Multi-objective quasi-reflection learning and weight strategy-based moth flame optimization algorithm
Neural Computing and Applications(2024)
摘要
For the simultaneous optimization of many conflicting objectives, the capability of optimization algorithms must be increased. In this research, a non-dominated sorting (NDS) and crowding distance (CD)-based multi-objective variant of an advanced moth flame optimization (MFO) has been offered. Firstly, a mathematical quasi-reflection-based learning (QRL) strategy and weight strategy (WS) have been added to the classical MFO to alleviate the drawbacks of MFO. Next, this advanced MFO has been extended into multi-objective variant, namely MOQRMFO, with the help of NDS and CD approaches for well-distributed Pareto optimal front. The efficiency of the suggested MOQRMFO algorithm is tested in three phases. In the first phase, five ZDT multi-objective optimization problems (MOOPs) were considered under four performance metrics, namely general distance (GD), inverted general distance (IGD), spacing (S) and spread metric ( Δ ) and then compared it with competitive multi-objective optimization algorithms. Secondly, six problems from the DTLZ test suite are considered under the above performance metrics to examine the effectiveness of the suggested MOQRMFO algorithm. The MOQRMFO algorithm’s capacity to solve real-world problems has been evaluated by considering four multi-objective real-world engineering design issues in the third phase using several performance metrics. The experimental outcomes show that the MOQRMFO is a better candidate algorithm achieving more than 95
更多查看译文
关键词
Moth flame optimization,Quasi-reflection strategy,Multi-objective algorithm,Non-dominated sorting,Crowding distance
AI 理解论文
溯源树
样例
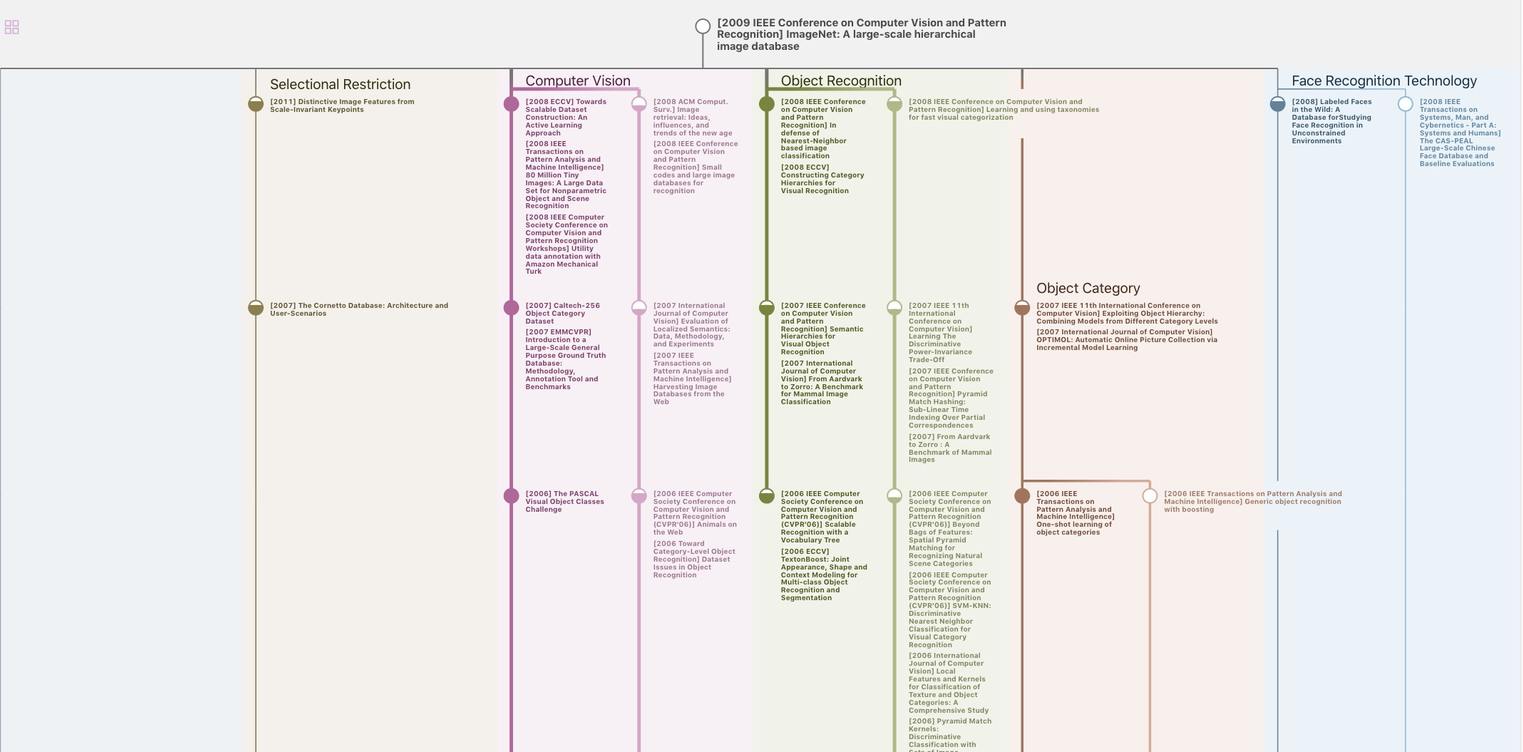
生成溯源树,研究论文发展脉络
Chat Paper
正在生成论文摘要