MCHFormer: A Multi-Cross Hybrid Former of Point-Image for 3D Object Detection.
IEEE Trans. Intell. Veh.(2024)
摘要
Mismatch often occurs between local and global information in multimodal data during downscaling transformation, which results in the loss of localization information. A Multi-Cross Hybrid Former (MCHFormer) of point-image is proposed for 3D object detection in autonomous driving, which cross-fuses LiDAR with cameras at multiple levels. Specifically, the voxelized point cloud is firstly extracted through a Dual-Stream Feature Extraction (DSFE) network. Local fine-grained area information is integrated into the global feature information, which results in a multi-layered Bird's Eye View (BEV). Meanwhile, the raw coordinates of points are incorporated into point-wise features through position coding. Then, point features are projected onto image and BEV features to obtain highly coupled multimodal information, which achieves alignment of point cloud with image information. Finally, a multi-cross Transformer fuses multiple unimodal data into a hybrid representation with more spatial awareness, which achieves accurate 3D object detection. MCHFormer are conducted extensive comparative experiments with other State-Of-The-Art (SOTA) algorithms on the KITTI, NuScenes, Waymo datasets and real road scenes. Experimental results show that the proposed algorithm not only has better accuracy and generalization capability, but also has accurate detection effect on real road scenarios.
更多查看译文
关键词
3D Object Detection,Automatic Driving,Multimodal Fusion,Multi-Cross Feature Extraction,Transformer
AI 理解论文
溯源树
样例
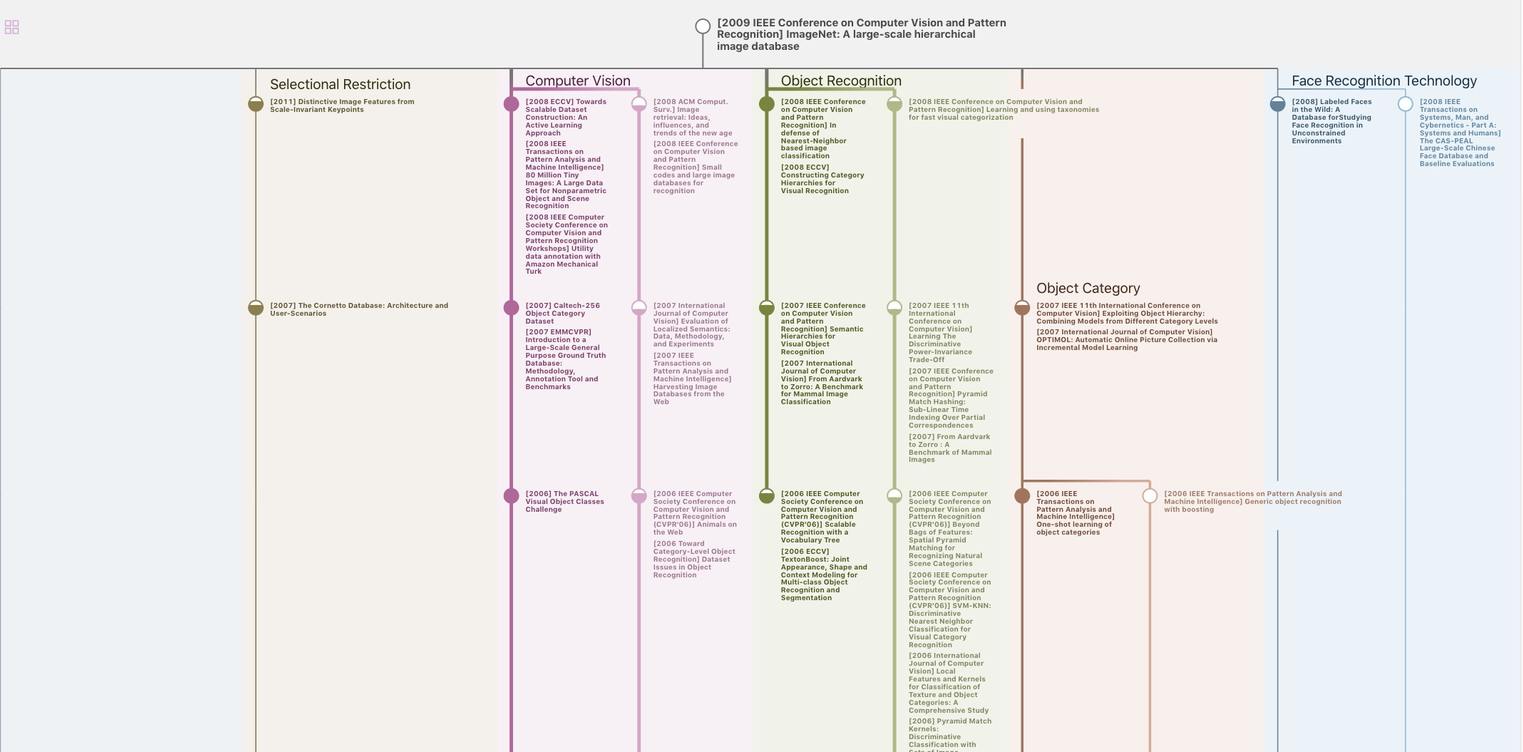
生成溯源树,研究论文发展脉络
Chat Paper
正在生成论文摘要