An Approach for Multi-Object Tracking with Two-Stage Min-Cost Flow
arXiv (Cornell University)(2023)
摘要
The minimum network flow algorithm is widely used in multi-target tracking. However, the majority of the present methods concentrate exclusively on minimizing cost functions whose values may not indicate accurate solutions under occlusions. In this paper, by exploiting the properties of tracklets intersections and low-confidence detections, we develop a two-stage tracking pipeline with an intersection mask that can accurately locate inaccurate tracklets which are corrected in the second stage. Specifically, we employ the minimum network flow algorithm with high-confidence detections as input in the first stage to obtain the candidate tracklets that need correction. Then we leverage the intersection mask to accurately locate the inaccurate parts of candidate tracklets. The second stage utilizes low-confidence detections that may be attributed to occlusions for correcting inaccurate tracklets. This process constructs a graph of nodes in inaccurate tracklets and low-confidence nodes and uses it for the second round of minimum network flow calculation. We perform sufficient experiments on popular MOT benchmark datasets and achieve 78.4 MOTA on the test set of MOT16, 79.2 on MOT17, and 76.4 on MOT20, which shows that the proposed method is effective.
更多查看译文
关键词
tracking,flow,multi-object,two-stage,min-cost
AI 理解论文
溯源树
样例
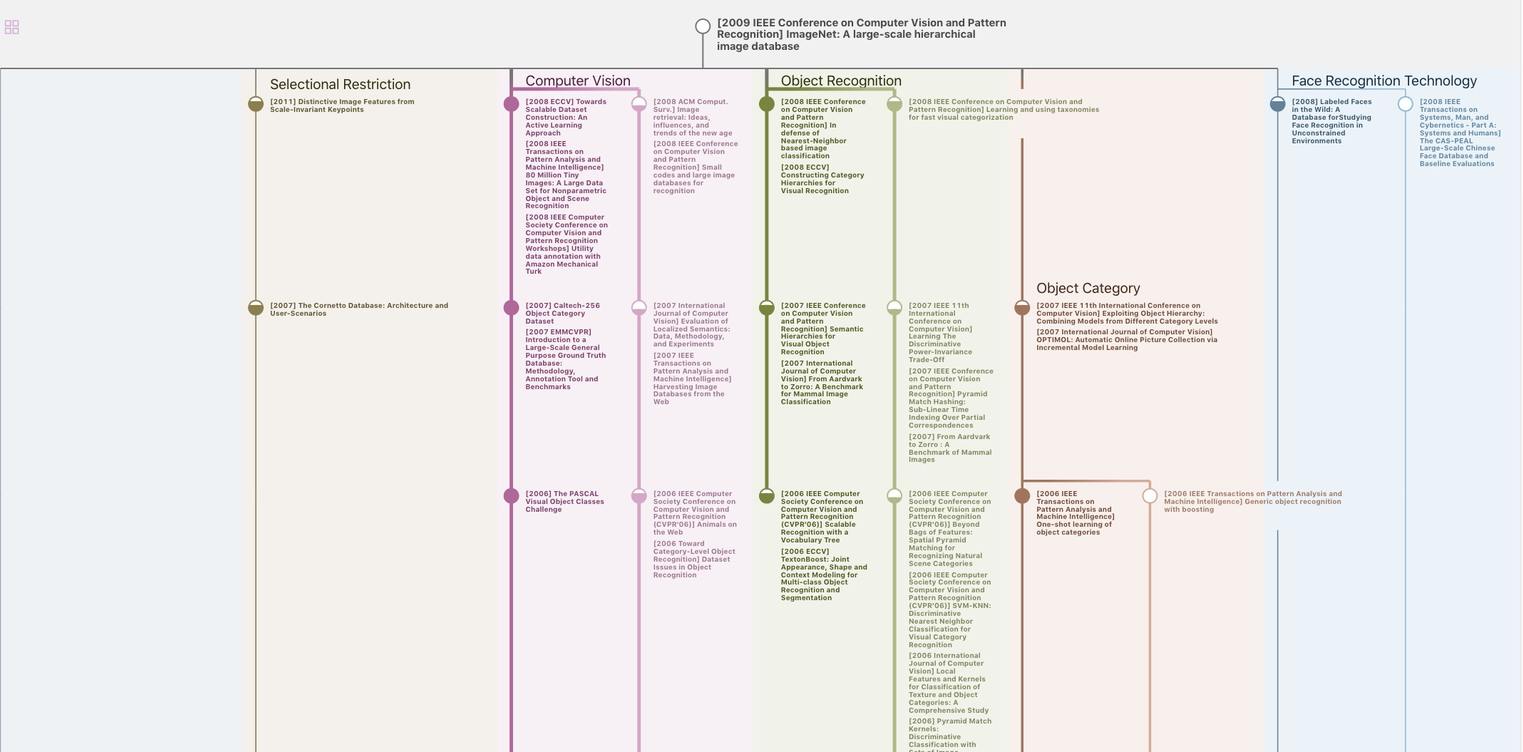
生成溯源树,研究论文发展脉络
Chat Paper
正在生成论文摘要