Fast convergence of sample-average approximation for saddle-point problems
arxiv(2023)
摘要
Stochastic saddle point (SSP) problems are, in general, less studied compared
to stochastic minimization problems. However, SSP problems emerge from machine
learning (adversarial training, e.g., GAN, AUC maximization), statistics
(robust estimation), and game theory (stochastic matrix games). Notwithstanding
the existing results on the convergence of stochastic gradient algorithms,
there is little analysis on the generalization, i.e., how learned on train data
models behave with new data samples. In contrast to existing
$\mathcal{O}(\frac{1}{n})$ in expectation result arXiv:2006.02067,
arXiv:2010.12561 and $\mathcal{O}(\frac{\log(\frac{1}{\delta})}{\sqrt{n}})$
results with high probability arXiv:2105.03793 , we present
$\mathcal{O}(\frac{\log(\frac{1}{\delta})}{n})$ result in high probability for
strongly convex-strongly concave setting. The main idea is local norms
analysis. We illustrate our results in a matrix-game problem.
更多查看译文
AI 理解论文
溯源树
样例
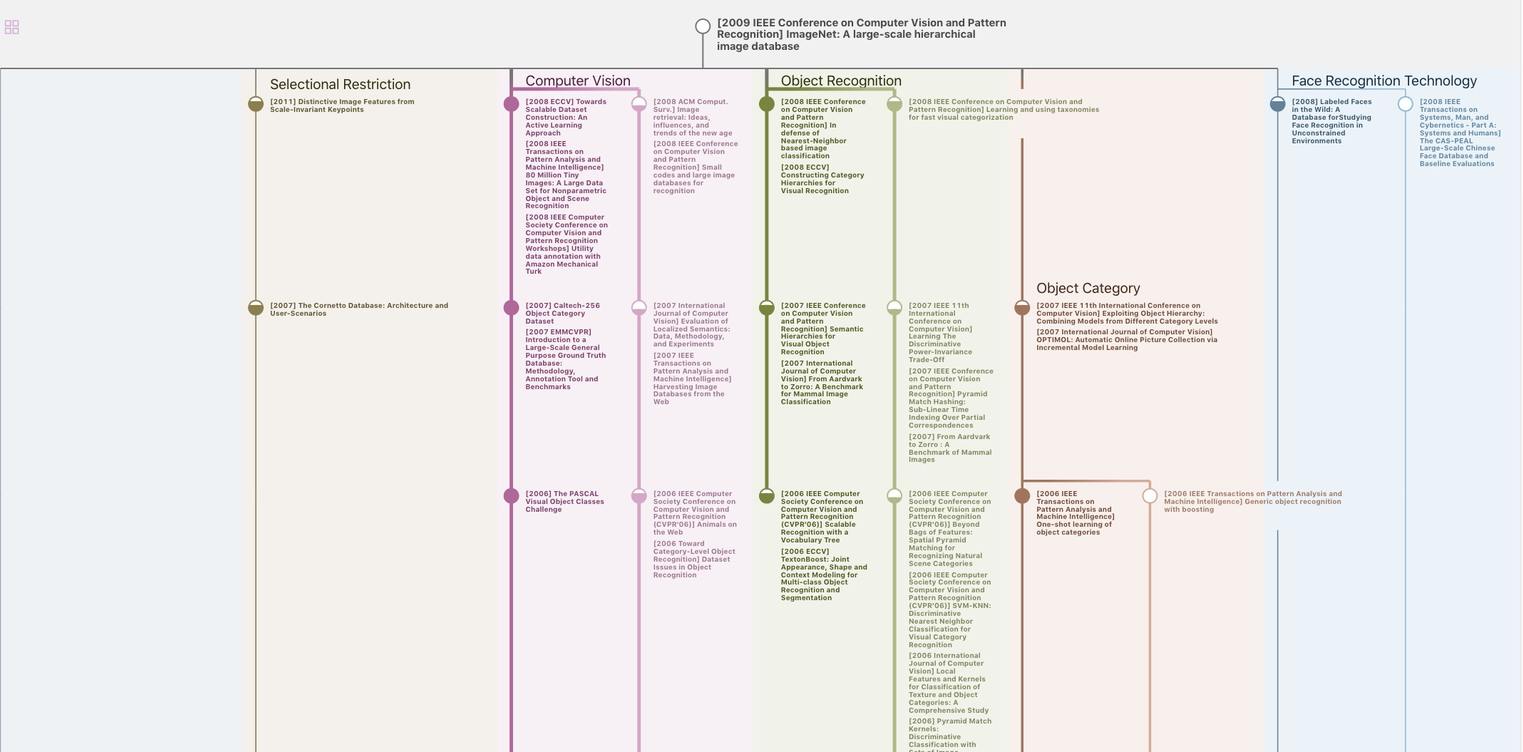
生成溯源树,研究论文发展脉络
Chat Paper
正在生成论文摘要