Robust Weakly Supervised Learning Prestack Multitrace Seismic Inversion.
IEEE Transactions on Geoscience and Remote Sensing(2024)
摘要
Pre-stack seismic inversion is one of the most commonly used methods to describe reservoirs. Deep learning (DL) has received wide attention because of its ability to handle complex nonlinear problems. The existing DL-based seismic inversion method adopts a single training dataset, which is prone to poor generalizability and geological artifact. In addition, field data are often difficult to transfer learning due to the lack of a training dataset. To partially overcome these problems, we improve the training dataset and network parameters, thus developing a new DL-based pre-stacked inversion framework. First, we generate a training dataset using a forward model and pre-train the network by adding labels to a portion of it as the training dataset. We use multi-trace information for training and prediction to reduce the lateral geological artifact problem. For unlabeled or less-labeled datasets, we construct data residual loss terms and initial model constraints to achieve weakly supervised or even unsupervised learning, which solves the poor generalizability problem due to insufficient training datasets. Compared with previous data-driven approaches, the inverse framework in this paper compensates for the lack of the training dataset, diminishes geological artifacts, and improves generalization and robustness. The effectiveness of the framework is verified by synthetic data from two different models and field data from S-fields, and good inversion results are obtained even with low signal-to-noise ratio or lack of well data.
更多查看译文
关键词
Pre-stack inversion,data residual loss terms,Weakly supervised
AI 理解论文
溯源树
样例
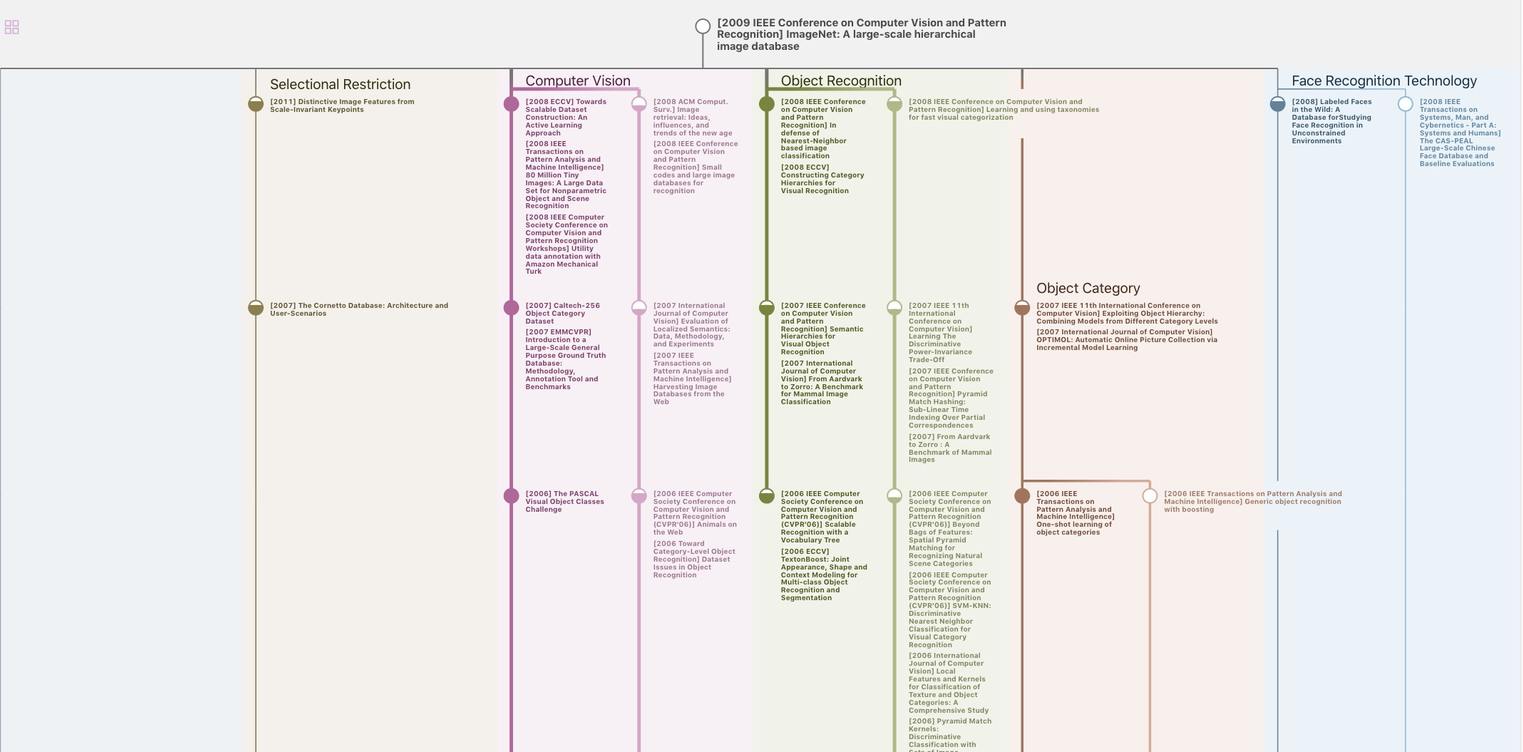
生成溯源树,研究论文发展脉络
Chat Paper
正在生成论文摘要