Distributed Resource Allocation and User Association for Max-Min Fairness in HetNets
IEEE TRANSACTIONS ON VEHICULAR TECHNOLOGY(2024)
摘要
In heterogeneous networks (HetNets), it is difficult to guarantee uniform wireless connectivity satisfying quality of service (QoS) on users' minimum rates because of intensive and capricious inter-cell interference. Therefore, user association (UA) and resource allocation (RA) should be carefully designed at all interfering base stations (BSs) to enhance the achievable QoS on users' minimum rates. However, existing UA frameworks mainly focus on improving sum-rate and proportional fairness, which often results in frequent failure to guarantee minimum rate QoS. In addition, most of them require global channel state information (CSI) or time-consuming iterative optimization. Hence, existing UA approaches cannot be applied under practical conditions such as fast time-varying channels and highly limited information exchange among the BSs. To cope with these practical conditions, we propose a deep-reinforcement-learning (DRL)-based joint UA and RA scheme to maximize the minimum rate, i.e., max-min fairness (MMF), with highly limited information exchange among the BSs. The proposed DRL algorithm optimizes UA at each BS in a distributed manner to maximize an MMF objective function with only local CSI and without any iterative process. Numerical results show that the proposed scheme outperforms the existing schemes and CSI-exchange-based schemes under various scenarios with fast time-varying channels and highly limited information exchange among the BSs.
更多查看译文
关键词
Deep reinforcement learning,limited information exchange,max-min fairness,resource allocation,user association
AI 理解论文
溯源树
样例
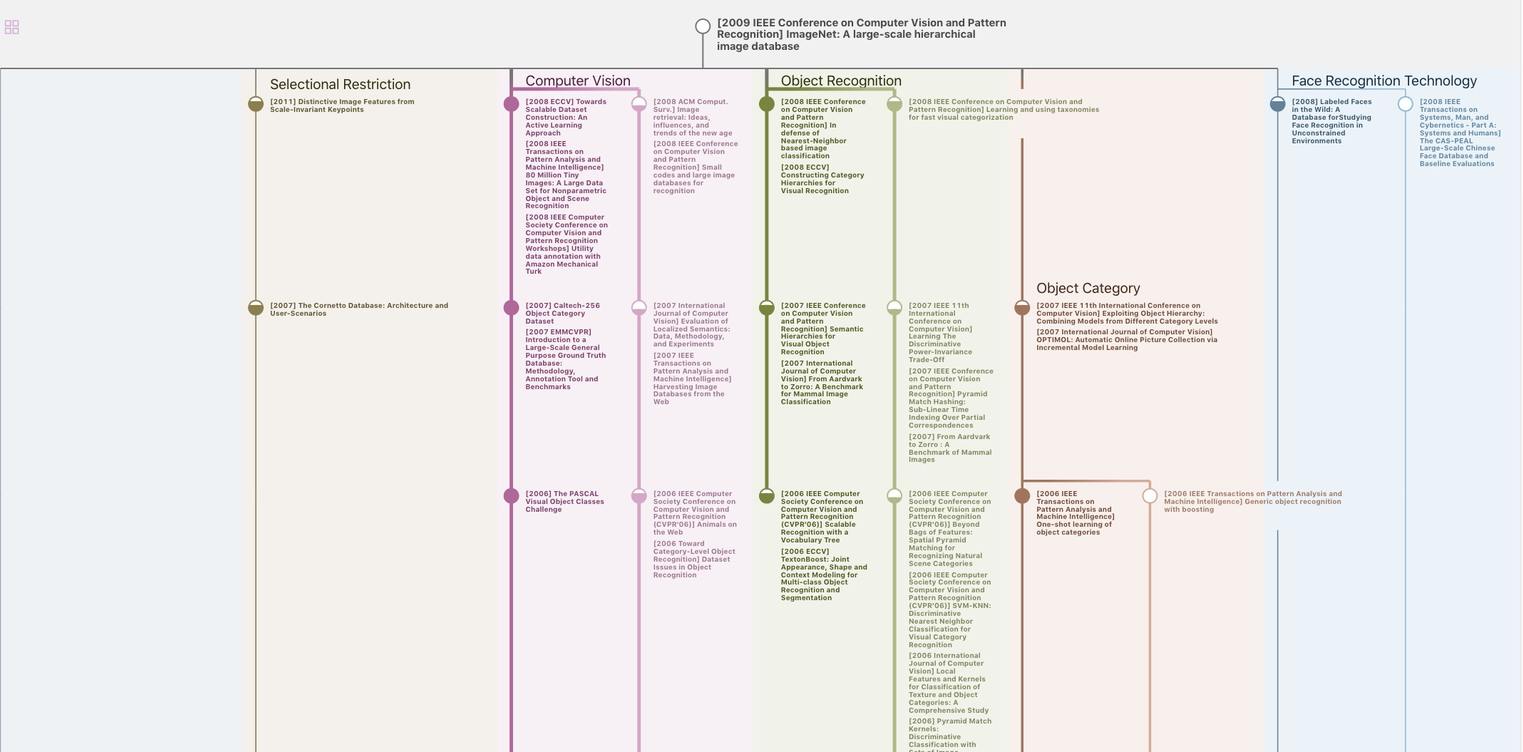
生成溯源树,研究论文发展脉络
Chat Paper
正在生成论文摘要