Modeling Electro-Erosion Wear of Cryogenic Treated Electrodes of Mold Steels Using Machine Learning Algorithms
Lecture notes in mechanical engineering(2023)
摘要
Electro-erosion wear (EEW) is a significant problem in the mold steel industry, as it can greatly reduce the lifespan of electrodes. This study presents a machine-learning approach for predicting and modeling electrode and workpiece wear on an electrical discharge machining (EDM) machine. In the experimental design, EDM of CuCrZr and Cu electrodes of AISI P20 tool steel was carried out at different pulse currents and duration levels. In addition, CuCrZr and Cu electrodes used in the experiment were cryogenically treated at a predefined degree for multiple periods and then tempered. This study employed machine learning algorithms such as decision trees, random forests, and k-nearest neighbors to model the EEW of cryogenically treated electrodes made of mold steels. The results were compared according to the coefficient of determination (R2), adjusted R2, and root mean squared error. As a result, the decision trees outperformed the other algorithms with 0.99 R2 performance. This study provides valuable insights into the behavior of EEW in mold steel electrodes and could be used to optimize the manufacturing process and extend the lifespan of the electrodes.
更多查看译文
关键词
Electrical Discharge Machining,Electrochemical Machining,Tool Wear,Metal Cutting,Machining
AI 理解论文
溯源树
样例
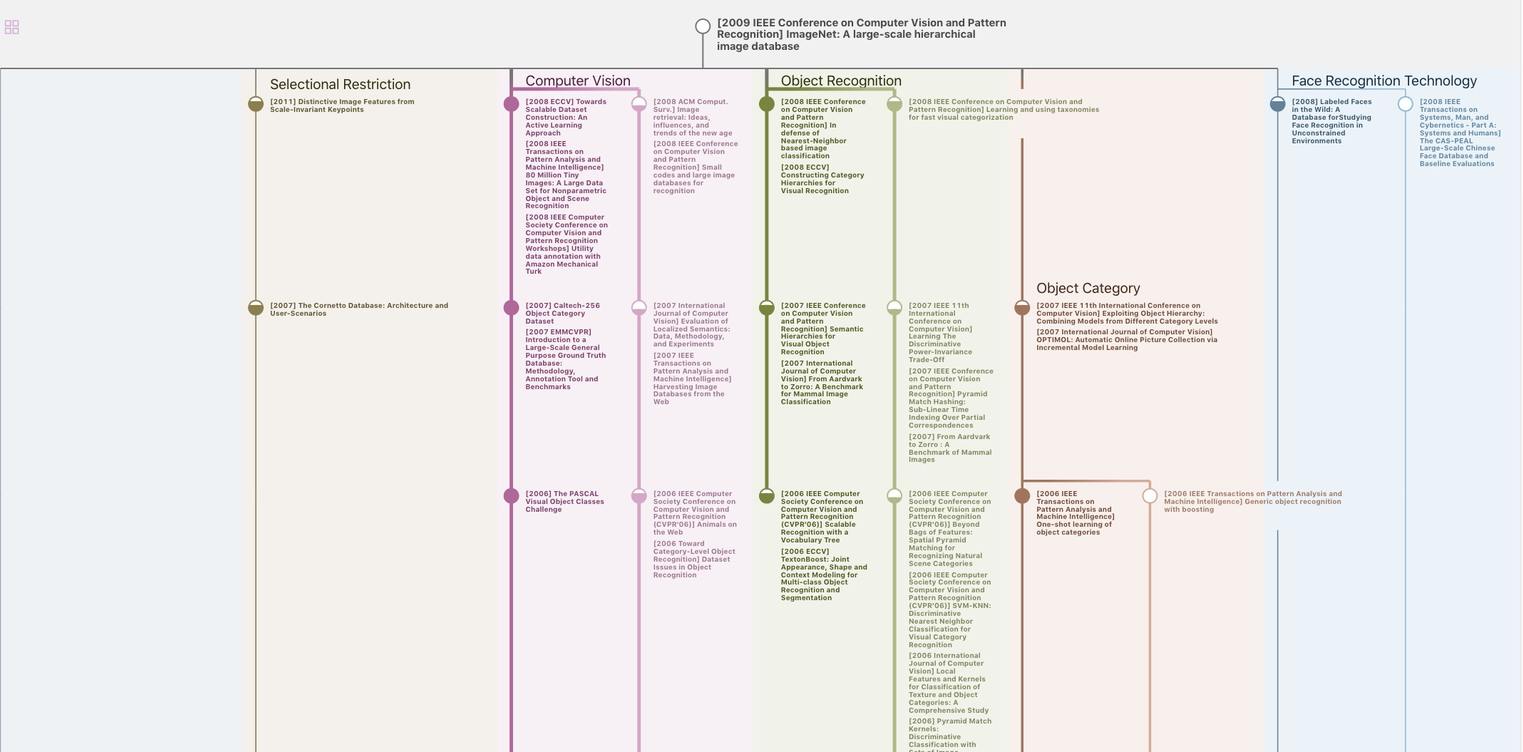
生成溯源树,研究论文发展脉络
Chat Paper
正在生成论文摘要