Central Attention with Multi-Graphs for Image Annotation
Neural Processing Letters(2024)
摘要
In recent decades, the development of multimedia and computer vision has sparked significant interest among researchers in the field of automatic image annotation. However, much of the research has primarily focused on using a single graph for annotating images in semi-supervised learning. Conversely, numerous approaches have explored the integration of multi-view or image segmentation techniques to create multiple graph structures. Yet, relying solely on a single graph proves to be challenging, as it struggles to capture the complete manifold of structural information. Furthermore, the computational complexity of building multiple graph structures based on multi-view or image segmentation is substantial and time-consuming. To address these issues, we propose a novel method called "Central Attention with Multi-graphs for Image Annotation." Our approach emphasizes the critical role of the central image region in the annotation process. Remarkably, we demonstrate that impressive performance can be achieved by leveraging just two graph structures, composed of central and overall features, in semi-supervised learning. To validate the effectiveness of our proposed method, we conducted a series of experiments on benchmark datasets, including Corel5K, ESPGame, and IAPRTC12. These experiments provide empirical evidence of our method’s capabilities.
更多查看译文
关键词
Automatic image annotation,Lplacian feature mapping,Multi-graphs,Central attention
AI 理解论文
溯源树
样例
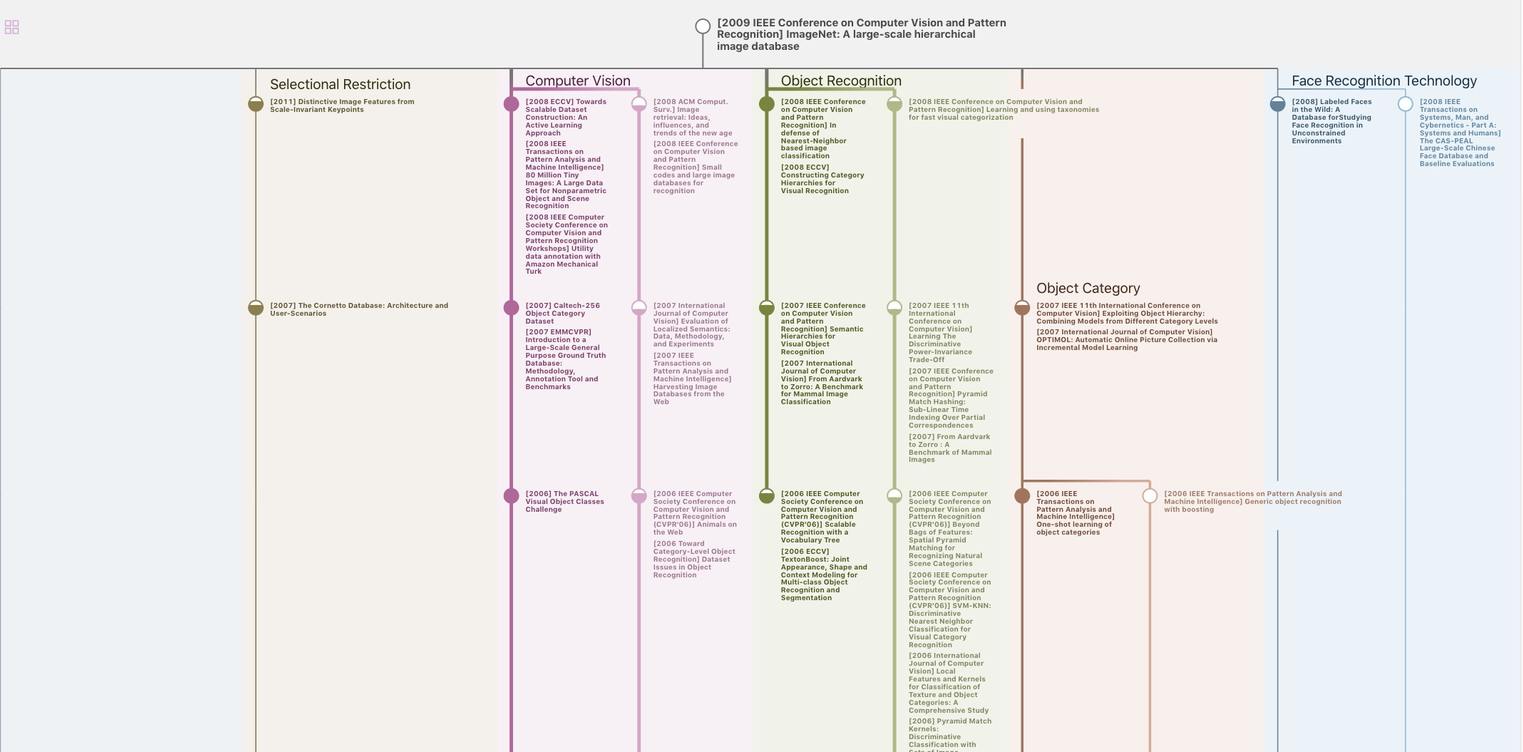
生成溯源树,研究论文发展脉络
Chat Paper
正在生成论文摘要