Moving beyond P values in The Journal of Physiology: A primer on the value of effect sizes and confidence intervals
The Journal of Physiology(2023)
摘要
Most physiology studies, including those published in The Journal of Physiology, still rely on a statistical approach known as null hypothesis significance testing (NHST). NHST informs us (via P values) of the probability of the observed or more extreme data given that the null hypothesis is true. In most cases, the null hypothesis is a statement that no difference or relationship exists (i.e. a nil hypothesis). P values below the designated error rate (typically 5% or 0.05) are called ‘significant’, with significance in this context simply meaning that the statistical computation has signified something is peculiar about the data that may merit further consideration, rather than that something ‘worthwhile’ has been discovered or observed (Caldwell & Cheuvront, 2019). Using NHST as the sole approach to interpreting findings from research has several shortcomings that have been extensively outlined in the literature (Colquhoun, 2017; Szucs & Ioannidis, 2017). Chief among these is the fact that P values alone do not provide us with the two key pieces of information for statistical inference: estimates of (1) the magnitude of the effect of interest (i.e. does it have practical, physiological or clinical relevance) and (2) the precision of that estimate (e.g. confidence intervals for effect size) (Nakagawa & Cuthill, 2007). Ultimately, it is the physiological importance of the data that those publishing in The Journal of Physiology should be most concerned with, rather than the statistical significance. Many mathematically trained statisticians are now moving away from the NHST-centric approach, and it is becoming less common in fields such as biomedical statistics, psychology and various social sciences (Altman et al., 2001; Association, 2022; Fidler et al., 2004). Some journals have gone as far as to ban the reporting of P values (Woolston, 2015). Although consensus agreement on a suitable alternative to NHST is currently lacking (Ho et al., 2019), it is clear that many of the limitations and misunderstandings caused by the isolated use of NHST can be overcome through the complementary use of other statistics, namely effect sizes and confidence intervals (Bernard, 2019; Cumming, 2014). A move towards estimation methods, which focus on effect sizes and their confidence intervals, should help shift the extant data-analysis culture in physiology away from dichotomous thinking (i.e. ‘significant’ or ‘not significant’) toward more nuanced quantitative reasoning (Ho et al., 2019). Although some journals in the field of physiology (e.g. ‘eNeuro’) have already made the step towards encouraging the use of estimation statistics (Bernard, 2019), their use is still relatively rare, perhaps because of a lack of awareness of their benefits or lack of knowledge regarding their calculation and presentation. We aim to briefly address these points below. An effect size refers simply to the magnitude of the observed effect and may be presented in unstandardised units (if the original units of measurement are meaningful) or units-free or standardised form (e.g. r statistics such as Pearson's or Spearman's, d statistics such as Cohen's d or Hedges’ g, measures of the proportion of variance accounted for such as Omega squared and comparative risk statistics such as the odds ratio) (Nakagawa & Cuthill, 2007). Although generic threshold values exist for interpreting standardised effect size statistics (e.g. small: 0.20, medium: 0.50 and large: 0.80 for Cohen's d) (Cohen, 2013), ideally, thresholds specific to each physiological domain should be empirically derived from meta-analyses (Lovakov & Agadullina, 2021). Presenting effect sizes helps to reduce P value misinterpretation; overpowered studies can identify statistically significant but trivial and physiologically meaningless effects (Nature Human Behaviour, 2023). This specific issue becomes more salient as we enter the era of ‘big data’ (Fan et al., 2014). Conversely, a result that is not statistically significant may imply that either the study was poorly designed or implemented (inadequate sample size, unreliable or inaccurate measures, etc.) or the effect size was below a level of practical/clinical/physiological significance (Kraemer, 2014). In such circumstances, effect size estimates are required to determine how the non-significant result should be interpreted, as well as to guide appraisal of the motivating theory/hypothesis. The smallest effect size of interest also needs to be specified when undertaking sample size estimation prior to the commencement of a study, to ensure adequate (statistical) power to detect effects of this magnitude. A further benefit to presenting effect sizes is that it facilitates their inclusion in future systematic