Economic-threshold-based classification of soybean aphid, Aphis glycines, infestations in commercial soybean fields using Sentinel-2 satellite data
CROP PROTECTION(2024)
摘要
The soybean aphid (SBA), Aphis glycines Matsumura (Hemiptera: Aphididae), is a significant insect pest of soybean, Glycine max (L.) Merrill (Fabales: Fabaceae), and field treatment decisions for this pest are based on average field populations. Previous studies indicated that ground- and drone-based red-edge and near-infrared remote sensing can be used to detect plant stress caused by SBA infestations in soybean. However, it remains to be determined if remote sensing for SBA can be expanded to field or landscape scale using satellite-based platforms. Thus, this research was conducted in three steps to determine the potential of using Sentinel-2 satellite data for the classification of SBA infestations in soybean fields using simulated and actual Sentinel-2 satellite spectral reflectance. In the first step, as a proof of concept, hyperspectral data from cage studies were used to simulate Sentinel-2 bands and vegetation indices (VIs), conducted in nine trials at multiple locations between 2013 and 2021. The effects of SBA from caged plants on simulated data were evaluated with random intercept linear mixed models. The satellite simulation indicated a significant effect of SBA on the spectral reflectance of caged soybean plants (p < 0.05) for four satellite bands (5, 6, 7, and 8A) and five VIs (NDVI, GNDVI, SAVI, OSAVI, and NDRE). In the second step, actual Sentinel-2 spectral reflectance and corresponding aphid counts of commercial soybean fields, collected from 2017 to 2019, were obtained. The relationship between SBA counts and Sentinel-2 spectral reflectance from commercial soybean fields were evaluated with general linear models. A significant effect of SBA was observed for three satellite bands (6, 7, and 8A) and three VIs (NDVI, SAVI, and OSAVI). In the third step, linear support vector machine (LSVM) models for the classification of SBA infestations as above or below a previously determined economic threshold of 250 aphids per plant were developed using simulated Sentinel-2 bands and VIs from the caged plots, and were tested on actual Sentinel-2 data from commercial soybean fields. The best LSVM model for the classification of aphids in soybean reached 91% accuracy, 85.7% sensitivity, and 93.3% specificity. Thus, simulations with caged plots can be used as an indication of the potential of using satellite data for the detection of plant stresses on a larger scale. Furthermore, this study advances decision-making for SBA, and the developed LSVM model can be used to update regional and local monitoring for the management of SBA.
更多查看译文
关键词
Machine learning,Linear support vector machine,Simulation,Soybean spectral response
AI 理解论文
溯源树
样例
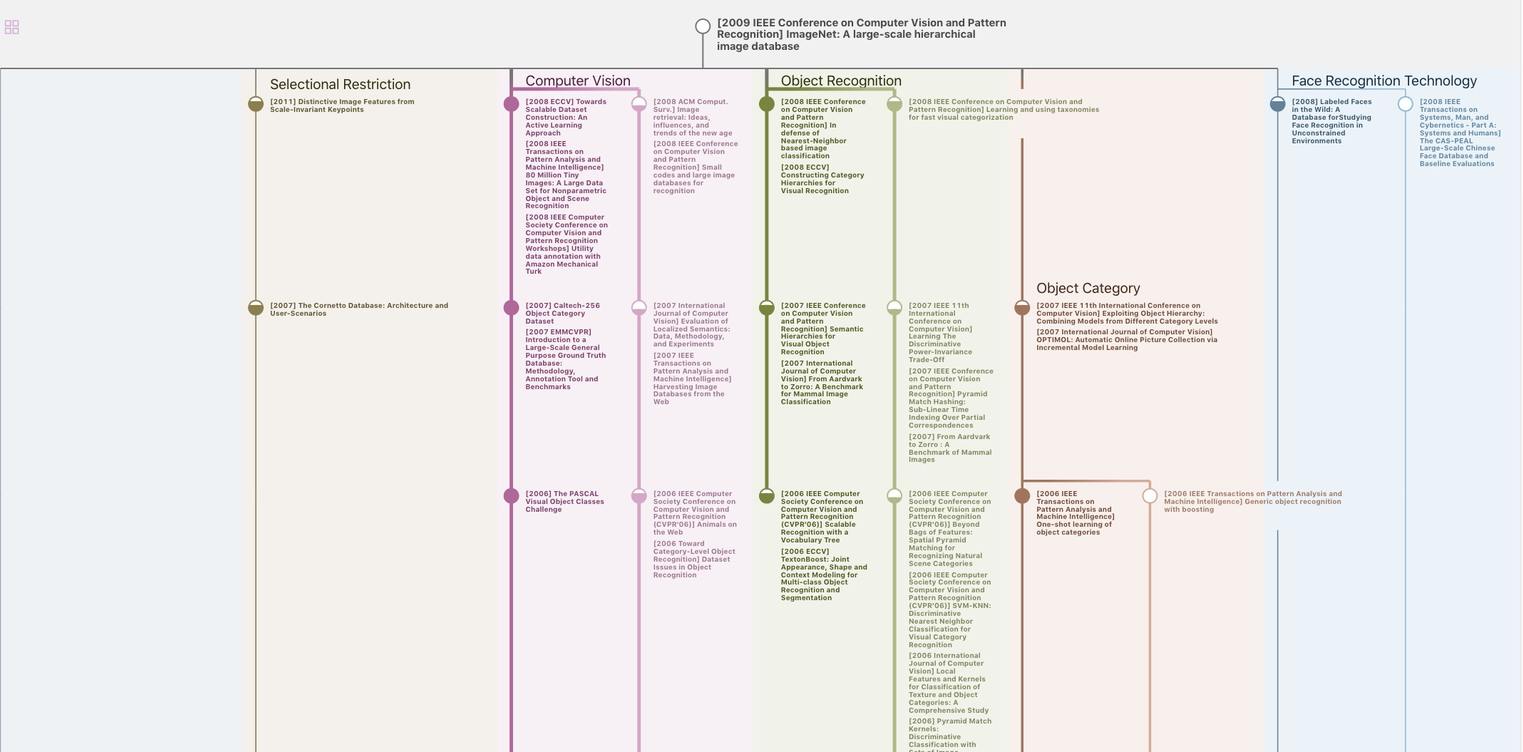
生成溯源树,研究论文发展脉络
Chat Paper
正在生成论文摘要