Exploring Structure Incentive Domain Adversarial Learning for Generalizable Sleep Stage Classification
ACM TRANSACTIONS ON INTELLIGENT SYSTEMS AND TECHNOLOGY(2024)
摘要
Sleep stage classification is crucial for sleep state monitoring and health interventions. In accordance with the standards prescribed by the American Academy of Sleep Medicine, a sleep episode follows a specific structure comprising five distinctive sleep stages that collectively form a sleep cycle. Typically, this cycle repeats about five times, providing an insightful portrayal of the subject’s physiological attributes. The progress of deep learning and advanced domain generalization methods allows automatic and even adaptive sleep stage classification. However, applying models trained with visible subject data to invisible subject data remains challenging due to significant individual differences among subjects. Motivated by the periodic category-complete structure of sleep stage classification, we propose a Structure Incentive Domain Adversarial learning (SIDA) method that combines the sleep stage classification method with domain generalization to enable cross-subject sleep stage classification. SIDA includes individual domain discriminators for each sleep stage category to decouple subject dependence differences among different categories and fine-grained learning of domain-invariant features. Furthermore, SIDA directly connects the label classifier and domain discriminators to promote the training process. Experiments on three benchmark sleep stage classification datasets demonstrate that the proposed SIDA method outperforms other state-of-the-art sleep stage classification and domain generalization methods and achieves the best cross-subject sleep stage classification results.
更多查看译文
关键词
Deep Learning for EEG
AI 理解论文
溯源树
样例
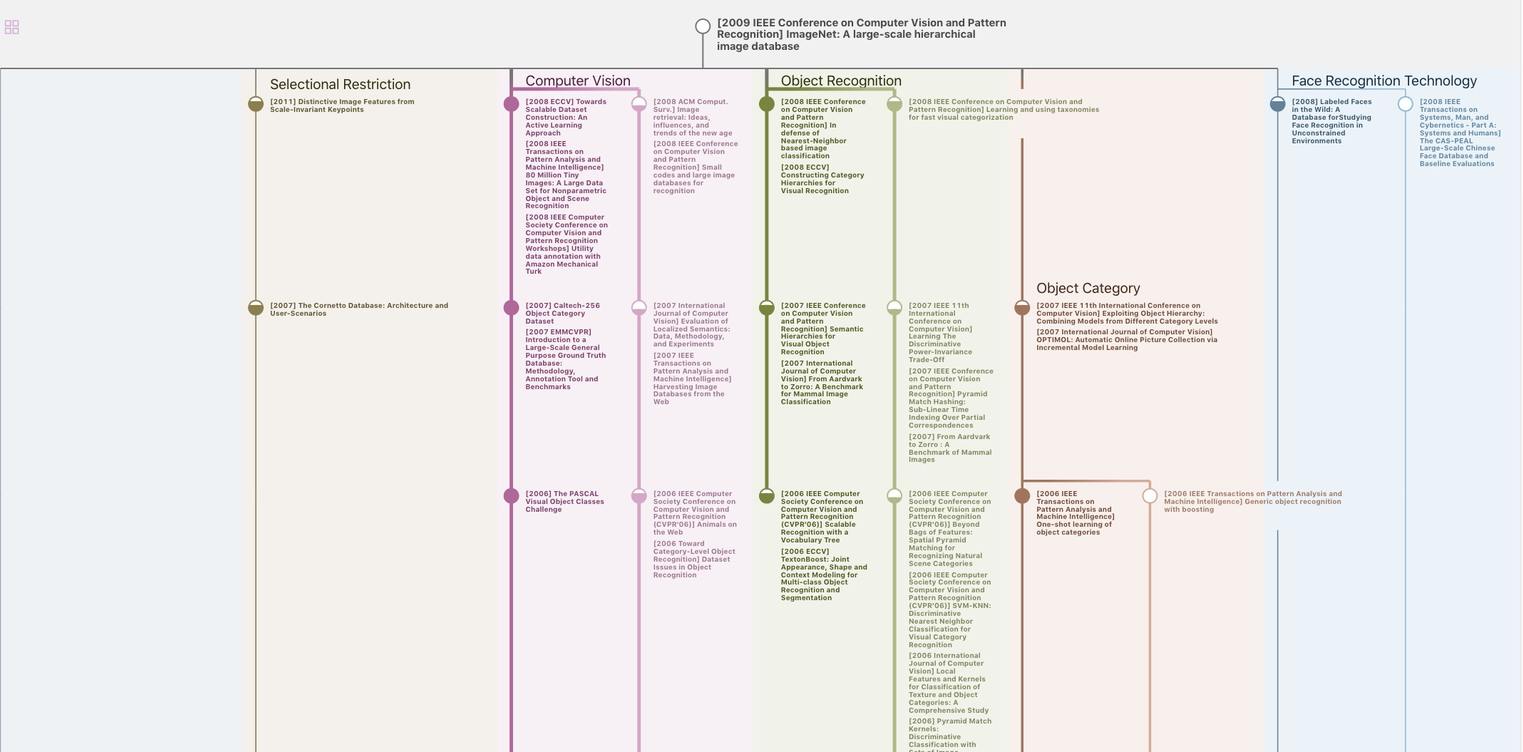
生成溯源树,研究论文发展脉络
Chat Paper
正在生成论文摘要