STD-NET: Search of Image Steganalytic Deep-Learning Architecture Via Hierarchical Tensor Decomposition
IEEE Transactions on Dependable and Secure Computing(2023)
摘要
Steganalysis aims to reveal covert communication established via steganography. In the arm race with steganography, steganalysis has evolved from the old-style hand-crafted features set to deep-learning architectures. However, recent studies show that the majority of existing deep steganalysis models have a large amount of redundancy, which leads to a huge waste of storage and computing resources. The existing model compression method cannot flexibly compress the convolutional layer in residual shortcut block so that a satisfactory shrinking rate cannot be obtained. In this paper, we propose STD-NET, an unsupervised deep-learning architecture search approach via hierarchical tensor decomposition for image steganalysis. Our proposed strategy will not be restricted by various residual connections, since this strategy does not change the number of input and output channels of the convolution block. We propose a normalized distortion threshold to evaluate the sensitivity of each involved convolutional layer of the base model to guide STD-NET to compress target network in an efficient and unsupervised approach, and obtain two network structures of different shapes with low computation cost and similar performance compared with the original one. Extensive experiments have confirmed that, on one hand, our model can achieve comparable or even better detection performance in various steganalytic scenarios due to the great adaptivity of the obtained network architecture. On the other hand, the experimental results also demonstrate that our proposed strategy is more efficient and can remove more redundancy compared with previous steganalytic network compression methods.
更多查看译文
关键词
deep-learning deep-learning,decomposition,std-net
AI 理解论文
溯源树
样例
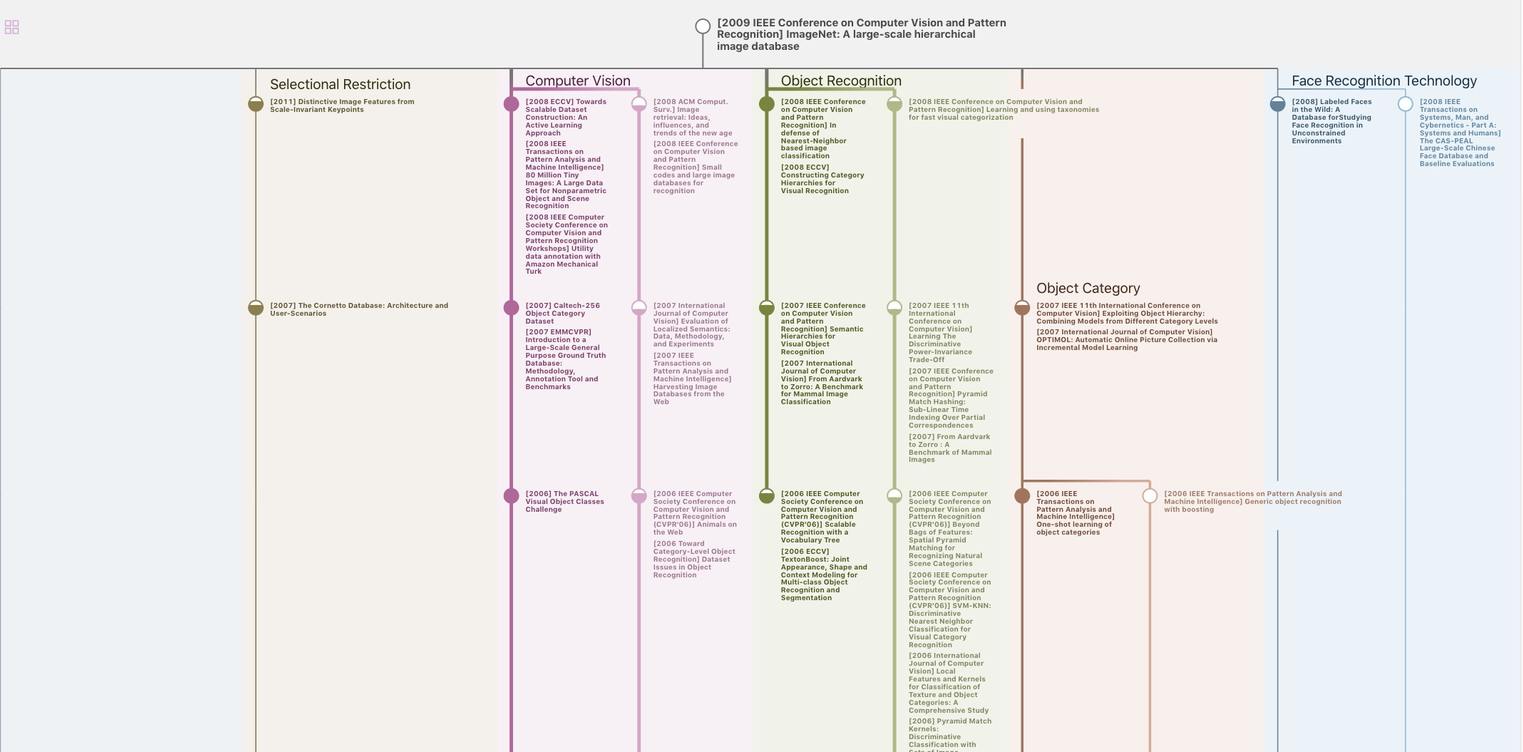
生成溯源树,研究论文发展脉络
Chat Paper
正在生成论文摘要