reviews and meta-analyses. Such works provide a pooled estimate of effect size, inferred from research conducted to date, that is closest to the unknown common truth. Because meta-analytic estimates of effect size are considered the most trustworthy source of evidence, they are often the basis of guidance provided to practitioners (Cumming, 2013). In addition, meta-analyses facilitate the calculation of empirically derived effect size thresholds. In frequentist statistics, a 95% confidence interval indicates that if the study was repeated many times using randomly selected samples of the same size and from the same population and a confidence interval was computed for the effect statistic of interest from each of these samples, 95% of these hypothetical intervals would contain the true population value (Casella & Berger, 2021). A ‘credible interval’ is the Bayesian statistical alternative of the confidence interval and may be interpreted as an interval with a given probability (typically 95%) of containing the true value of the population parameter (Makowski et al., 2019). Both provide information about the precision of the estimate, with narrower confidence intervals indicating more precision in the population parameter estimate (Davis et al., 2021). Fortunately, most statistical software packages now provide confidence intervals by default, or else they can be derived from the information that is provided (Hopkins, 2007). Providing both the effect size and its confidence interval provides far more valuable information than the P value alone. For example, consider a study that investigates the effect of a new drug on mean systolic blood pressure (SBP) in a group of patients with hypertension. If the researchers report only the P value from their analysis (e.g. P = 0.06), both they and the reader may conclude that the new drug has had no beneficial effect. By providing the effect size and confidence intervals [e.g. a mean reduction in SBP of 10 mmHg with a 95% confidence interval of −20 to 1 mmHg], the researchers can demonstrate a large and clinically meaningful mean reduction in blood pressure that may be worthy of further investigation in a larger trial. If the same study were to report P = 0.04, the result may be considered as ‘significant’, but if the corresponding effect size and confidence intervals were a mean reduction in SBP of 3 mmHg with a 95% confidence interval of −5 to −1 mmHg, the clinical/practical value of the new drug would be considered far less promising. There are now numerous resources available for those wishing to implement estimation methods in their research. An excellent ‘beginner's instruction manual’ for effect size and confidence interval calculation, including key technical considerations relevant to physiologists, can be found in Nakagawa and Cuthill (2007); we would highly recommend reading this paper as a starting point. The clear benefits of using estimation statistics are also elegantly described and illustrated with examples by Calin-Jageman and Cumming (2019). There are numerous web applications available to calculate effect sizes and their confidence intervals (e.g. https://www.campbellcollaboration.org/escalc), as well as plot experimental data from an estimation statistics perspective, such as the excellent https://www.estimationstats.com (Ho et al., 2019). These websites are extremely user-friendly, allowing easy adaptation to this method. We would also encourage collaboration with a statistician who is familiar with these concepts and their involvement in the research process at an early stage whenever possible. Greater collaboration with trained statisticians will help avoid statistical errors that can occur during study design (e.g. performing appropriate a priori sample size estimations), data analysis and statistical reporting (Sainani et al., 2021). There is a growing acknowledgement of the shortcomings of NHST and the benefits of an estimation approach. The effect size and confidence interval tell us everything a P value does about a result, and so much more. We strongly recommend the use of estimation methods for those publishing in The Journal of Physiology; doing so will undoubtedly enhance the interpretation of research findings and improve the synthesis of results across our field. Please note: The publisher is not responsible for the content or functionality of any supporting information supplied by the authors. Any queries (other than missing content) should be directed to the corresponding author for the article. No competing interests declared. S.W., R.C. and K.T. were responsible for the conception or design of the work and drafting the work or revising it critically for important intellectual content. All authors approved the version of the manuscript submitted for publication. All authors agree to be accountable for all aspects of the work. No funding was received.
