Joint Sparse Least Squares Via Generalized Fused Lasso Penalty for Identifying Nonlinear Dynamical Systems
NONLINEAR DYNAMICS(2024)
摘要
This paper proposes a joint sparse least-square model that utilizes a generalized fused lasso penalty to jointly identify governing equations of nonlinear dynamical systems from multiple noisy state measurements. The idea to combine a data fusion approach with least squares is that the fusion term added could make use of the more similarity information carried by different state measurements, which can further improve identified accuracy of dynamical system in regression tasks. The threshold joint sparse least-square algorithm is developed, wherein the threshold parameter is picked using the L-curve criterion. The results from the simulation experiment demonstrate our method possesses a higher accuracy in identifying dynamical system compared to traditional sparse least squares, indicating its potential power to understand gradually more complex systems from multiple data sources in the future.
更多查看译文
关键词
Nonlinear system identification,Sparse regression,Generalized fused lasso penalty,Threshold joint sparse least-square algorithm
AI 理解论文
溯源树
样例
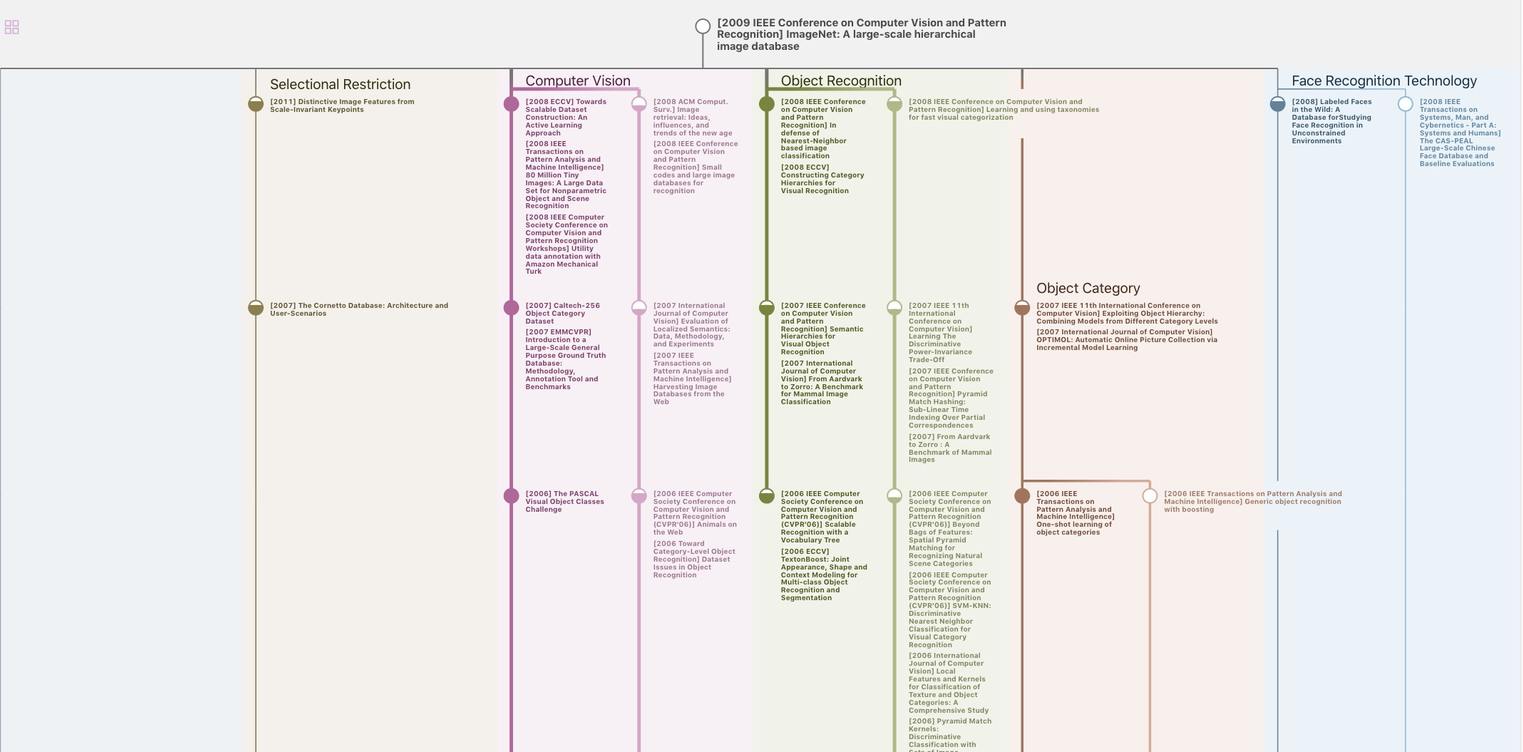
生成溯源树,研究论文发展脉络
Chat Paper
正在生成论文摘要