A hybrid spatiotemporal deep belief network and sparse representation-based framework reveals multilevel core functional components in decoding multitask fMRI signals
NETWORK NEUROSCIENCE(2023)
摘要
Decoding human brain activity on various task-based functional brain imaging data is of great significance for uncovering the functioning mechanism of the human mind. Currently, most feature extraction model-based methods for brain state decoding are shallow machine learning models, which may struggle to capture complex and precise spatiotemporal patterns of brain activity from the highly noisy fMRI raw data. Moreover, although decoding models based on deep learning methods benefit from their multilayer structure that could extract spatiotemporal features at multiscale, the relatively large populations of fMRI datasets are indispensable, and the explainability of their results is elusive. To address the above problems, we proposed a computational framework based on hybrid spatiotemporal deep belief network and sparse representations to differentiate multitask fMRI (tfMRI) signals. Using a relatively small cohort of tfMRI data as a test bed, our framework can achieve an average classification accuracy of 97.86% and define the multilevel temporal and spatial patterns of multiple cognitive tasks. Intriguingly, our model can characterize the key components for differentiating the multitask fMRI signals. Overall, the proposed framework can identify the interpretable and discriminative fMRI composition patterns at multiple scales, offering an effective methodology for basic neuroscience and clinical research with relatively small cohorts. Decoding different cognitive processes using task-based functional magnetic resonance imaging (tfMRI) is crucial for understanding the relationship between brain activities and cognitive states. However, existing machine learning-based feature extraction methods for decoding brain states may struggle to capture the complex and precise spatiotemporal patterns of brain activity from the highly noisy raw fMRI data. Additionally, current deep learning-based end-to-end decoding models struggle to unveil interpretable components in tfMRI signal decoding. To address these limitations, we proposed a novel framework, the hybrid spatiotemporal deep belief network and sparse representations (DBN-SR) framework, which effectively distinguished multitask fMRI signals with an average accuracy of 97.86%. Furthermore, it simultaneously identified multilevel temporal and spatial patterns of multiple cognitive tasks. By utilizing a novel ratio-of-activation metric, our framework unveiled interpretable components with greater classification capacity, offering an effective methodology for basic neuroscience and clinical research.
更多查看译文
关键词
Multitask classification,Task-based fMRI,Deep belief network,Sparse representation,Functional brain network
AI 理解论文
溯源树
样例
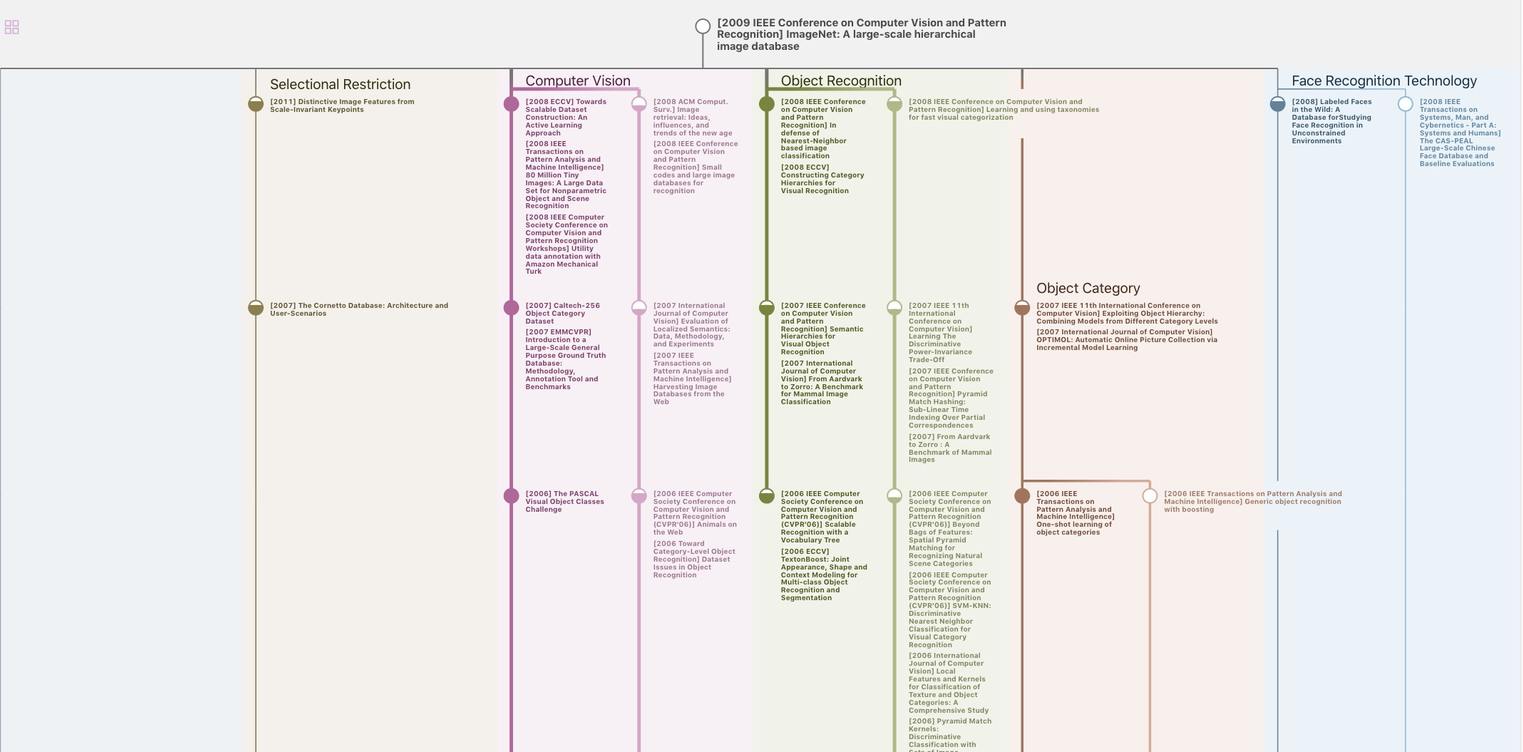
生成溯源树,研究论文发展脉络
Chat Paper
正在生成论文摘要