Explainable formation energy prediction for uncovering the relationship between the electronic structure and stability of the heterogeneous catalyst
Computer-aided chemical engineering(2023)
摘要
In recent studies, machine learning (ML) applications in the heterogeneous catalysis field for material properties prediction accelerate the catalyst discovery with desired properties. However, due to its high complexity, most ML models suffer from the black-box problem, which cannot provide a basis for prediction. Thus, reliable application and physical insight generation are challenging with conventional black-box models. Here, we developed an ML model that predicts formation energy (Ef) from the density of states (DOS). More importantly, by interpreting the model, we also confirmed the possibility of uncovering the relationship between the electronic structure of materials and their stability. Our model achieves successful performance demonstrating its superior capability of DOS featurization.
更多查看译文
关键词
explainable formation energy prediction,catalyst,electronic structure,stability
AI 理解论文
溯源树
样例
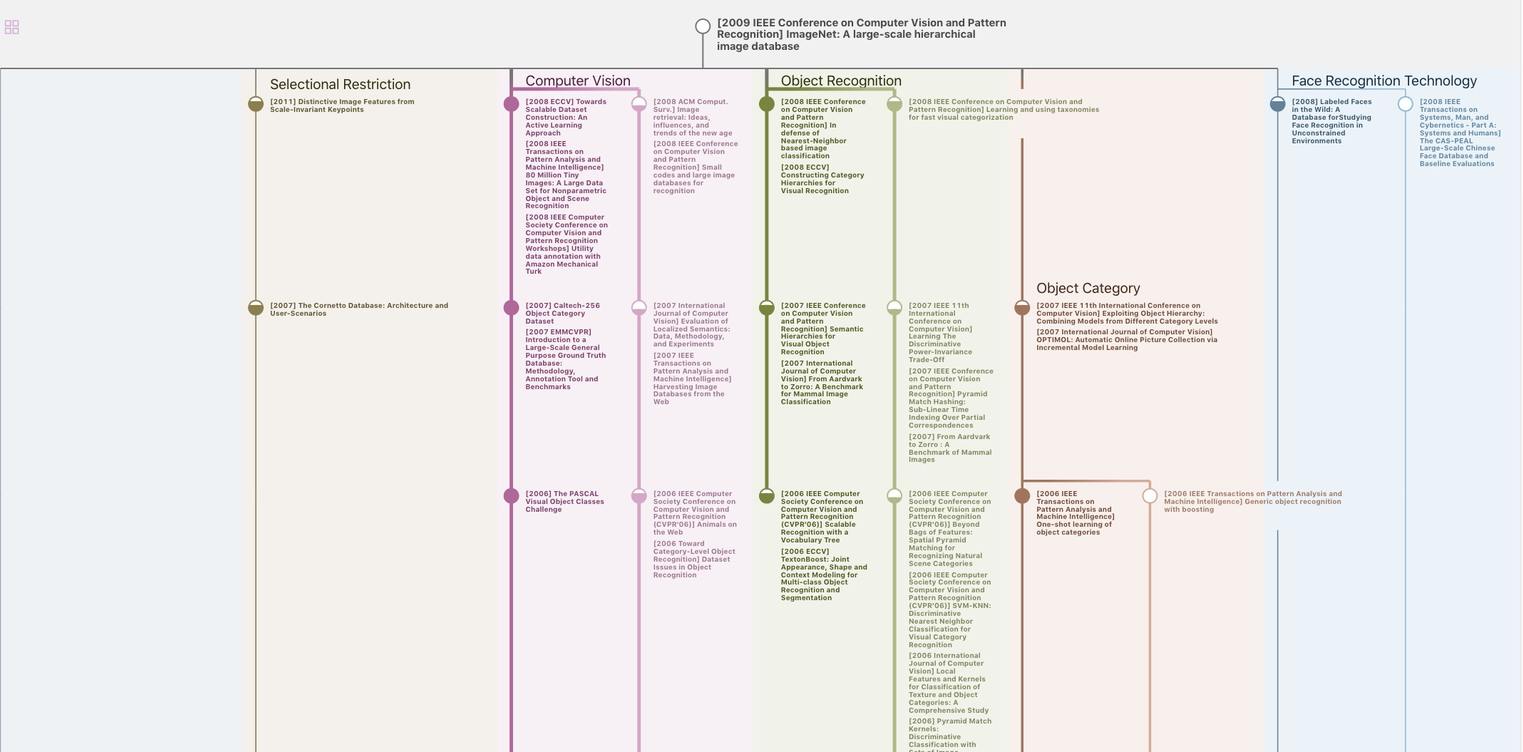
生成溯源树,研究论文发展脉络
Chat Paper
正在生成论文摘要