RVEAPE: An Approach to Computation Offloading for Connected Autonomous Vehicles
IEEE TRANSACTIONS ON AUTOMATION SCIENCE AND ENGINEERING(2023)
摘要
With the development of information technology, a variety of mobile devices with large computing requirements and delay constraints are growing rapidly, such as Connected Autonomous Vehicles (CAVs). In addition to the energy consumption required for vehicle driving, the CAV also needs to support the normal operation of sensors and computing platforms, which brings huge energy consumption and affects the endurance of vehicles. In this paper, a Mobile Edge Computing (MEC) system is considered that includes three types of network nodes: connected Autonomous Vehicles, Roadside Units (RSUs), and a Base Station (BS). Considering the task duration of the CAV, tasks on the CAVs are partitioned into three sections, which are executed at CAVs, RSUs, and BS, respectively. It is challenging to correlate resource-limited CAVs with other types of network nodes with high performance and implement partial computation offloading between them to minimize the total energy consumption of CAVs and RSUs under the delay constraint of CAVs. Based on the classical orthogonal-frequency-division multiple-access (OFDMA), a joint computing and communication cooperation offloading protocol is proposed to minimize the total energy consumption of all CAVs and RSUs under delay constraints and is described as a constrained Mixed-Integer Nonlinear Program (MINLP) problem. To address this problem, a Reference Vector-Guided Evolutionary Algorithm for Multi-Objective Optimization based on Prior Experience (RVEAPE) is designed in this paper. RVEAPE jointly optimizes the task division ratio variables and resource allocation variables (computation resources, communication resources). Simulations demonstrate that RVEAPE significantly outperforms the GA, NSGA-II, and NSGA-II algorithms in terms of the total energy consumption of CAVs and RSUs in the whole system consisting of CAVs, RSUs, and a BS. Note to Practitioners-This paper is motivated by the use of multi-objective optimization algorithms to improve the efficiency and quality of CAVs in edge computing networks. Our goal is to reduce energy consumption by offloading the computation tasks of the CAV to be computed in the RSU and BS. To this end, this paper proposes the use of the RVEAPE algorithm to jointly optimize offloading decisions and resource allocation variables to achieve the lowest energy consumption. The proposed method is theoretically effective in reducing the energy consumption of the CAV under the time constraint. Experimental studies show that the RVEAPE algorithm can effectively offload the computational tasks of the CAV to the BS, and can thus reduce the total energy consumption of the CAV and the RSU. In future research, we will implement computation offloading in large-scale CAV scenarios and consider a binary offloading model.
更多查看译文
关键词
Mobile edge computing,connected autonomous vehicle,joint computation and communication cooperation,computation offloading,resource allocation
AI 理解论文
溯源树
样例
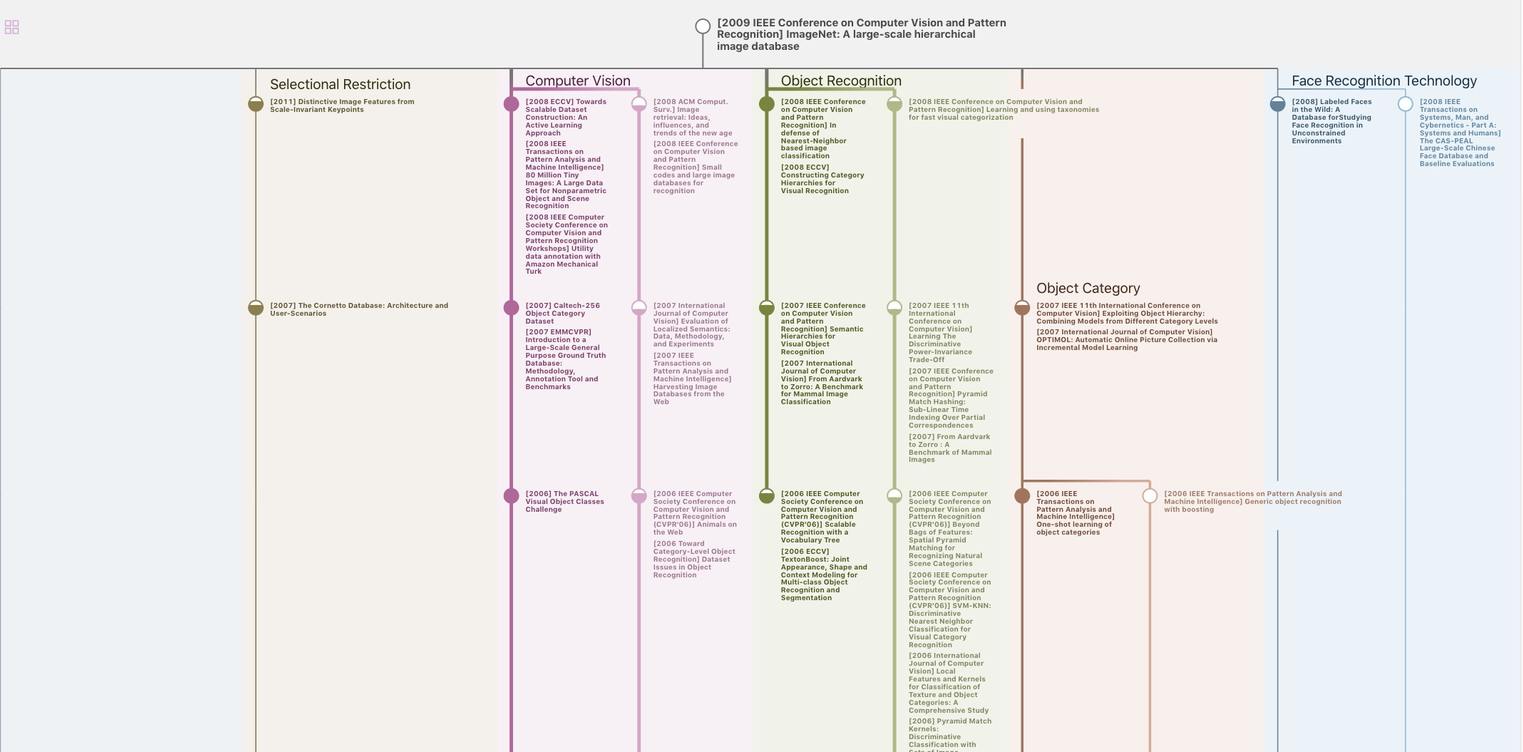
生成溯源树,研究论文发展脉络
Chat Paper
正在生成论文摘要