Backdoor Attacks in Peer-to-Peer Federated Learning
arXiv (Cornell University)(2023)
摘要
Most machine learning applications rely on centralized learning processes, opening up the risk of exposure of their training datasets. While federated learning (FL) mitigates to some extent these privacy risks, it relies on a trusted aggregation server for training a shared global model. Recently, new distributed learning architectures based on Peer-to-Peer Federated Learning (P2PFL) offer advantages in terms of both privacy and reliability. Still, their resilience to poisoning attacks during training has not been investigated. In this paper, we propose new backdoor attacks for P2PFL that leverage structural graph properties to select the malicious nodes, and achieve high attack success, while remaining stealthy. We evaluate our attacks under various realistic conditions, including multiple graph topologies, limited adversarial visibility of the network, and clients with non-IID data. Finally, we show the limitations of existing defenses adapted from FL and design a new defense that successfully mitigates the backdoor attacks, without an impact on model accuracy.
更多查看译文
关键词
backdoor attacks,learning,peer-to-peer
AI 理解论文
溯源树
样例
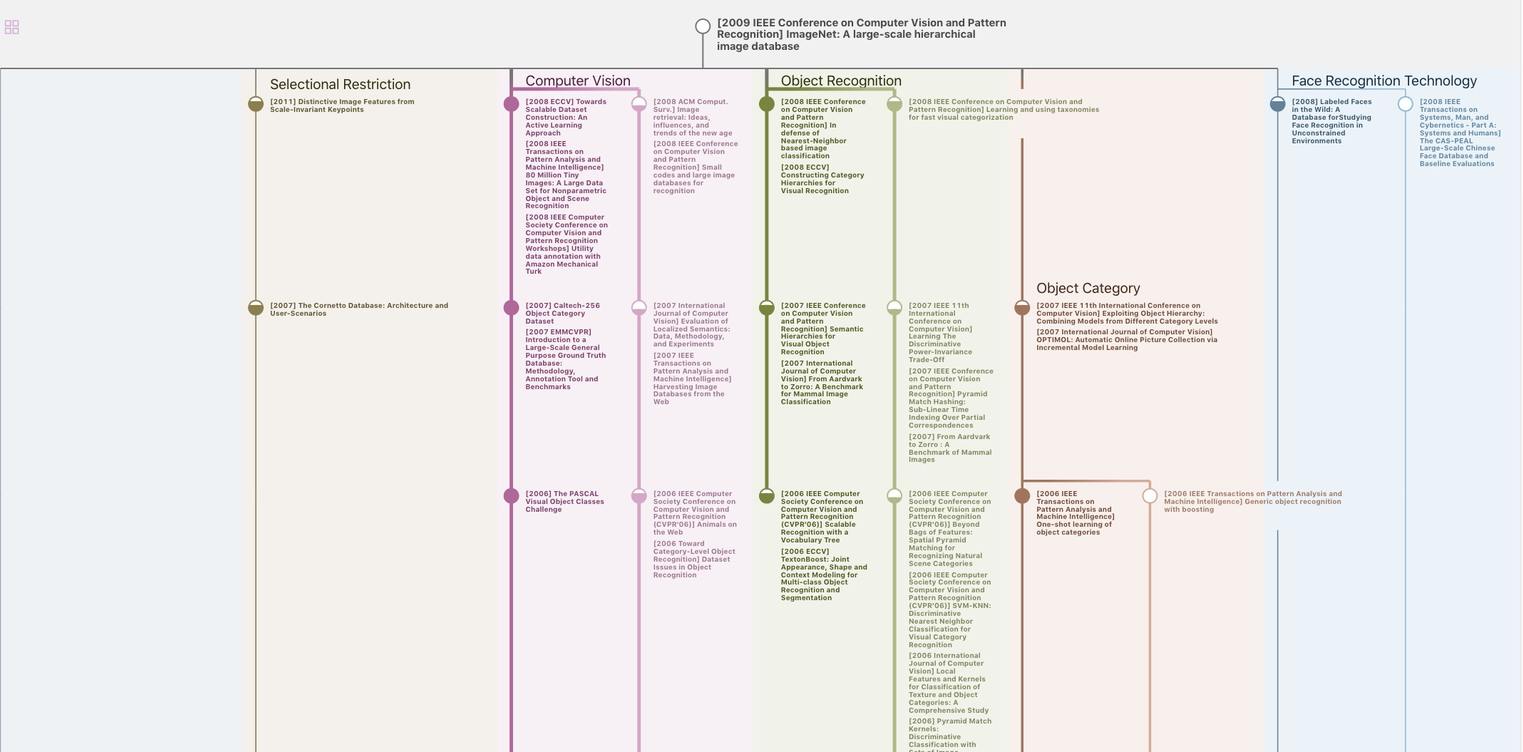
生成溯源树,研究论文发展脉络
Chat Paper
正在生成论文摘要