Neural City Maps for GNSS NLOS Prediction
Proceedings of the Satellite Division's International Technical Meeting(2023)
摘要
Tall city structures and street-side vegetation block signals from the Global Navigation Satellite Systems (GNSS) and impede localization in urban settings. To improve accuracy, GNSS-based urban localization approaches use a 3D city map. Although the field of GNSS-based urban localization with 3D city maps is broad, predicting non-line-of-sight (NLOS) effects, or signal blockage, is a core operation that impacts many techniques. An NLOS prediction approach must handle the complex urban geometry of buildings and vegetation while making quick predictions to be effective. We leverage recent advancements in computer vision and graphics to propose Neural City Maps built atop Neural Radiance Fields. Neural City Maps enable the user to capture the urban geometry at a higher fidelity, but the challenges and error sources differ from maps commonly used in GNSS-based urban localization. We develop a framework to use Neural City Maps to make NLOS predictions and evaluate several predictors to ascertain quality and consistency in light of the unique error sources of Neural City Maps. Lastly, we show overall agreement between residuals from weighted least squares and NLOS prediction from the Neural City Map using data from the Google Smartphone Decimeter Challenge.
更多查看译文
关键词
maps,prediction,city
AI 理解论文
溯源树
样例
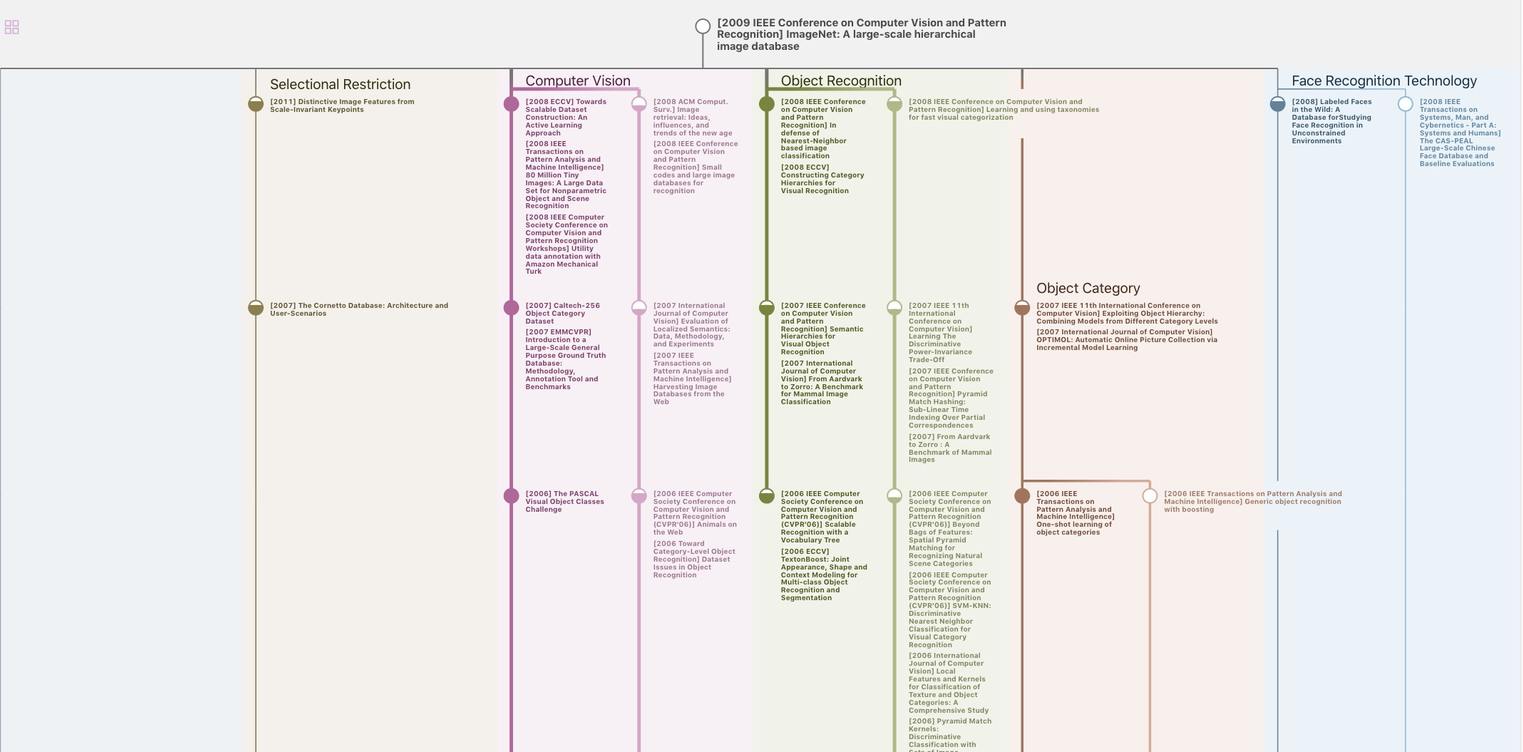
生成溯源树,研究论文发展脉络
Chat Paper
正在生成论文摘要