Abstract: Trainable Joint Bilateral Filters for Enhanced Prediction Stability in Low-dose CT.
Bildverarbeitung für die Medizin(2023)
摘要
Low-dose computed tomography (CT) denoising algorithms aim to enable reduced patient dose in routine CT acquisitions while maintaining high image quality. Recently, deep learning (DL)-based methods were introduced, outperforming conventional denoising algorithms on this task due to their high model capacity. However, for the transition of DL-based denoising to clinical practice, these data-driven approaches must generalize robustly beyond the seen training data. We, therefore, propose a hybrid denoising approach consisting of a set of trainable joint bilateral filters (JBFs) combined with a convolutional DL-based denoising network to predict the guidance image. Our proposed denoising pipeline combines the high model capacity enabled by DL-based feature extraction with the reliability of the conventional JBF. The pipeline’s ability to generalize is demonstrated by training on abdomen CT scans without metal implants and testing on abdomen scans with metal implants as well as on head CT data. When embedding RED-CNN/QAE, two well-established DL-based denoisers in our pipeline, the denoising performance is improved by 10%/82% (RMSE) and 3%/81% (PSNR) in regions containing metal and by 6%/78% (RMSE) and 2%/4% (PSNR) on head CT data, compared to the respective vanilla model. Concluding, the proposed trainable JBFs limit the error bound of deep neural networks to facilitate the applicability of DL-based denoisers in low-dose CT pipelines.We made our trainable bilateral filter layer package (PyTorch, GPU accelerated) publicly available [1, 2].
更多查看译文
关键词
trainable joint bilateral filters,enhanced prediction stability,abstract,low-dose
AI 理解论文
溯源树
样例
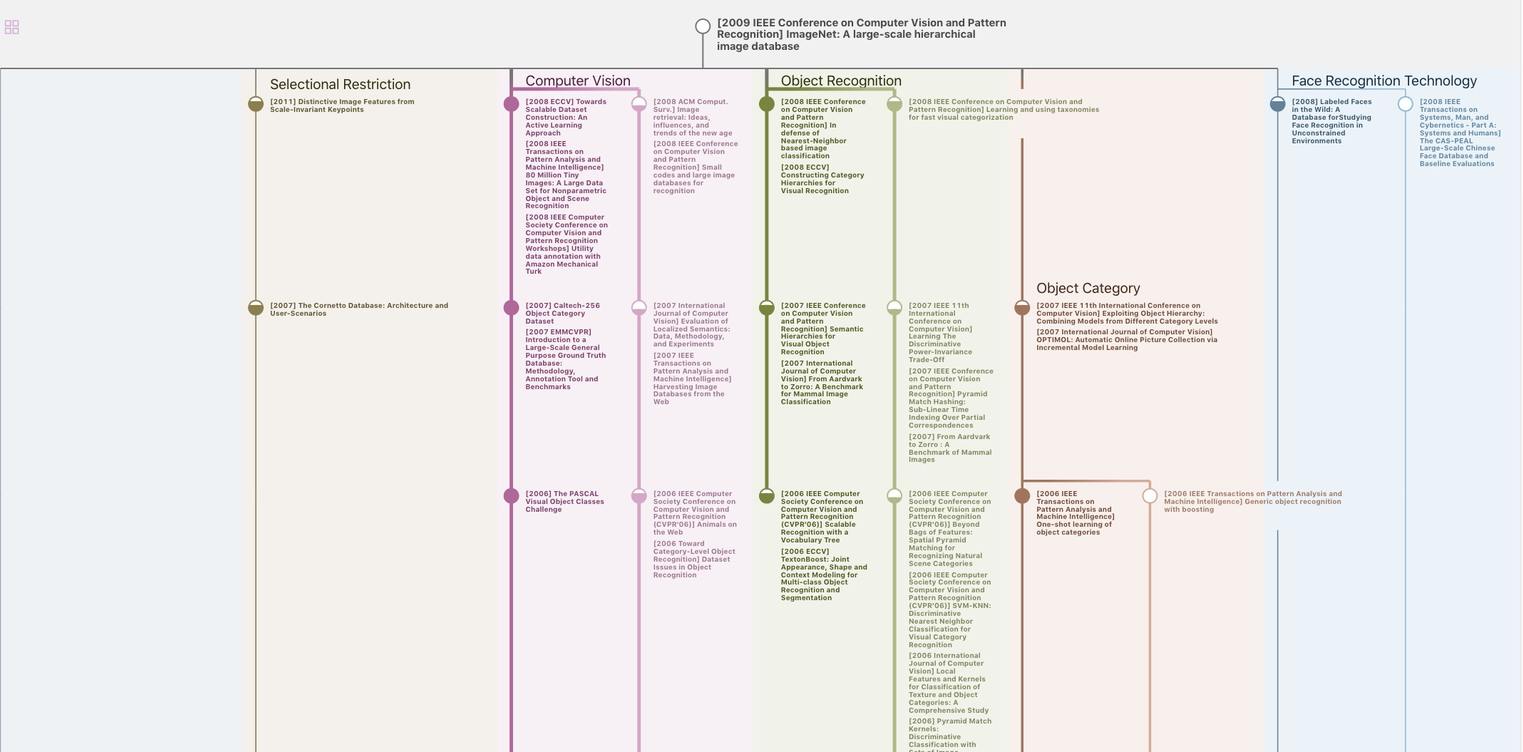
生成溯源树,研究论文发展脉络
Chat Paper
正在生成论文摘要