Enhancing Representation Learning for Periodic Time Series with Floss: A Frequency Domain Regularization Approach
arXiv (Cornell University)(2023)
摘要
Time series analysis is a fundamental task in various application domains, and deep learning approaches have demonstrated remarkable performance in this area. However, many real-world time series data exhibit significant periodic or quasi-periodic dynamics that are often not adequately captured by existing deep learning-based solutions. This results in an incomplete representation of the underlying dynamic behaviors of interest. To address this gap, we propose an unsupervised method called Floss that automatically regularizes learned representations in the frequency domain. The Floss method first automatically detects major periodicities from the time series. It then employs periodic shift and spectral density similarity measures to learn meaningful representations with periodic consistency. In addition, Floss can be easily incorporated into both supervised, semi-supervised, and unsupervised learning frameworks. We conduct extensive experiments on common time series classification, forecasting, and anomaly detection tasks to demonstrate the effectiveness of Floss. We incorporate Floss into several representative deep learning solutions to justify our design choices and demonstrate that it is capable of automatically discovering periodic dynamics and improving state-of-the-art deep learning models.
更多查看译文
关键词
frequency domain regularization approach,periodic time series,representation learning,frequency domain
AI 理解论文
溯源树
样例
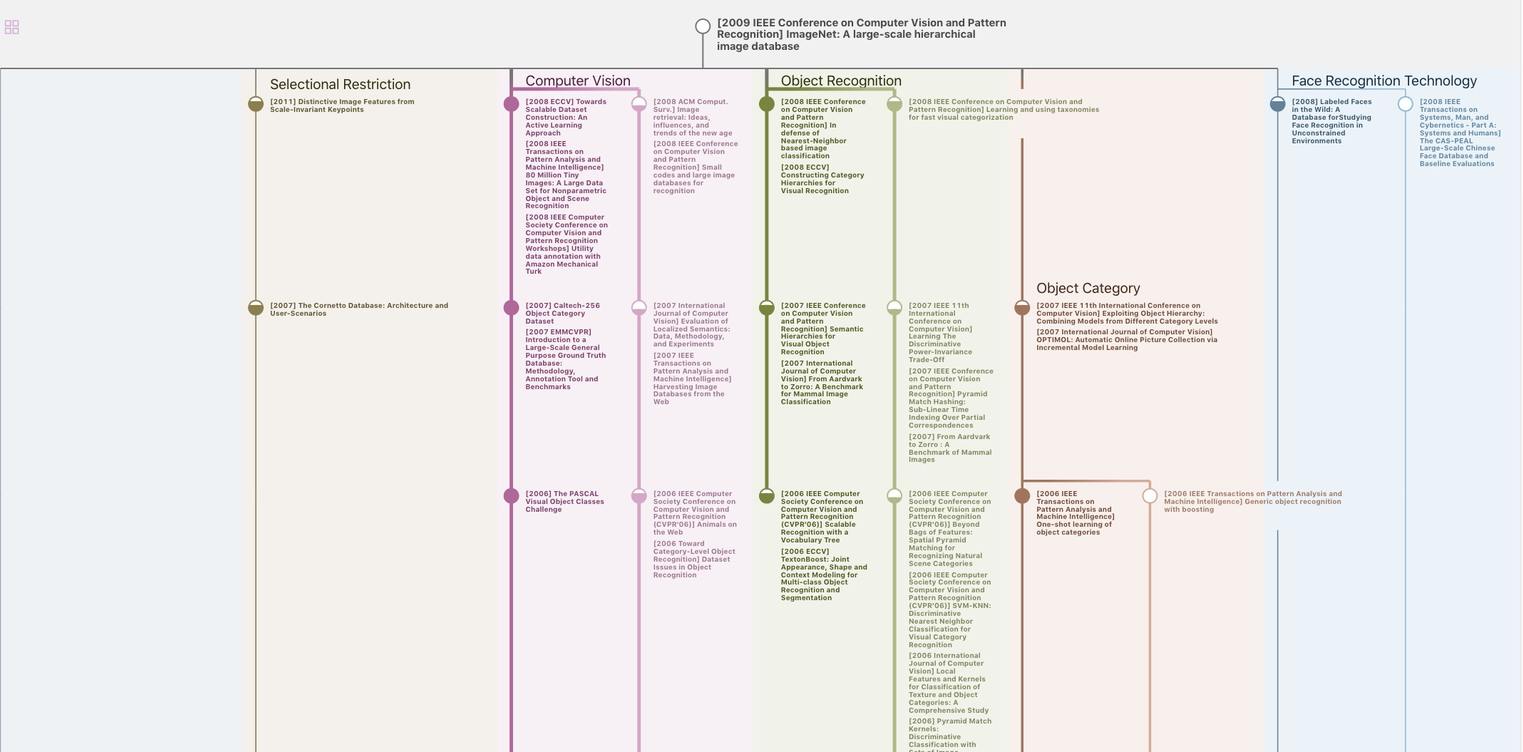
生成溯源树,研究论文发展脉络
Chat Paper
正在生成论文摘要