A morphology-based deep learning for fetal head circumference measurement
ULTRASOUND IN OBSTETRICS & GYNECOLOGY(2023)
摘要
During pregnancy, ultrasound imaging is utilised to measure fetal biometrics, including fetal head circumference. Although deep learning has been applied in numerous attempts to address this challenge, current research has not adequately considered the morphological characteristics of the fetal head. Therefore, we have developed a morphology-based artificial intelligence method for fetal head circumference measurement. Our method can effectively capture and smooth out the head boundaries. We validated the performance of our proposed model using the HC18 data set. We predicted 32 sets of data simultaneously for each pixel in the image. Each set of data contained the distance from the pixel to the centre of the fetal head and whether the corresponding pixel was inside the fetal head, guided by manually annotated head boundary information. Non-maximum suppression was applied to restore the segmentation boundary of the fetal head using this data. Our proposed method considers global information and can form a smooth segmentation boundary of the fetal head. The proposed algorithm first outputs the segmentation boundary of the entire fetal head, from which the fetal head circumference can be obtained. Based on this segmentation result, various fetal biometrics such as head circumference, ellipse long and short diameters, and skew angle can be calculated. Our results showed a mean absolute error (MAE) of 1.47 and a mean squared error (MSE) of 6.95, indicating the high accuracy of the proposed method in measuring fetal head circumference. Through the development of a deep learning algorithm for fully automatic fetal head detection, we effectively leverage the morphological information of the fetal head and propose a more efficient deep model for automatic calculation of fetal head circumference. This methodology can potentially assist in fetal status detection during maternal pregnancy, improve the diagnostic efficiency of doctors' fetal status analysis.
更多查看译文
AI 理解论文
溯源树
样例
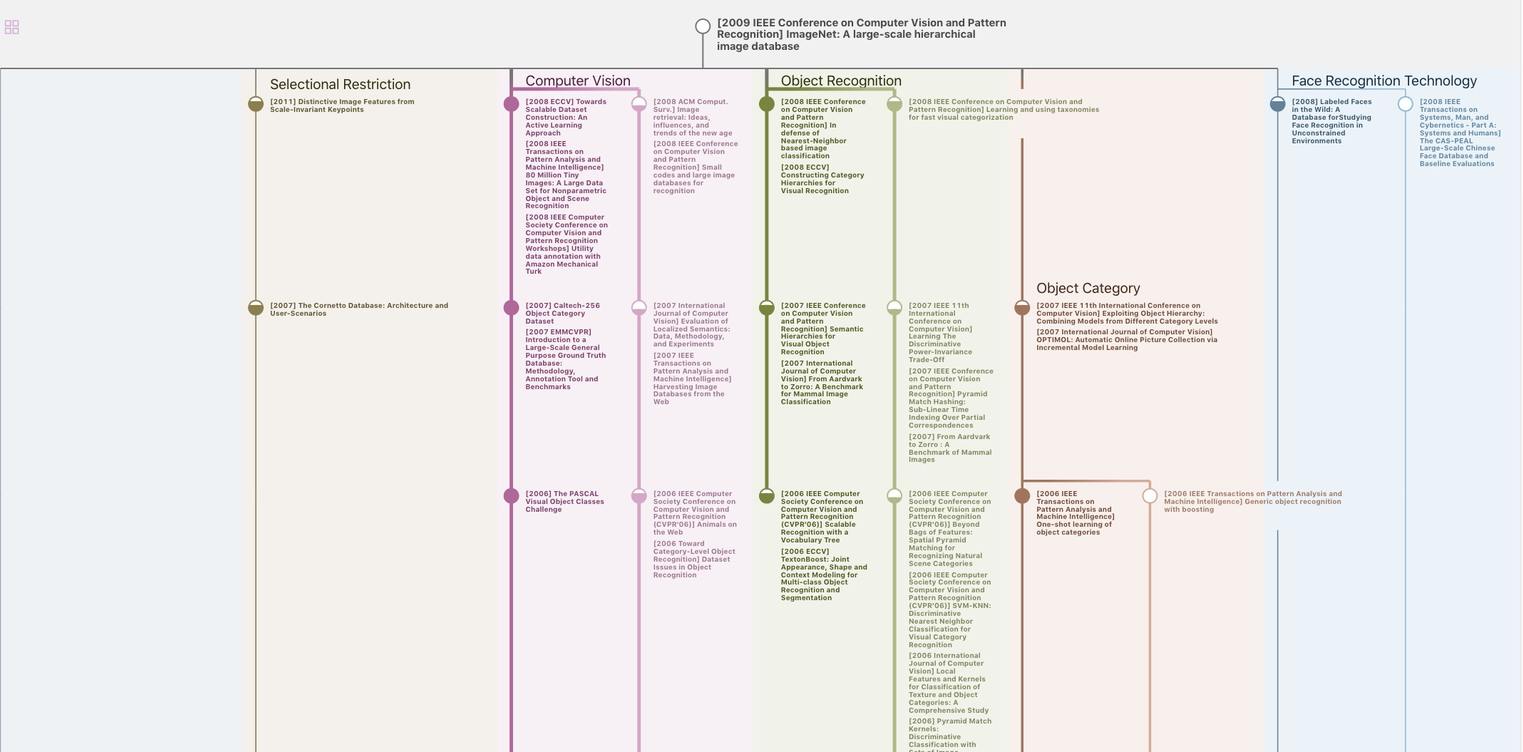
生成溯源树,研究论文发展脉络
Chat Paper
正在生成论文摘要