Refined Deep Transfer Learning with CNN-LSTM and SDAE for Adaptive Assessment of Power System Transient Stability with Time Series Data
Authorea (Authorea)(2023)
摘要
Transient stability assessment (TSA) plays a critical role in ensuring the reliable operation of power systems. However, existing approaches for TSA often encounter challenges such as data imbalances, limited sample sizes, and the need for adaptability in the face of system changes, necessitating the exploration of more advanced techniques. This paper proposes a novel deep transfer learning (DTL) framework to address these limitations that incorporates CNN-LSTM and stacked denoising auto-encoder (SDAE) techniques, aiming to significantly improve the speed and accuracy of power system TSA, especially in online applications and adaptability to system changes. First, the utilization of SDAE enables effective feature extraction, while the implementation of class weight balancing and cross-entropy loss function techniques effectively addresses data imbalances. Second, a CNN-LSTM classifier is constructed using transfer progressive learning. This approach allows for the effective analysis of spatial and temporal dynamic measurements by leveraging unsupervised pre-training (auto-encoder) and additional CNN-LSTM layers. Third, we propose the DTL, which leverages knowledge transfer from the CNN-LSTM model and incorporates fine-tuning techniques. This innovative approach ensures adaptability under in four scenarios, which is a prevalent challenge in power systems for continuous prediction. As compared with other techniques, the results demonstrate that our proposed approach achieves TSA accuracy of up to 99.68% on the IEEE 39-bus system and 99.80% on the South Carolina 500-bus system. Furthermore, to compare the performance of continuous prediction with other methods, our proposed method exhibits a significant improvement of 2% even with a limited sample size.
更多查看译文
关键词
power system transient stability,power system,adaptive assessment,cnn-lstm
AI 理解论文
溯源树
样例
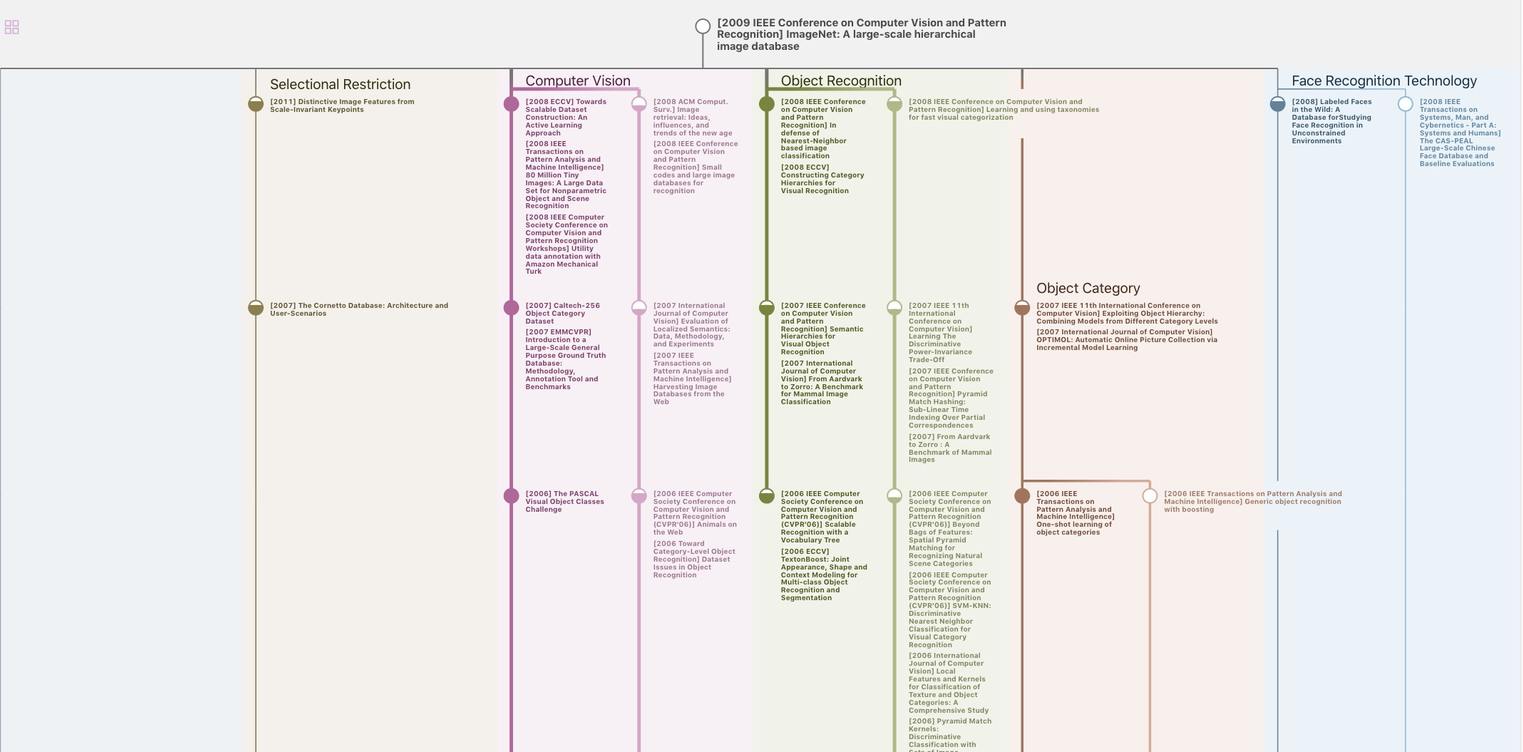
生成溯源树,研究论文发展脉络
Chat Paper
正在生成论文摘要