Similar question retrieval with incorporation of multi-dimensional quality analysis for community question answering
Neural Computing and Applications(2024)
摘要
The semantic-based method for question retrieval is an important method for searching similar questions in community question answering (CQA). The major challenges in question retrieval lie in polysemy and lexical gaps between questions, and the quality of retrieved similar questions by semantic retrieval model might not be high enough to effectively solve one’s doubts. In order to address these challenges, a high-quality and multi-level semantic analysis-based similar question retrieval framework named HQML-QR is proposed, which consists of semantic representation from tag-level and sentence-level semantics for question retrieval (TS-QR) and multi-dimensional quality analysis (MDQQ). Firstly, TS-QR extracts multi-level semantic features of the question contents, where graph embedding model is utilized to learn coarse-grained semantics of questions from the scope of the tag. Meanwhile, in order to effectively identify polysemy and extract fine-grained sentence semantic of questions, TS-QR integrates the pre-trained language model based on self-attention mechanism to ensure the accuracy of question retrieval. Secondly, based on the quality factors in CQA (i.e., popularity, question, answer and user), MDQQ constructs a multi-dimensional quality evaluation model to provide a reasonable quality measurement standard for questions. Under the guidance of the quality of questions, the similarity score obtained by semantic vector matching is updated to retrieve high-quality and semantically similar questions. Finally, experiments are executed on CQADupStack dataset from Stack Overflow and the experimental results show that the P @ N of HQML-QR has an average increase of 5.65%, 4.44% and 4.34% compared with LDA-VSM-SEM, WET-QR, RCM-QR, respectively.
更多查看译文
关键词
Community question answering,Question retrieval,Question quality,Questions tag representation
AI 理解论文
溯源树
样例
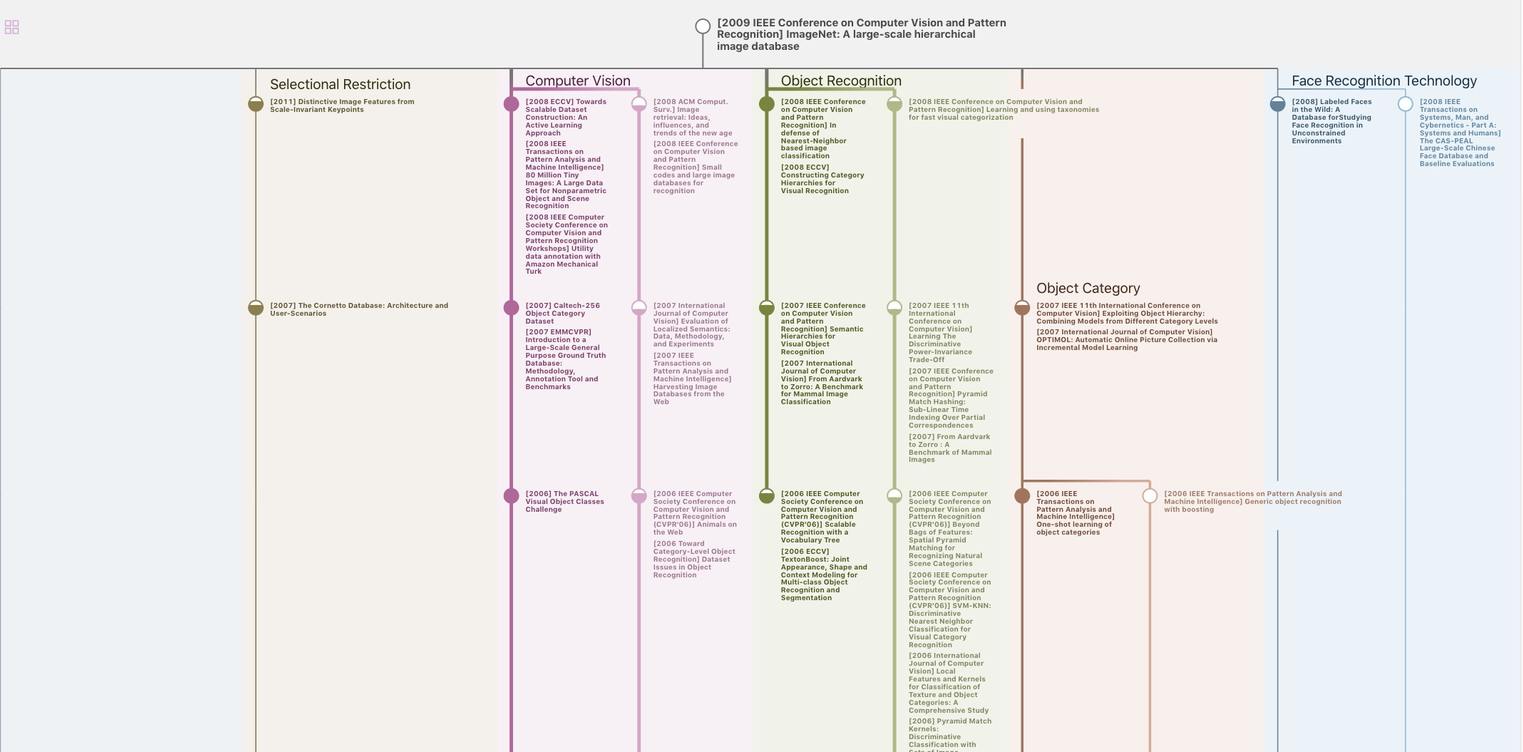
生成溯源树,研究论文发展脉络
Chat Paper
正在生成论文摘要