Image Enhancement for Unconstrained Environments
2023 IEEE Western New York Image and Signal Processing Workshop (WNYISPW)(2023)
摘要
De-noising and exposure correction are both well-studied in the field of computer vision. Prior research has handled both problems separately. The ultimate goal of our work is to combine these into a single problem as most realistic low-light images inherently come with noise. Tailor-made solutions like custom de-noise filters were used for image enhancement but recent applications have replaced that with DNN due to their ability to generalize in different noise and exposure scenarios. But these models need a sheer amount of realistic data for training, which is an issue as it requires human labor and it’s infeasible to capture images that model all types of irregularities. In this research, we present a novel use of DNN for processing low-light images. It involves de-noising and exposure correction which greatly improves the usability of images. We explore ways to generate realistic low-light images that are noisy while considering both denoising and exposure-correction factors. These images can be used to train any suitable DNN model. The data generation process requires a detailed understanding of the noise architecture with a digital camera’s image acquisition process. Experimental results conclude that irrespective of the DNN model being used the performance gets better when trained on our synthetic noisy dataset.
更多查看译文
关键词
image denoising,Super Resolution GAN,image enhancement,biometrics,face recognition
AI 理解论文
溯源树
样例
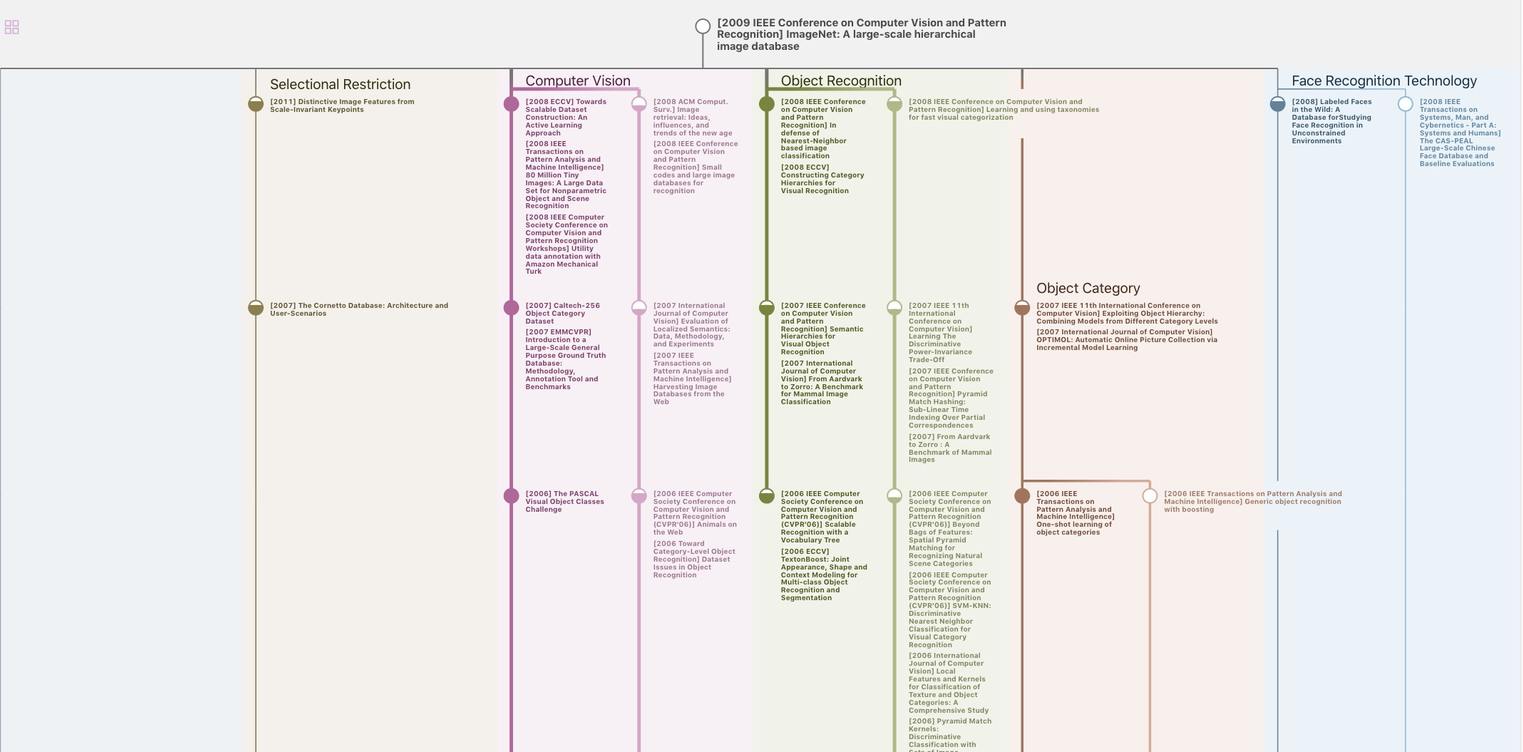
生成溯源树,研究论文发展脉络
Chat Paper
正在生成论文摘要