Duopoly Business Competition in Cross-Silo Federated Learning
IEEE TRANSACTIONS ON NETWORK SCIENCE AND ENGINEERING(2024)
摘要
In cross-silo federated learning, clients (e.g., organizations) collaboratively train a global model using private local data. In practice, clients may be not only collaborators but also business competitors. This article studies the overlooked but practically important problem of business competition in cross-silo FL. We formulate the clients' market competition as a three-stage game, where the clients decide FL training strategies in Stage I and the pricing strategies in Stage II, and then heterogeneous customers decide purchasing strategies in Stage III. The game analysis is highly challenging, as clients' collaborations and competitions are complexly coupled. We manage to characterize the equilibrium properties and find that market competition always reduces the clients' profits and can further lead to a worse global model when clients' costs are high. To mitigate this issue, we propose a general framework that enables proper revenue (profit plus cost) sharing among clients. Both theoretical and numerical results with MNIST and CIFAR-10 show that revenue sharing can greatly improve the global model accuracy and clients' profits. Counter-intuitively, even if market competition limits clients' profits, it can lead to a better global model when clients' costs are low, as clients strive to survive in the market by contributing more training data.
更多查看译文
关键词
Business,Training,Data models,Pricing,Collaboration,Numerical models,Costs,Federated learning,business competition,data sharing,game theory,machine learning
AI 理解论文
溯源树
样例
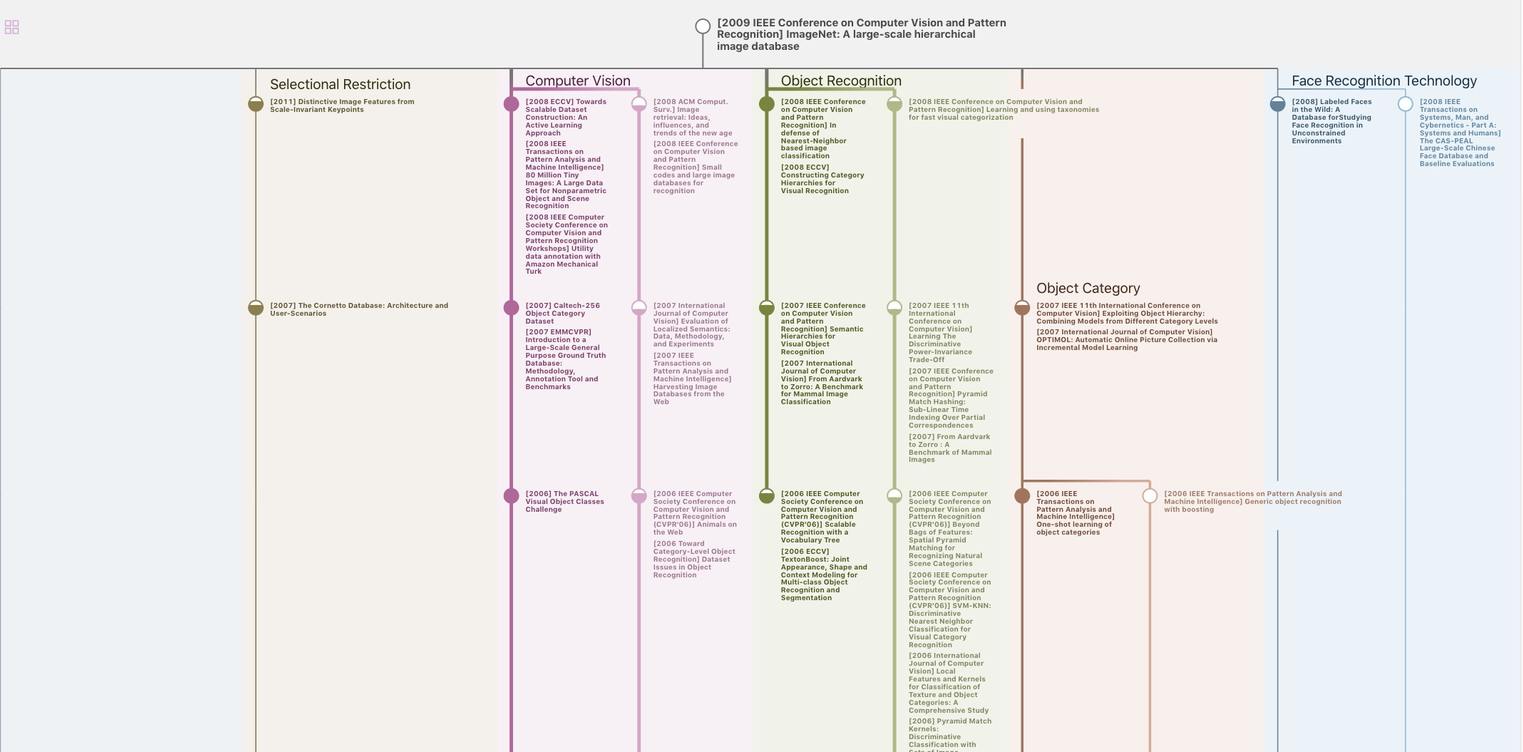
生成溯源树,研究论文发展脉络
Chat Paper
正在生成论文摘要