Low-Complexity Variational Bayesian Inference Based Groupwise Detection for Massive MIMO Uplinks
IEEE TRANSACTIONS ON WIRELESS COMMUNICATIONS(2023)
摘要
This paper proposes a low-complexity groupwise detection scheme for massive multiple-input multiple-output (MIMO) systems based on two key features of massive MIMO channels. First, most inter-user correlations tend to be negligible as the number of antennas M at the base station (BS) increases, while some users might have a high correlation that is indistinguishable for linear projection-based detectors. We hence develop a variational Bayesian inference-based groupwise (VBI-G) detector based on a factorization approximation of the a posteriori probability of transmitted symbols, which sequentially performs nonlinear detection for small groups of highly correlated users without computations of large-scale matrices. Second, the variation of normalized inter-user correlation is insignificant over a wide range of frequency, thereby user grouping based on correlation coefficients can be performed on a wideband basis. Accordingly, we propose a low-complexity incremental greedy grouping algorithm for minimizing the Kullback-Leibler divergence of the unconditioned posterior probabilities with the constraint of a maximum group size. Theoretical analysis proves that for a BS equipped with a uniform linear array, the maximum group size grows logarithmically with the number of users K with high probability in the regime M, K -> infinity with K/M < 1/2 under the worst line-of-sight propagation condition. Simulation results verify that the proposed VBI-G detector achieves superior performance with an extremely low computational overhead for massive MIMO systems.
更多查看译文
关键词
Detectors,Massive MIMO,Correlation,Symbols,Uplink,Interference cancellation,Bayes methods,groupwise detection,variational Bayesian inference,computational efficiency
AI 理解论文
溯源树
样例
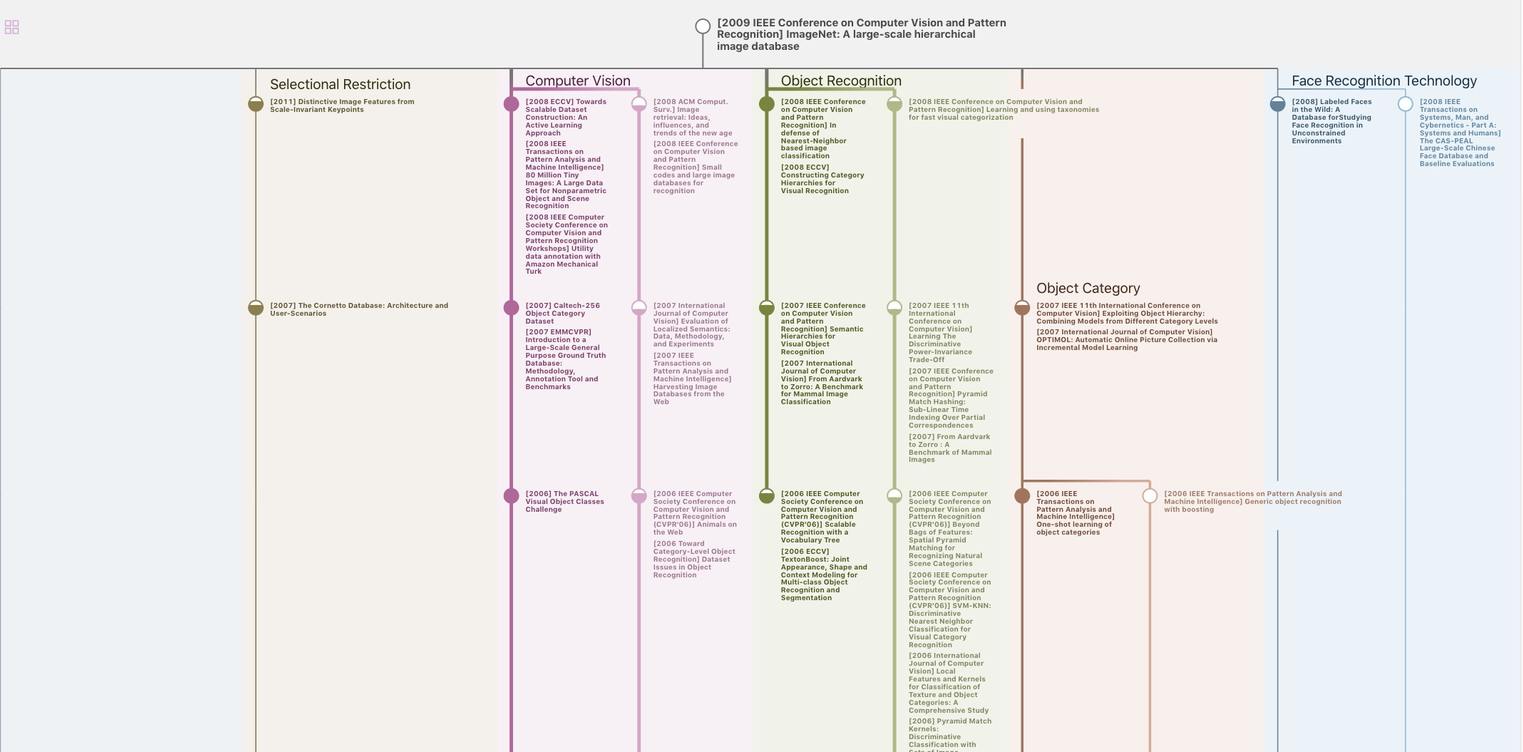
生成溯源树,研究论文发展脉络
Chat Paper
正在生成论文摘要