Data representation: from multiscale transforms to neural networks
Elsevier eBooks(2024)
摘要
Transients in 1D/2D signals often carry critical information, and hence constitute useful features for analysis and inference. A 2D signal, for example, is visually represented as an image and typically contains spatially distributed geometrical shapes that can be characterized by edges that signal the transients from one object to other objects. The edges are the discontinuities in an image intensity function along curves, contours, or lines and can be more closely studied by zooming in to the most important isotropic or anisotropic features in an image. This is the multiscale analysis which is the primary focus of this chapter. Additionally, it covers some recent developments in integrating wavelet analysis with neural networks for a joint exploitation of their respective advantages in statistical inference applications. Aimed as a tutorial, this chapter provides a basic introduction, with some perspective and a somewhat high-level exposition of the theoretical frameworks of classical wavelets and anisotropic wavelets, as well as a discussion on the integration of wavelets into neural networks. Our main goal is to provide working knowledge of these tools, focusing on their discrete scenarios as well as their potential for applications in information sciences and image processing in general, rather than the deep theoretical aspects which may be found in the extensive bibliography.
更多查看译文
关键词
multiscale transforms,neural networks,representation,data
AI 理解论文
溯源树
样例
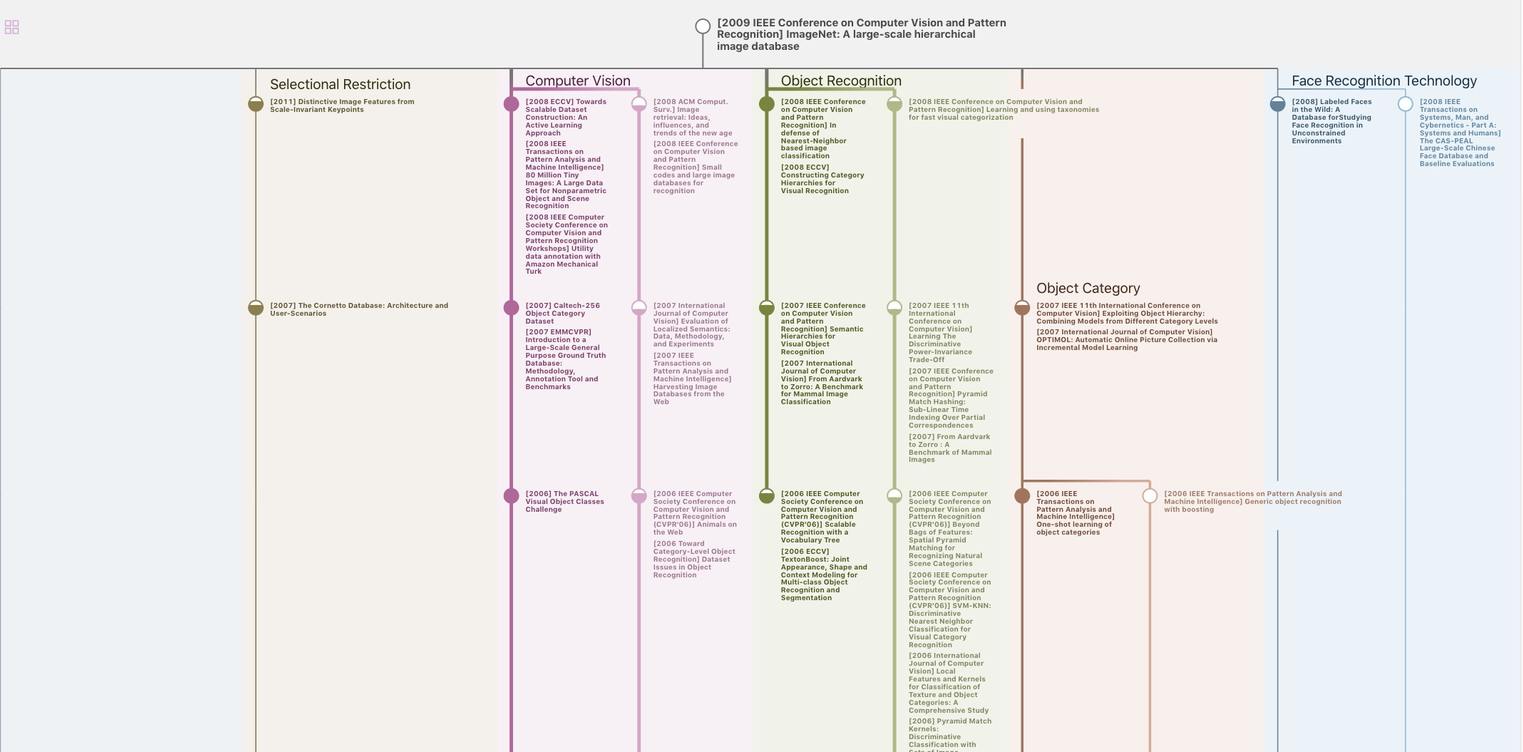
生成溯源树,研究论文发展脉络
Chat Paper
正在生成论文摘要