Self-Supervised Drivable Area Segmentation Using LiDAR's Depth Information for Autonomous Driving
2023 IEEE/RSJ INTERNATIONAL CONFERENCE ON INTELLIGENT ROBOTS AND SYSTEMS, IROS(2023)
摘要
Drivable area segmentation is an essential component of the visual perception system for autonomous driving vehicles. Recent efforts in deep neural networks have significantly improved semantic segmentation performance for autonomous driving. However, most DNN-based methods need a large amount of data to train the models, and collecting large-scale datasets with manually labeled ground truth is costly, tedious, time consuming and requires the availability of experts, making DNN-based methods often difficult to implement in real world applications. Hence, in this paper, we introduce a novel module named automatic data labeler (ADL), which leverages a deterministic LiDAR-based method for ground plane segmentation and road boundary detection to create large datasets suitable for training DNNs. Furthermore, since the data generated by our ADL module is not as accurate as the manually annotated data, we introduce uncertainty estimation to compensate for the gap between the human labeler and our ADL. Finally, we train the semantic segmentation neural networks using our automatically generated labels on the KITTI dataset [10] and KITTI-CARLA dataset [7]. The experimental results demonstrate that our proposed ADL method not only achieves impressive performance compared to manual labeling but also exhibits more robust and accurate results than both traditional methods and state-of-the-art self-supervised methods.
更多查看译文
AI 理解论文
溯源树
样例
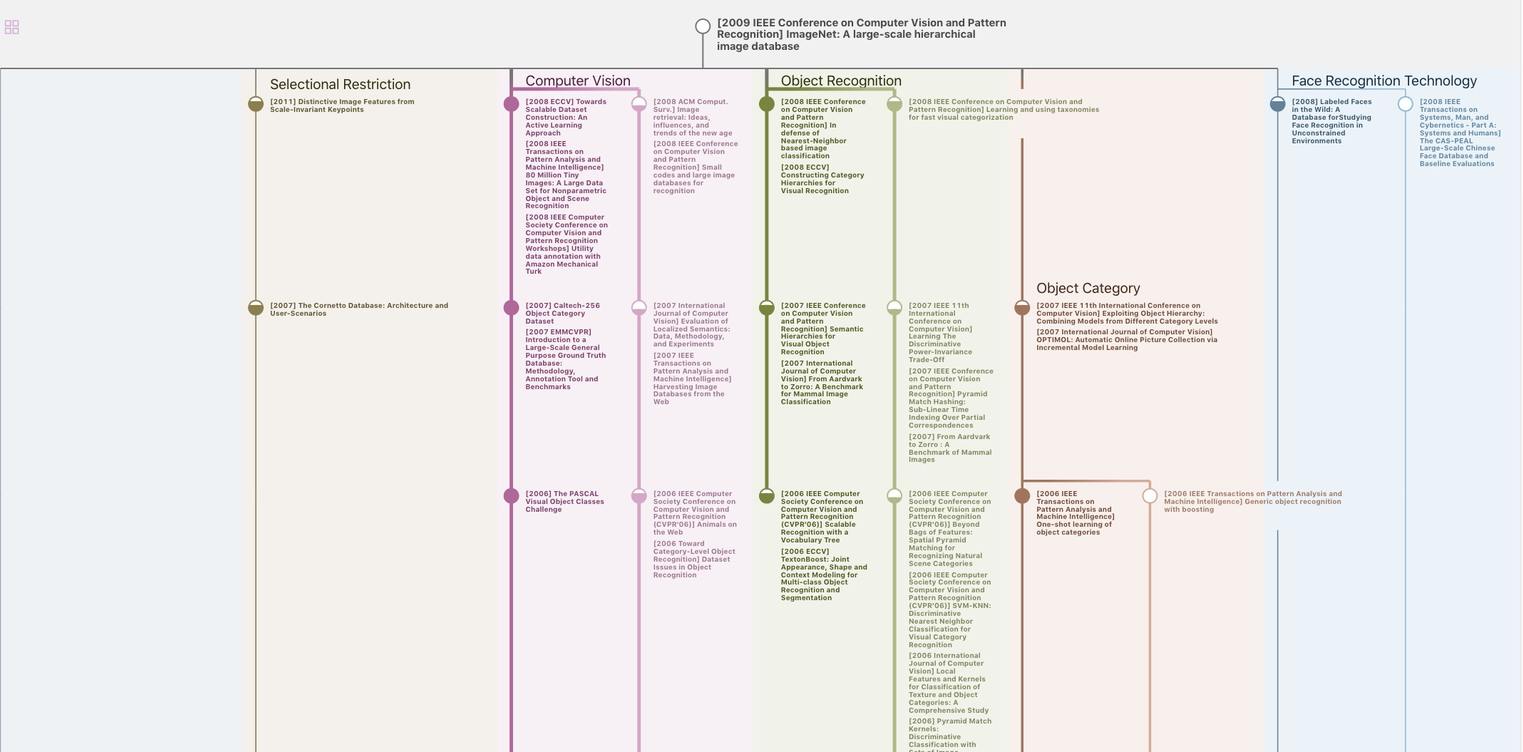
生成溯源树,研究论文发展脉络
Chat Paper
正在生成论文摘要