Measuring employer attractiveness in diverse talent markets
DECISION SUPPORT SYSTEMS(2024)
摘要
Previous work has measured employer attractiveness via proxies, such as the number of new hires or employee sentiment mined from surveys and reviews. The first drawback of such metrics is that they treat all talent as one homogeneous population with uniform recruitability patterns. Second, they ignore that a firm's attractiveness is heavily influenced by that of its competitors, as they all vie for the same talent. Third, they assume attractiveness remains constant across time. We address all three drawbacks via a methodology that can cluster job titles to identify prevalent talent types, as well as compute and monitor employer attractiveness for each type in a competitive market. Our clustering approach groups job titles based on their lexical similarity, their cooccurrence in professional resumes, and their overlap in terms of the skills that they require. Our work directly informs the decisions of employers trying to recruit in competitive talent markets, as well as of workers trying to identify the best destination for their career. We compare our approach to competitive baselines and demonstrate how it can improve the results of key applications.
更多查看译文
关键词
Employer attractiveness,Job title clustering,Workforce analysis
AI 理解论文
溯源树
样例
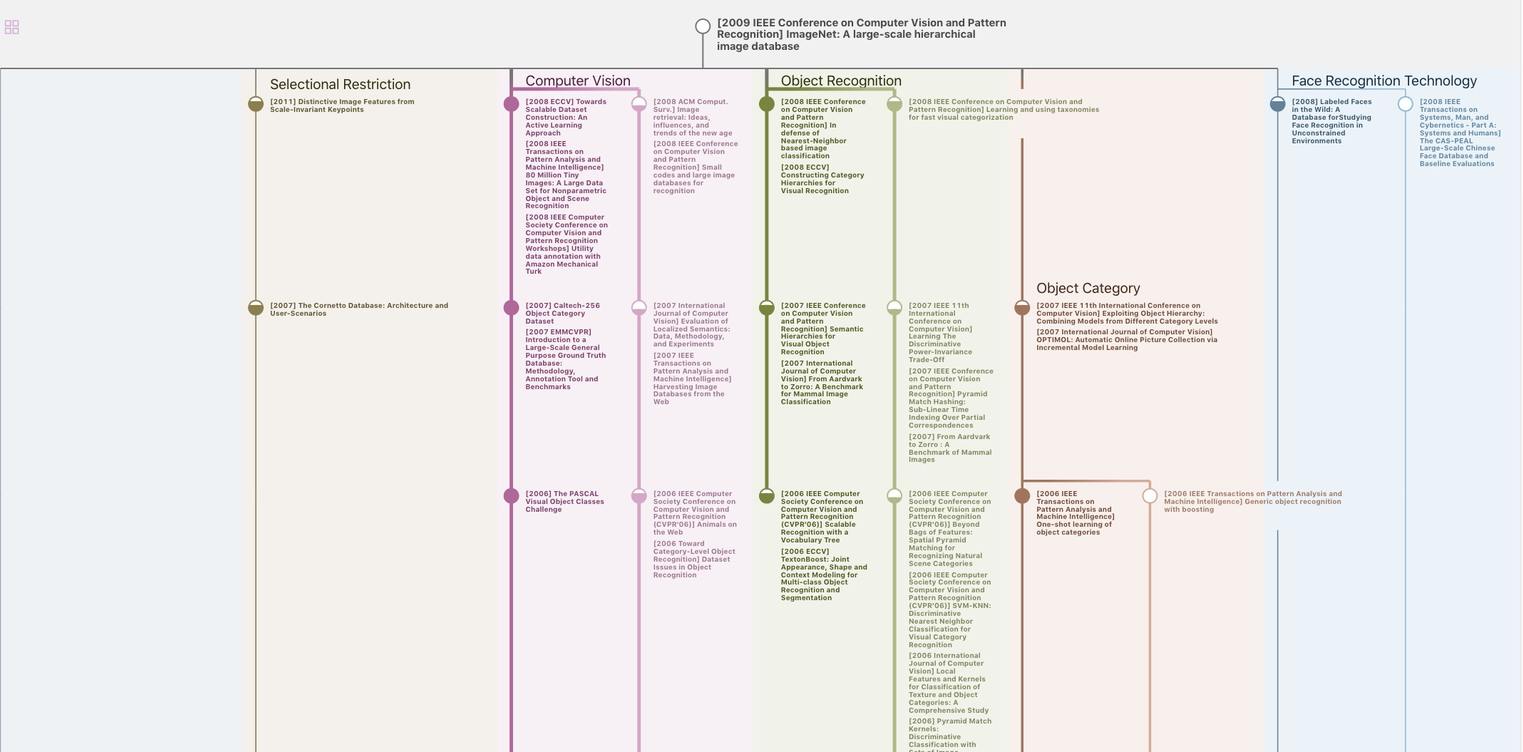
生成溯源树,研究论文发展脉络
Chat Paper
正在生成论文摘要