Data augmentation using virtual word insertion techniques in text classification tasks
EXPERT SYSTEMS(2024)
摘要
Labelling multiple training examples for text classification models is usually time-consuming and complex. Data augmentation can be used to automatically expand the dataset by transforming the original data. However, it may cause semantic changes without modifying the labels, which reduces the effectiveness of the classifiers. In this paper, we propose a data-augmentation method called the virtual word insertion technique, which generates new sentences by randomly inserting virtual words into existing sentences. Two methods are used to achieve virtual word embedding: unweighted average and weighted average. Furthermore, a new concept of weight is proposed: the class deviation factor, which demonstrates the correlation between words and classes. Based on this new concept, different weights are assigned to words of different classes. Experiments are conducted on five different classification tasks. Ablation experiments are also performed to explore the effects of random operation and number of augmented sentences for classification results. The results of these experiments show that our method improves the classification performance and outperforms two other contrasting data-augmentation methods in automatically augmenting the dataset. Compared to raw datasets, the average accuracy improvement of our method is 3.5% for a small-scale dataset and 1% for a large-scale dataset.
更多查看译文
关键词
class deviation factor,data augmentation,text classification,virtual word insertion techniques
AI 理解论文
溯源树
样例
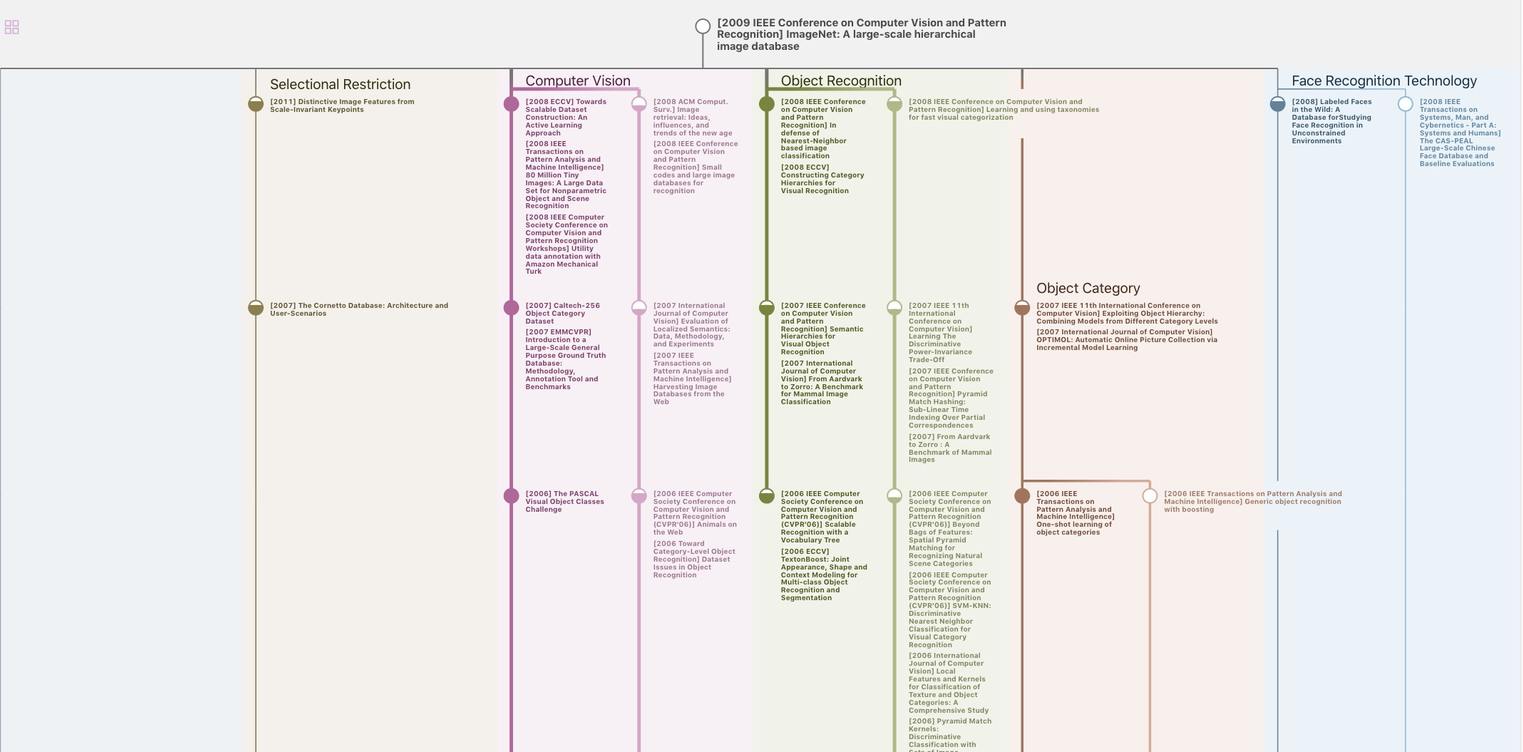
生成溯源树,研究论文发展脉络
Chat Paper
正在生成论文摘要