Predictive uncertainty on astrophysics recovery from multifield cosmology
Journal of Cosmology and Astroparticle Physics(2023)
摘要
We investigate how the constraints on cosmological and astrophysical parameters ($\Omega_{\rm m}$, $\sigma_{8}$, $A_{\rm SN1}$, $A_{\rm SN2}$) vary when exploiting information from multiple fields in cosmology. We make use of a convolutional neural network to retrieve the salient features from different combinations of field maps from IllustrisTNG in the CAMELS project. The fields considered are neutral hydrogen (HI), gas density (Mgas), magnetic fields (B) and gas metallicity (Z). We estimate the predictive uncertainty on the predictions of our model by using Monte Carlo dropout, a Bayesian approximation. Results show that overall, the performance of the model improves on all parameters as the number of channels of its input is increased. As compared to previous works, our model is able to predict the astrophysical parameters with up to $5\%$ higher in accuracy. In the best setup which includes all fields (four channel input, Mgas-HI-B-Z) the model achieves $R^{2} > 0.96$ on all parameters. Similarly, we find that the total uncertainty, which is dominated by the aleatoric uncertainty, decreases as more fields are used to train the model in general. The uncertainties obtained by dropout variational inference are overestimated on all parameters in our case, in that the predictive uncertainty is much larger than the actual squared error. After calibration, which consists of a simple $\sigma$ scaling method, the average deviation of the total uncertainty from the actual error goes down to $25\%$ at most (on $A_{\rm SN1}$).
更多查看译文
关键词
multifield cosmology,astrophysics recovery,predictive uncertainty
AI 理解论文
溯源树
样例
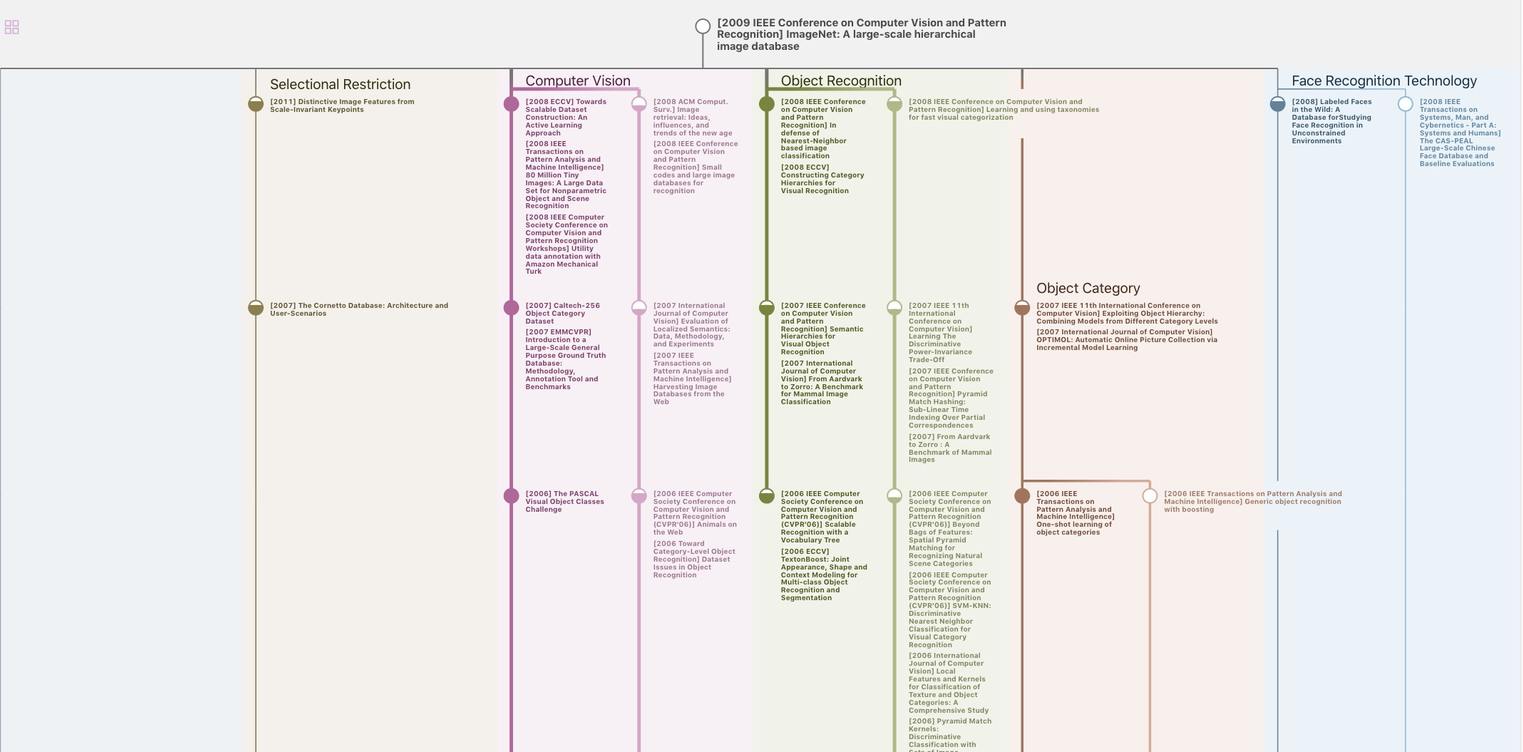
生成溯源树,研究论文发展脉络
Chat Paper
正在生成论文摘要