Pb2377: predicting cell of origin from digitized images of hematoxylin and eosin-stained slides of diffuse large b-cell lymphomas using a cell-based deep-learning model
HemaSphere(2023)
摘要
Topic: 20. Lymphoma Biology & Translational Research Background: Based on gene expression profiling, diffuse large B-cell lymphoma (DLBCL) can be classified by cell of origin (COO) as activated B-cell-like (ABC) or germinal center B-cell-like (GCB) tumors. These differ in prognosis, which means that timely and accurate classification is important to stratify patients and provide appropriate treatment. Determining COO using immunohistochemistry can be time-consuming and weakly reproducible, and poorly reflects underlying tumor biology. Aims: We developed a deep-learning (DL) model to perform COO classification using whole-slide images (WSIs) of hematoxylin and eosin (H&E)-stained pathology slides from patients with DLBCL. Methods: Algorithms were trained, validated and tested using data from the phase 2 CAVALLI (NCT02055820) and phase 3 GOYA (NCT01287741) trials. The slides from GOYA were split into a training set and a test set for model tuning, containing (ABC/GCB/total) 106/202/308 and 21/46/67 WSIs, respectively. The CAVALLI slides were used as an independent holdout set for further validation of the final model to prevent overfitting, and contained (ABC/GCB/total) 57/110/167 WSIs. For COO prediction, a transformer was used to aggregate extracted features and predict the WSI label in a weakly supervised manner. We compared the performance of two types of features as the input to the transformer: a tile-based model that extracted tumor regions from WSIs and a nucleus-based model that used automatically segmented nuclei from the same tiles. For each model, a fixed network pretrained with digital pathology images was used to extract features, which were used to make patch-level predictions that were aggregated at slide level. A model explainability method was applied, which assigned attention gradients to cells to gain insights into the cell-level patterns that weighted the ABC versus GCB classification. Results: Using the tile-based model, the areas under the receiver operating characteristic curves (AUCs) for the test set and holdout set were 0.73 and 0.67, and the average F1 scores were 0.68 and 0.61 respectively; the nucleus-based model had AUCs of 0.63 and 0.70, and average F1 scores of 0.61 and 0.64 using the same test settings. Visual inspection of the highest-scoring tiles and nucleus patches revealed a higher density of tumor cells and larger overall cell size for ABC than for GCB DLBCL. Similarly, the ratio of tumor cells to nontumor cells, and the average tumor cell size, were significantly larger for ground truth ABC images than GCB images (p < 0.001 for all data sets). In addition, on pathologist inspection of the nucleus images and explainability model attention gradients, more ABC predictions were related to bright areas of nuclei with lower chromatin content than for GCB predictions. Summary/Conclusion: Our DL models demonstrated reasonable performance in COO classification of DLBCL using WSIs of H&E-stained slides without use of ancillary techniques. In addition, our models may be able to identify novel cellular features that differentiate ABC from GCB DLBCL. With rigorous validation and regulatory approval, the models may have the potential to supplement the pathologist’s diagnostic tool kit with a reliable method of COO classification. Keywords: Diffuse large cell lymphoma, Artificial intelligence, Lymphoma, Prognostic groups
更多查看译文
关键词
deep-learning deep-learning model,deep-learning deep-learning,eosin-stained,b-cell,cell-based
AI 理解论文
溯源树
样例
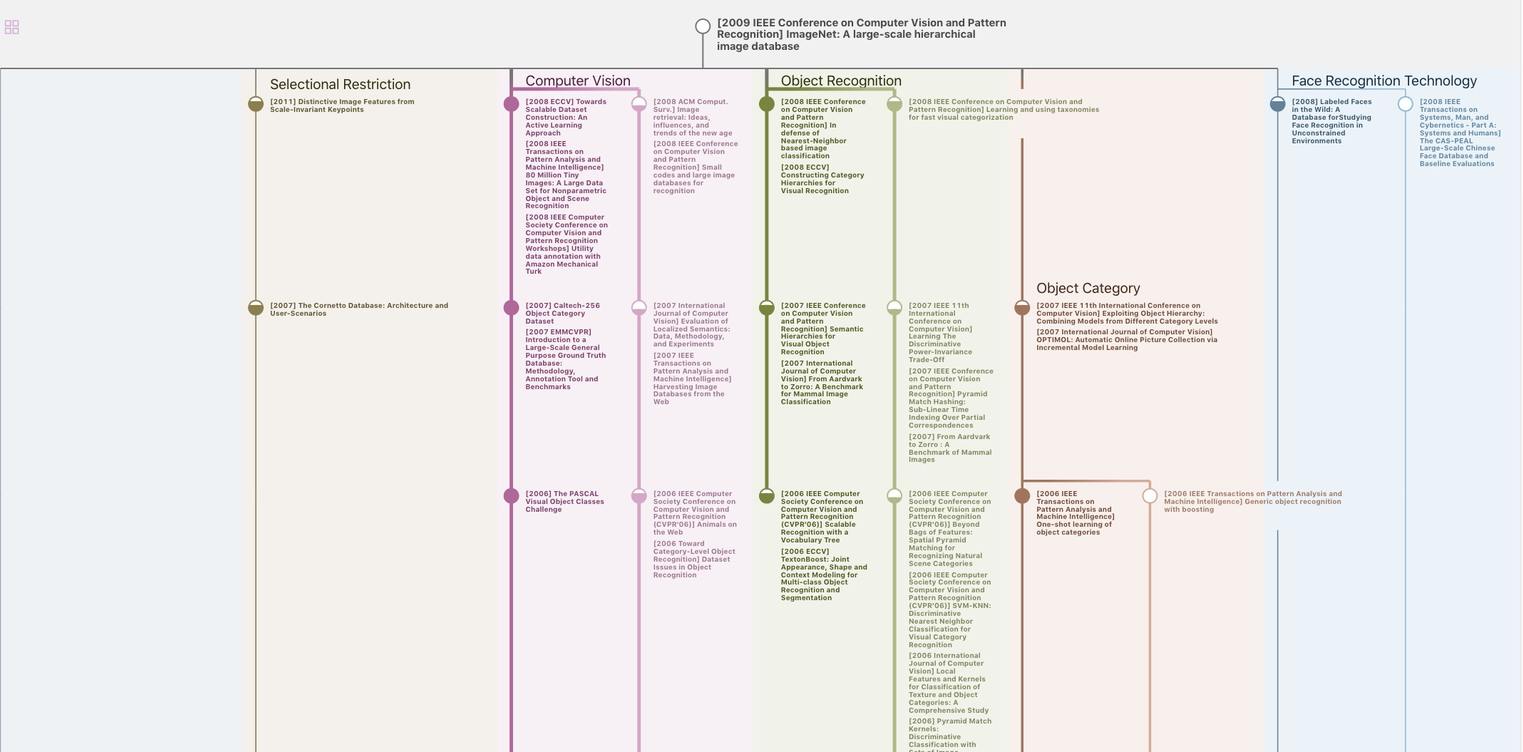
生成溯源树,研究论文发展脉络
Chat Paper
正在生成论文摘要