Data-Driven Robust Adaptive Control With Deep Learning for Wastewater Treatment Process
IEEE TRANSACTIONS ON INDUSTRIAL INFORMATICS(2024)
摘要
Owing to high complexity and time-variant operation, as well as increasingly requirements for water quality, stability, and reliability, the wastewater treatment process (WWTP) is regarded as an adaptive control problem. In this study, a data-driven adaptive control with deep learning (DRAC-DL) is developed to improve the operational performance of the WWTP. First, a feedback controller is designed to construct the closed-loop control scheme. Second, an adaptive deep belief network (ADBN), based on the data-driven self-incremental learning strategy, is proposed to approximate the ideal control law. Third, the stability of the DRAC-DL scheme is analyzed in detail. The main advantage of DRAC-DL lies in its improved robustness and efficiency, which benefit from the Lyapunov-based closed-loop strategy and the efficient ADBN controller. Finally, the feasibility and applicability of DRAC-DL are verified by two parts: 1) simulation on the nonlinear system and 2) application to the WWTP on the benchmark simulation model No.1. The experimental results show the applicability and effectiveness, among which DRAC-DL reduces the output fluctuation (variance) by no less than 82% and realizes the better stability and robustness.
更多查看译文
关键词
Adaptive control,adaptive deep belief network (ADBN),stability analysis,wastewater treatment process (WWTP)
AI 理解论文
溯源树
样例
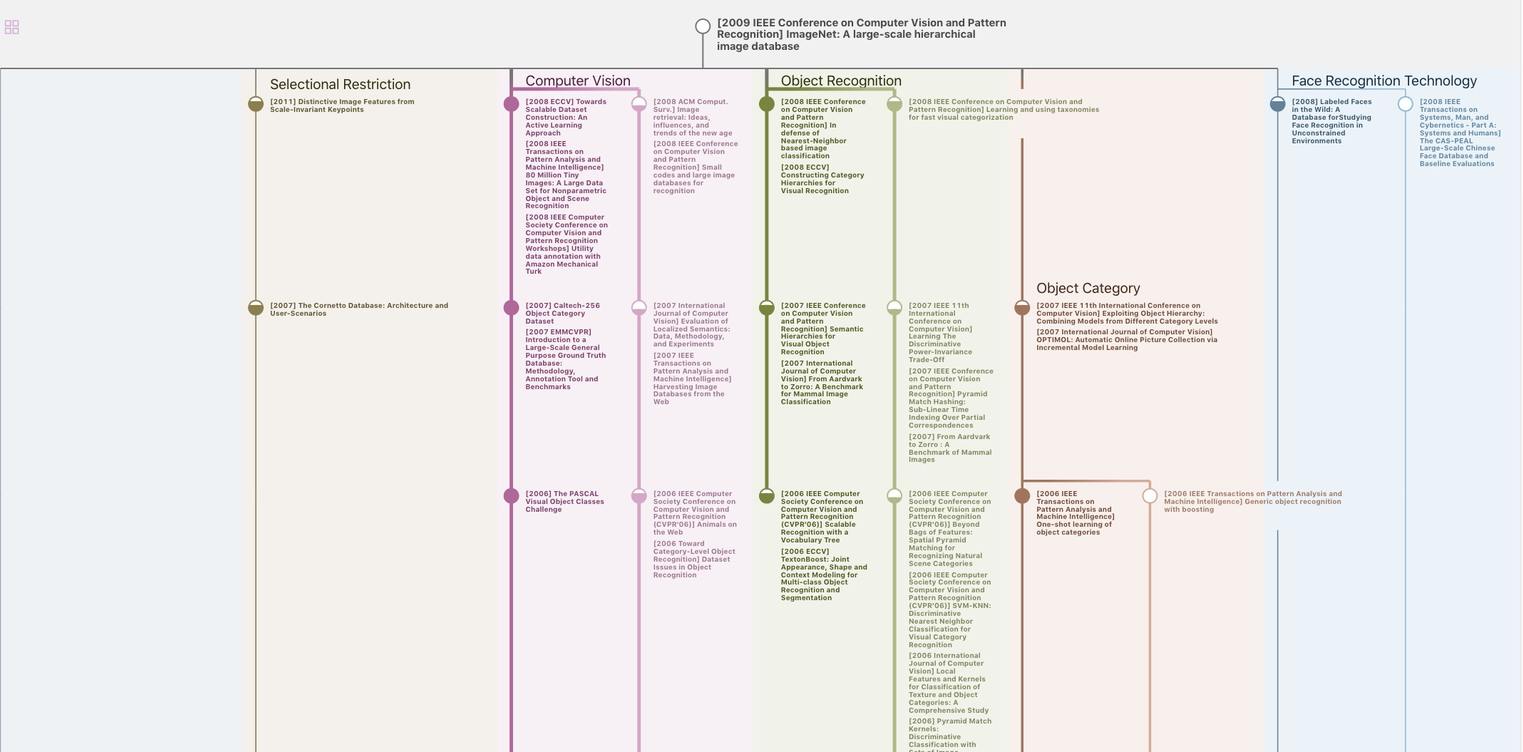
生成溯源树,研究论文发展脉络
Chat Paper
正在生成论文摘要