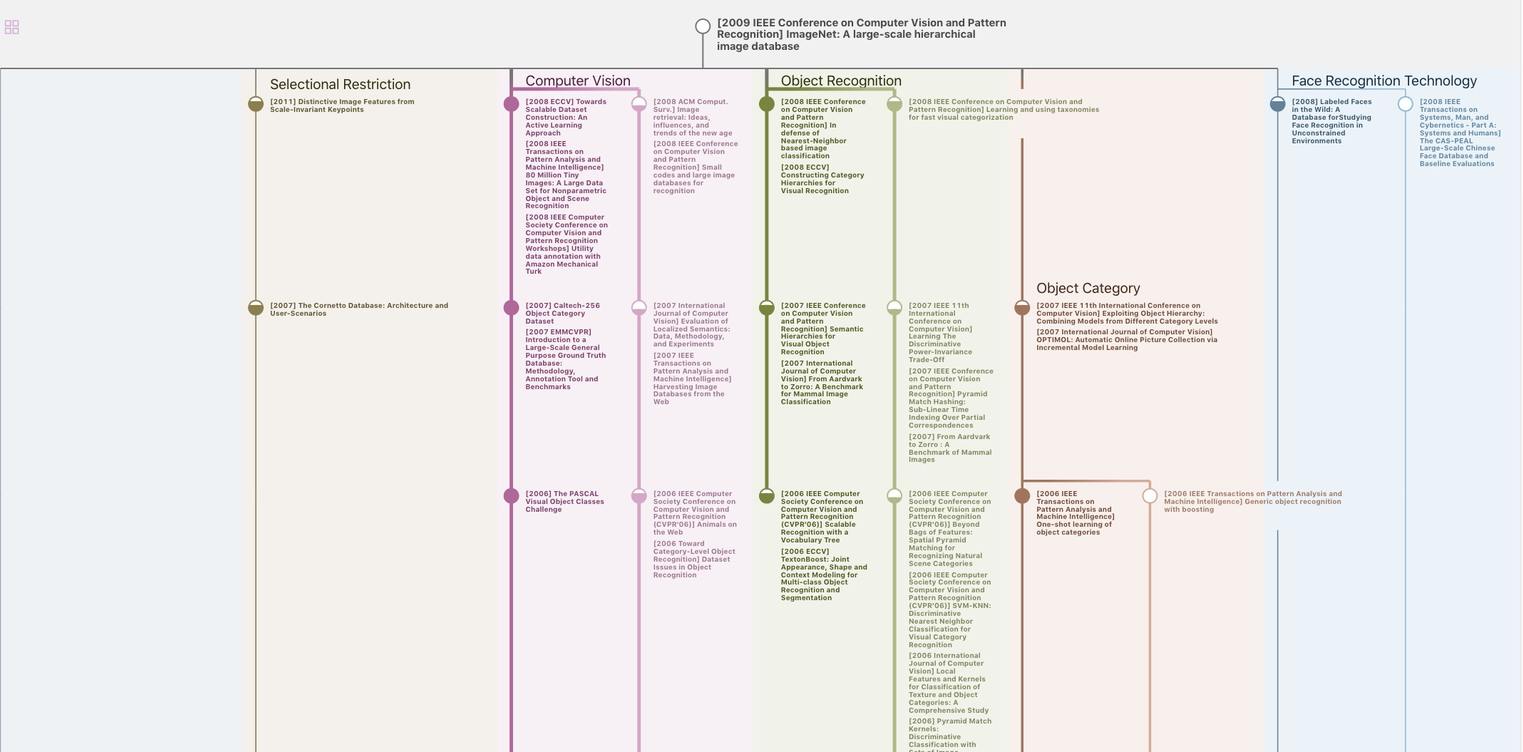
生成溯源树,研究论文发展脉络
Chat Paper
正在生成论文摘要