Physics-constrained Data-Driven Variational method for discrepancy modeling
COMPUTER METHODS IN APPLIED MECHANICS AND ENGINEERING(2023)
摘要
A data-driven discrepancy modeling method is presented that variationally embeds measured data in the modeling and analysis framework. The proposed method exploits the variationally derived loss function that is comprised of the residual between the first-principles theory and sensor-based measurements to augment the physics-based model. The method was first developed in the context of linear elasticity (Masud and Goraya, J. Appl. Mech. 89 (11) 2022) wherein the relation between the discrepancy model and the loss terms was derived to show that the data embedding terms behave like residual-based least-squares regression functions. An interpretation of the stabilization tensor as a kernel function was formally established and its role in assimilating a-priori knowledge of the problem in the modeling method was highlighted. The present paper employs linear elastodynamics as a model problem where Data-Driven Variational (DDV) method incorporates high-fidelity data into forward calculations. This results in driving the problem with not only the boundary and initial conditions, but also with the sensor data that is available at only a small subset of the total domain. The effect of the loss function on the time-dependent response of the system is investigated under a variety of loading conditions and model discrepancies. The energy and Morlet wavelet analyses reveal that the problem with embedded data recovers the energy and the fundamental frequency band of the target system. Time histories of strain energy and kinetic energy of a cantilever beam undergoing damped oscillations are recovered by including known data in an undamped model. This highlights the discrepancy modeling attributes of the method under combined effects of parameter and model discrepancy.(c) 2023 Elsevier B.V. All rights reserved.
更多查看译文
关键词
Data-driven modeling,Physics-constrained modeling,Discrepancy modeling,Variationally derived Loss Function,Variationally embedded measured data,Elastodynamics
AI 理解论文
溯源树
样例
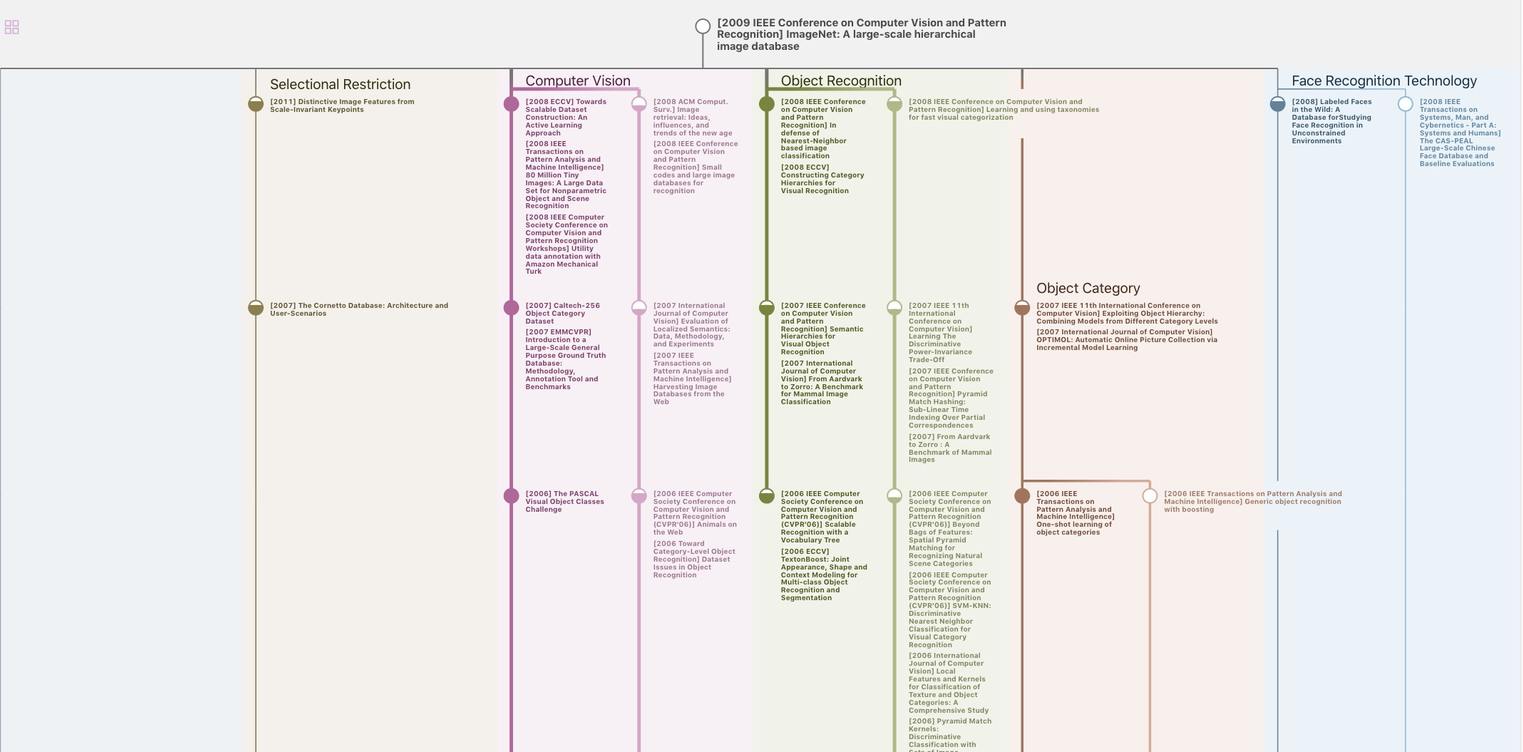
生成溯源树,研究论文发展脉络
Chat Paper
正在生成论文摘要