Protein Representation Learning via Knowledge Enhanced Primary Structure Modeling
arXiv (Cornell University)(2023)
摘要
Protein representation learning has primarily benefited from the remarkable development of language models (LMs). Accordingly, pre-trained protein models also suffer from a problem in LMs: a lack of factual knowledge. The recent solution models the relationships between protein and associated knowledge terms as the knowledge encoding objective. However, it fails to explore the relationships at a more granular level, i.e., the token level. To mitigate this, we propose Knowledge-exploited Auto-encoder for Protein (KeAP), which performs token-level knowledge graph exploration for protein representation learning. In practice, non-masked amino acids iteratively query the associated knowledge tokens to extract and integrate helpful information for restoring masked amino acids via attention. We show that KeAP can consistently outperform the previous counterpart on 9 representative downstream applications, sometimes surpassing it by large margins. These results suggest that KeAP provides an alternative yet effective way to perform knowledge enhanced protein representation learning.
更多查看译文
关键词
knowledge enhanced primary structure
AI 理解论文
溯源树
样例
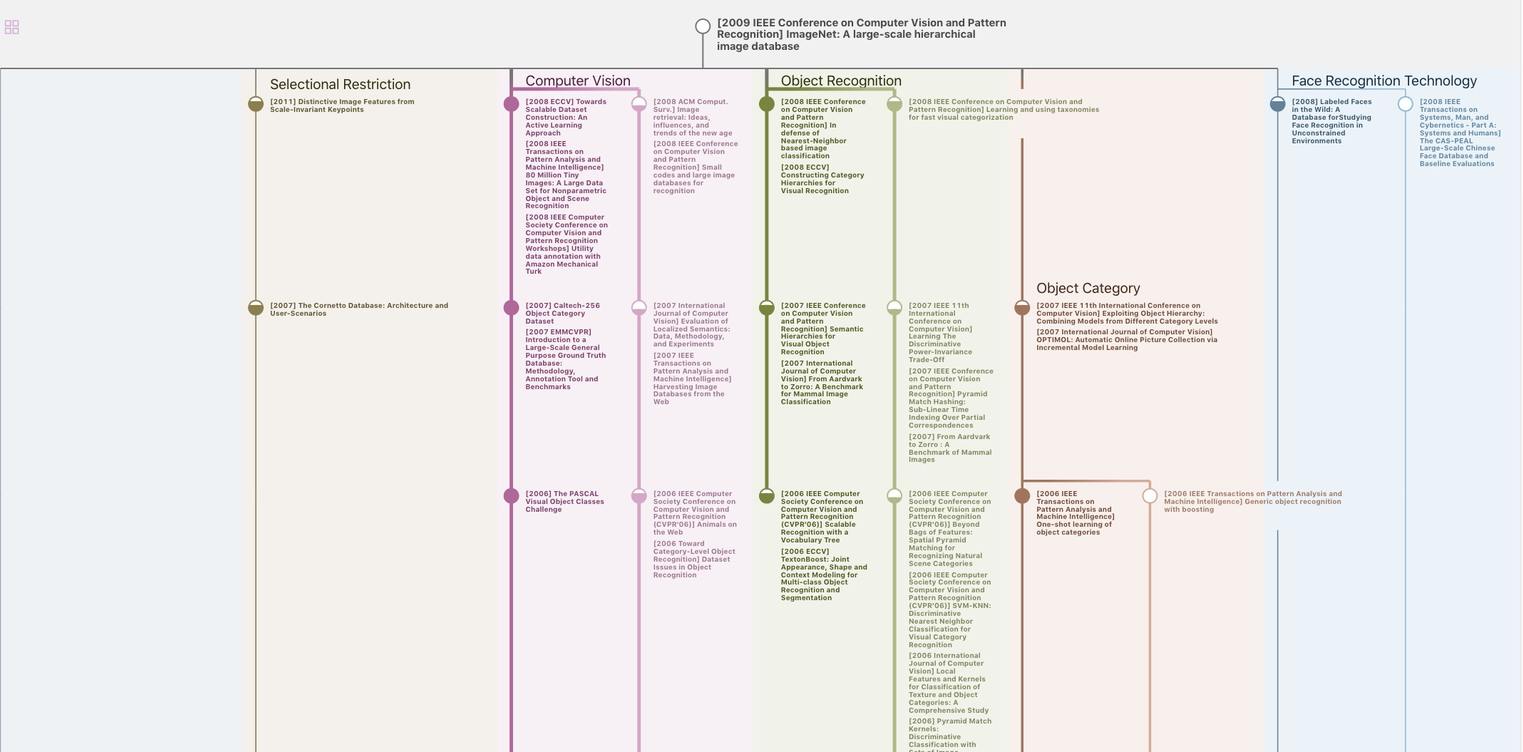
生成溯源树,研究论文发展脉络
Chat Paper
正在生成论文摘要