更多查看译文
关键词
effect sizes,physiology</i>,values
AI 理解论文
溯源树
样例
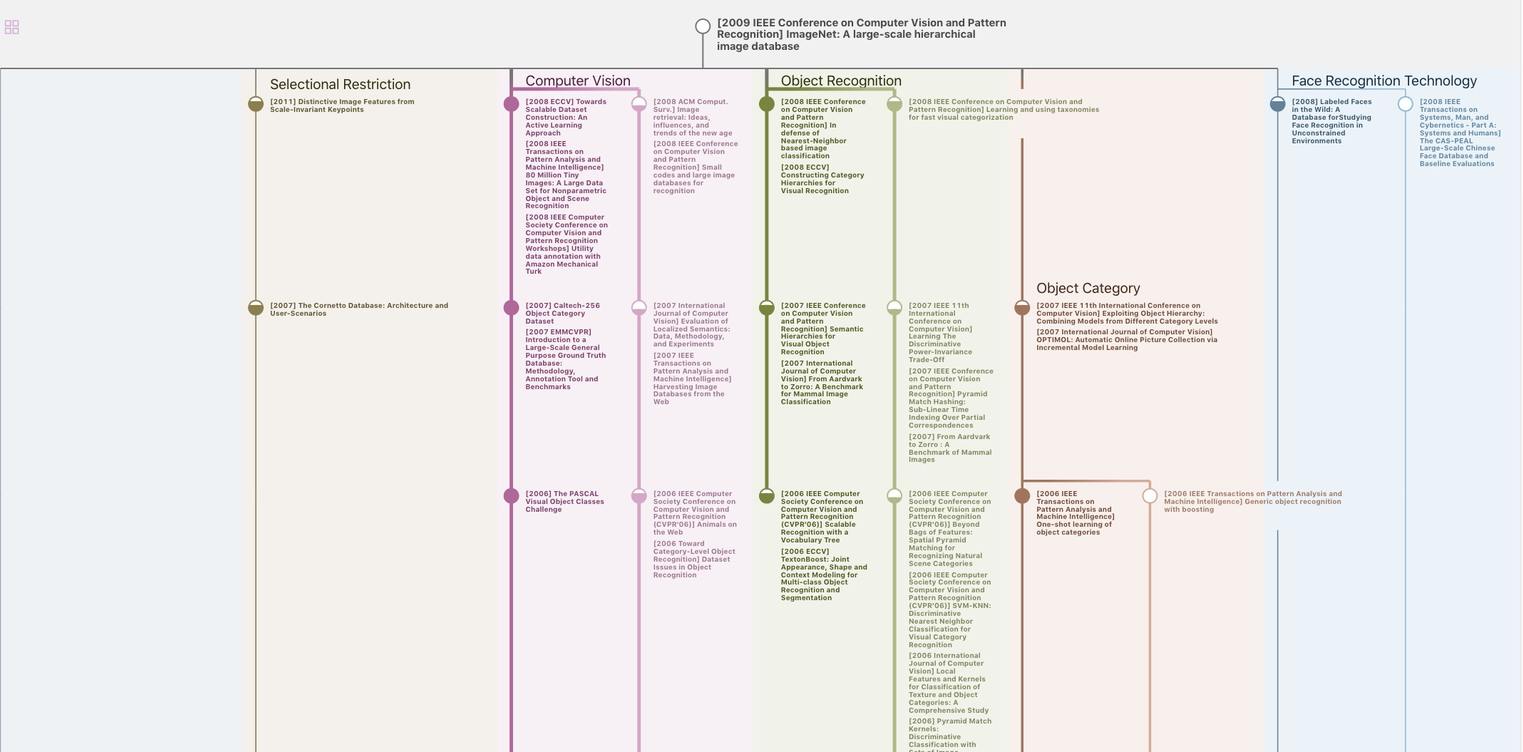
生成溯源树,研究论文发展脉络
Chat Paper
正在生成论文摘要