Real-time scheduling of renewable power systems through planning-based reinforcement learning
arXiv (Cornell University)(2023)
摘要
The growing renewable energy sources have posed significant challenges to traditional power scheduling. It is difficult for operators to obtain accurate day-ahead forecasts of renewable generation, thereby requiring the future scheduling system to make real-time scheduling decisions aligning with ultra-short-term forecasts. Restricted by the computation speed, traditional optimization-based methods can not solve this problem. Recent developments in reinforcement learning (RL) have demonstrated the potential to solve this challenge. However, the existing RL methods are inadequate in terms of constraint complexity, algorithm performance, and environment fidelity. We are the first to propose a systematic solution based on the state-of-the-art reinforcement learning algorithm and the real power grid environment. The proposed approach enables planning and finer time resolution adjustments of power generators, including unit commitment and economic dispatch, thus increasing the grid's ability to admit more renewable energy. The well-trained scheduling agent significantly reduces renewable curtailment and load shedding, which are issues arising from traditional scheduling's reliance on inaccurate day-ahead forecasts. High-frequency control decisions exploit the existing units' flexibility, reducing the power grid's dependence on hardware transformations and saving investment and operating costs, as demonstrated in experimental results. This research exhibits the potential of reinforcement learning in promoting low-carbon and intelligent power systems and represents a solid step toward sustainable electricity generation.
更多查看译文
关键词
renewable power systems,real-time,planning-based
AI 理解论文
溯源树
样例
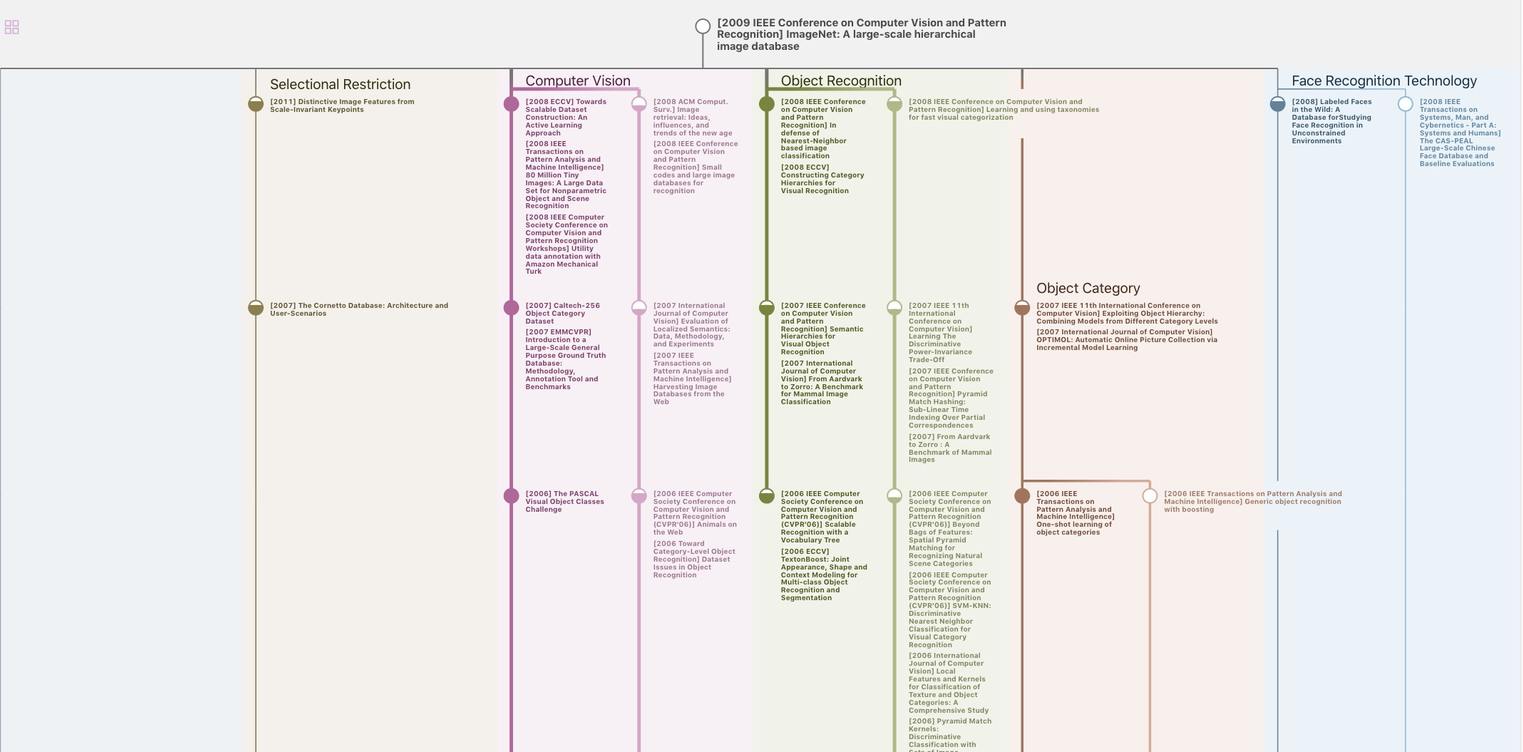
生成溯源树,研究论文发展脉络
Chat Paper
正在生成论文摘要