Radio environment maps for indoor visible light communications aided by machine learning
AEU-INTERNATIONAL JOURNAL OF ELECTRONICS AND COMMUNICATIONS(2023)
摘要
VLC has been proposed as reliable high-capacity wireless optical communication system for indoor access networks. This technology comprises a high bandwidth unlicensed solution and has been suggested for ultra-reliable environments like hospitals. In this study, Machine Learning is used to investigate indoor wireless optical channels where ultra-reliable communications and signal coverage are required. Specifically, we assess the precision of different Decision Trees ML algorithms based on their mean absolute error metric in comparison to the anticipated simulated outcomes for optical channel estimation in various rooms that could be used in medical care. We demonstrate that Decision Trees models are capable of making fast and accurate predictions. It is shown that the Stack model is the most appropriate Decision Trees-based method, from the ones we studied, for predicting indoor VLC RSS values and constructing the corresponding REMs. These models are 300-65,000 times faster than traditional calculation methods, depending on the number of reflections bounces considered, and deliver accurate predictions, with less than 0.015 mean absolute percentage error, of the Radio Environment Map of a communication system that supports VLC. This is the first implementation of constructing optical REMs for Indoor VLC systems to the best knowledge of the authors.
更多查看译文
关键词
Visible Light Communications (VLC),Optical wireless channel modelling,Machine Learning (ML)
AI 理解论文
溯源树
样例
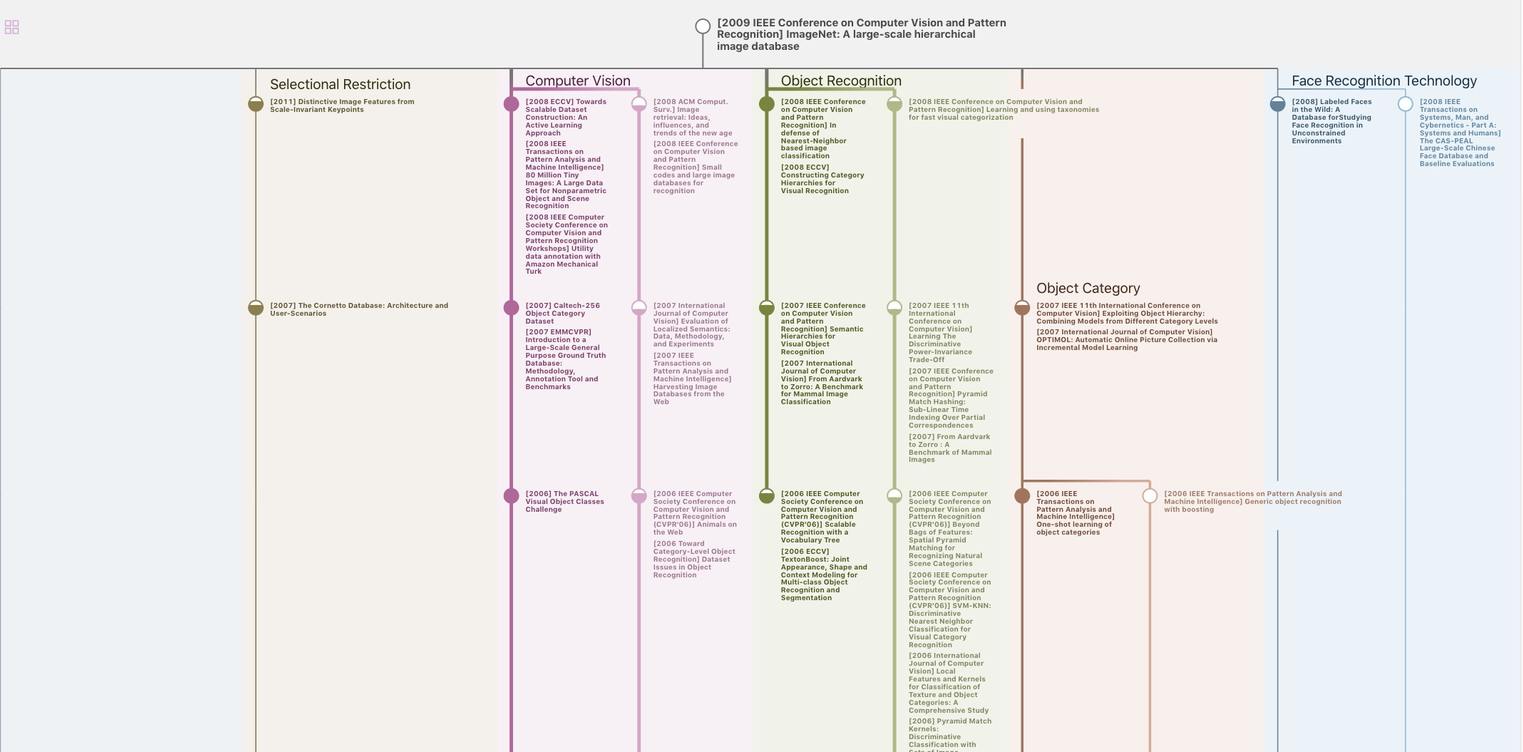
生成溯源树,研究论文发展脉络
Chat Paper
正在生成论文摘要